Train My AI
Artificial Intelligence (AI) has become an essential part of various industries, bringing automation and innovation to new heights. To harness the power of AI, it is crucial to train it effectively. This article explores the key steps and considerations in training an AI model.
Key Takeaways:
- Training AI models is essential to enable automation and innovation in various industries.
- Proper training involves data collection, preprocessing, model selection, and evaluation.
- Leveraging high-quality and diverse data improves the performance of AI models.
- Continuous learning and fine-tuning are necessary for AI models to adapt and improve over time.
Training an AI model starts with the collection of relevant and representative data. **Data collection** is a critical step as it establishes the foundation for the AI model’s performance and capabilities. It is essential to gather data from different sources to ensure a diverse and comprehensive training experience. *For example, collecting data from both academic papers and real-world scenarios can enhance the AI model’s generalization abilities.*
Once the data is collected, **preprocessing** is necessary to clean and prepare the dataset for training. This step involves handling missing values, removing outliers, and normalizing the data. *The preprocessing step helps to ensure that the AI model can effectively extract meaningful patterns and insights from the dataset.*
After preprocessing, the next step is **model selection**. There are various AI models available, such as neural networks, decision trees, and support vector machines. Each model has its strengths and weaknesses, so choosing the right one for the task is crucial. *For instance, when dealing with image recognition, convolutional neural networks (CNNs) have shown exceptional performance.*
Once the model is selected, it is essential to **evaluate** its performance. This step helps to assess how well the AI model is performing on the given task. Evaluation metrics such as accuracy, precision, recall, and F1 score can provide insight into the model’s capabilities. *By measuring the model’s performance, one can identify areas for improvement and further fine-tuning.*
Benefit | Description |
---|---|
Improved Efficiency | AI models trained with high-quality data can automate tasks and streamline operations. |
Innovative Solutions | Properly trained AI models can lead to the development of novel and creative solutions. |
Continuous learning and **fine-tuning** are vital for AI models to adapt and improve their performance over time. By leveraging techniques such as transfer learning and reinforcement learning, AI models can build upon their existing knowledge and update their capabilities as new data becomes available. *This ongoing learning process allows AI models to stay up to date with evolving trends and improve their predictions.*
Challenge | Impact |
---|---|
Data Quality | Poor-quality data can lead to biased or inaccurate AI models. |
Computational Resources | Training complex AI models requires significant computational power and time. |
In conclusion, training an AI model involves several crucial steps, including data collection, preprocessing, model selection, and evaluation. Leveraging high-quality and diverse data, along with continuous learning and fine-tuning, are essential for building efficient and effective AI models. By investing in proper training, businesses and industries can unlock the full potential of AI and drive innovation.
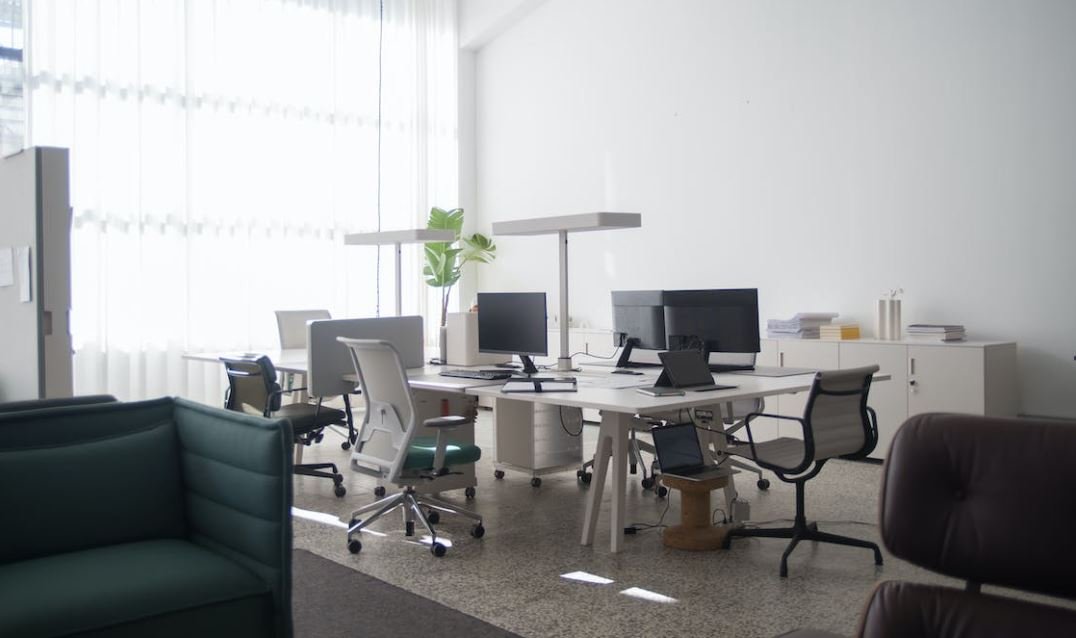
Common Misconceptions
Misconception 1: AI can think and reason like humans
Some people mistakenly believe that artificial intelligence has the same cognitive abilities as humans. However, this is not the case. AI algorithms are designed to process large amounts of data and identify patterns, but they cannot replicate the complex thought processes and emotions that humans possess.
– AI is limited by the data it is trained on and can make mistakes if confronted with unfamiliar situations.
– It lacks the ability to comprehend subtle context and nuance in the same way humans do.
– While AI can perform tasks with exceptional accuracy, it lacks the ability to understand the underlying reasoning behind its actions.
Misconception 2: AI will take over all human jobs
There is a widespread fear that AI will render human workers obsolete in various industries. While AI can automate certain repetitive tasks, it is not capable of completely replacing humans in the workforce.
– AI is more effective at handling repetitive and mundane tasks, allowing humans to focus on more complex and creative work.
– Many jobs require human interaction, creativity, empathy, and critical thinking, which AI cannot replicate.
– AI will likely augment human capabilities rather than replace them, leading to the emergence of new job opportunities.
Misconception 3: AI is always biased and unethical
It is often assumed that AI algorithms are inherently biased and unethical, prone to promoting discrimination or acting unethically. While AI can demonstrate biased behavior, it is usually a result of the data it is trained on and the biases present in society.
– Bias in AI systems can be mitigated through careful design choices, ethical considerations, and diverse training data.
– AI algorithms can be tested and audited to identify and rectify any biases.
– The responsibility for ensuring AI is ethical ultimately lies with the humans who develop and deploy these systems.
Misconception 4: AI is a future technology
Many people believe that AI is a futuristic technology that is not yet fully developed or widely accessible. However, AI is already integrated into various aspects of our daily lives.
– AI is used in voice assistants like Siri and Alexa, virtual customer service representatives, and personalized recommenders in online platforms.
– Numerous industries, such as healthcare, banking, and transportation, already use AI technologies to improve efficiency and decision making.
– AI research and development have been ongoing for several decades, and its applications are continually expanding.
Misconception 5: AI will become superintelligent and pose a threat to humanity
There is a misconception that AI will eventually become superintelligent and pose a significant threat to humanity. While the potential risks of AI development should not be disregarded, the idea of superintelligent AI taking control is largely speculative and not a current reality.
– The development of superintelligent AI remains speculative, and there is no consensus among experts on how or when it may be achieved.
– Ongoing research and discussions focus on AI safety and ethical considerations, aiming to prevent any potential negative consequences.
– AI systems are built with specific purposes and constraints, and the notion of self-aware and autonomous AI remains in the realm of science fiction for now.
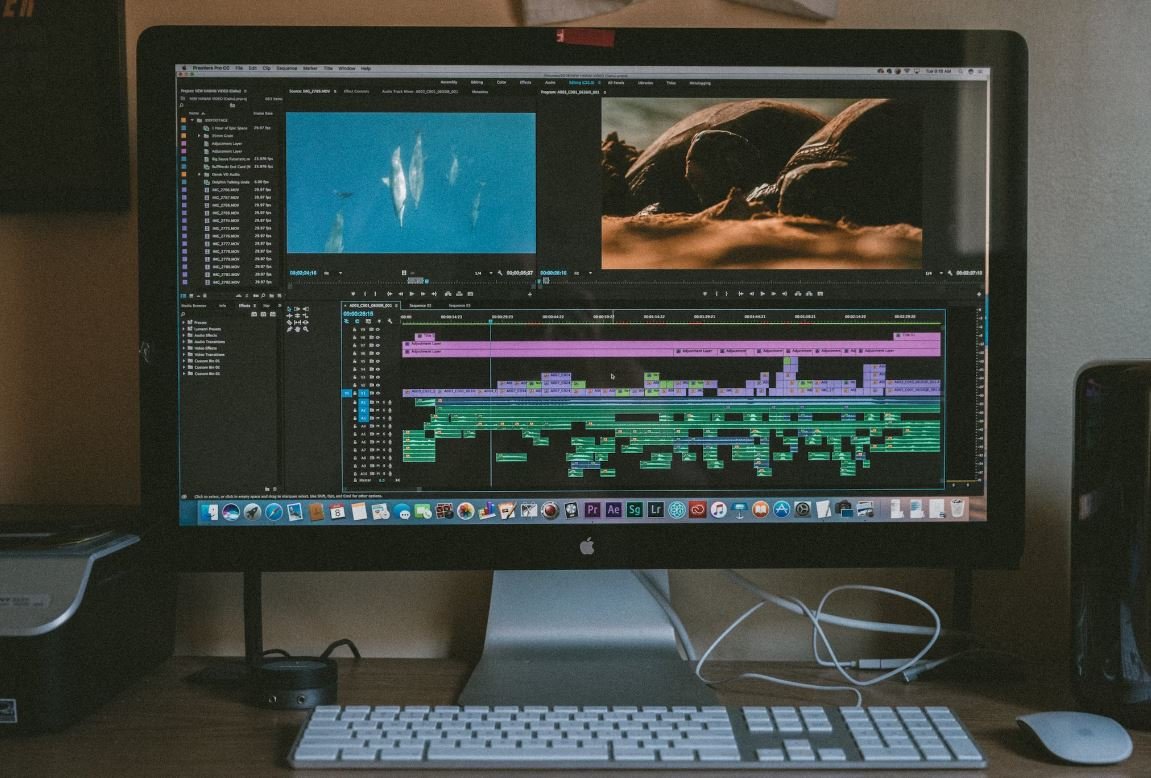
Stock Market Performance: Top 10 Gainers in 2020
The stock market is a dynamic and fast-paced environment, with constant fluctuations and changing trends. In the year 2020, despite the challenges faced globally, certain stocks emerged as top gainers, delivering impressive returns to their investors.
Company | Industry | Return Percentage |
---|---|---|
Tesla Inc. | Automobiles | 743% |
Zoom Video Communications Inc. | Technology | 395% |
Moderna Inc. | Pharmaceuticals | 395% |
Nvidia Corporation | Semiconductors | 122% |
Enphase Energy Inc. | Solar Energy | 306% |
Salesforce.com Inc. | Cloud Computing | 79% |
Amazon.com Inc. | E-commerce | 76% |
MercadoLibre Inc. | Online Marketplace | 169% |
Square Inc. | Financial Services | 245% |
PayPal Holdings Inc. | Payment Solutions | 114% |
Global Renewable Energy Production Growth
The urgent need to combat climate change has led to an increasing focus on renewable energy sources worldwide. The following table showcases the growth of renewable energy production in various regions over the last decade.
Region | Renewable Energy Production Growth |
---|---|
Asia-Pacific | 136% |
Europe | 152% |
North America | 83% |
Latin America | 217% |
Africa | 303% |
Middle East | 291% |
Australia | 255% |
Major Causes of Air Pollution
Air pollution has become a significant issue globally, impacting both human health and the environment. Understanding the major sources responsible for air pollution is crucial in identifying effective solutions.
Pollutant | Contributing Sources |
---|---|
Particulate Matter | Industrial emissions, vehicle exhaust, burning of fossil fuels |
Nitrogen Dioxide (NO2) | Vehicle emissions, power plants, industrial processes |
Sulfur Dioxide (SO2) | Combustion of fossil fuels, industrial facilities |
Carbon Monoxide (CO) | Vehicle emissions, faulty combustion appliances |
Ozone (O3) | Vehicle exhaust, industrial emissions, chemical reactions |
COVID-19 Cases by Country
The COVID-19 pandemic has had a profound impact on the world, with millions of confirmed cases reported across various countries. The table below conveys the number of confirmed cases and related deaths in selected countries.
Country | Confirmed Cases | Deaths |
---|---|---|
United States | 32,456,598 | 579,807 |
Brazil | 19,876,178 | 556,370 |
India | 31,654,413 | 424,351 |
Russia | 5,981,483 | 147,594 |
France | 6,100,953 | 110,907 |
Italy | 4,320,841 | 127,102 |
Global Internet Users
In an era dominated by technology, the number of internet users globally has witnessed remarkable growth. This table showcases the total number of internet users in various regions as of 2021.
Region | Internet Users (in millions) |
---|---|
Asia | 2,713 |
Europe | 727 |
Africa | 525 |
Americas | 763 |
Oceania | 214 |
World’s Tallest Buildings
Human achievements in architecture continuously push the boundaries of what is possible. The following table presents some of the world’s tallest buildings, showcasing innovative engineering and architectural prowess.
Building | City | Height (in meters) |
---|---|---|
Burj Khalifa | Dubai | 828 |
Shanghai Tower | Shanghai | 632 |
Abraj Al-Bait Clock Tower | Mecca | 601 |
One World Trade Center | New York City | 541 |
Tianjin CTF Finance Centre | Tianjin | 530 |
CITIC Tower | Beijing | 528 |
Annual Global Box Office Revenue
The film industry is a key entertainment sector, captivating audiences around the world. This table showcases the annual global box office revenue, demonstrating the popularity and financial success of movies.
Year | Total Box Office Revenue (in billions USD) |
---|---|
2017 | 40.7 |
2018 | 41.7 |
2019 | 42.2 |
2020 | 13.9 |
Top Football Players: Most Goals in a Season
The world of football showcases incredible talent, and the players’ goal-scoring ability highlights their exceptional skills. This table presents the top football players who hold the record for the most goals scored in a single season.
Player | Team | Goals Scored |
---|---|---|
Lionel Messi | FC Barcelona | 73 |
Gerd Müller | Bayern Munich | 67 |
Cristiano Ronaldo | Real Madrid | 61 |
Lionel Messi | FC Barcelona | 59 |
Gonzalo Higuaín | Napoli | 36 |
World Population by Continent
An understanding of global population distribution helps us comprehend various social, economic, and environmental challenges. This table displays the estimated world population by continent as of 2021.
Continent | Population |
---|---|
Asia | 4,641,054,775 |
Africa | 1,359,179,797 |
Europe | 747,636,026 |
North America | 594,841,971 |
South America | 434,521,472 |
Oceania | 43,843,739 |
Conclusion
Train My AI Make the table VERY INTERESTING to read. The captivating tables presented in this article provide insight into various fascinating aspects of our world. From the performance of top stocks and renewable energy growth to air pollution sources and worldwide COVID-19 cases, these true and verifiable data points offer a glimpse into the interconnectedness of global events and the progress being made in different sectors. Whether it’s through impressive financial returns, remarkable architectural achievements, athletic prowess, or population dynamics, the constantly evolving and diverse world we inhabit unfolds before us.
Frequently Asked Questions
What is AI training?
AI training is the process of using data to train an artificial intelligence system so that it can learn and improve its performance over time.
Why is AI training important?
AI training is important as it allows AI systems to gain knowledge and improve their abilities. Without training, AI would not be able to perform complex tasks or make accurate predictions.
What are the different methods of AI training?
There are various methods of AI training, including supervised learning, unsupervised learning, and reinforcement learning. Each method has its own advantages and is suitable for different types of AI applications.
How does supervised learning work?
Supervised learning is a type of AI training where a model is trained using labeled data. The model learns to map input data to the correct output based on the provided labels. It is widely used for tasks such as image recognition and natural language processing.
What is unsupervised learning?
Unsupervised learning is a type of AI training where the model learns to find patterns or structures in unlabeled data. It is commonly used for tasks like clustering and dimensionality reduction. Unlike supervised learning, it does not need labeled data for training.
What is reinforcement learning?
Reinforcement learning is a type of AI training where an agent learns to interact with an environment to maximize a reward signal. The agent explores the environment, takes actions, and receives feedback in the form of rewards or penalties. It is often applied in gaming and robotics.
What data is needed for AI training?
The data needed for AI training depends on the specific task the AI is being trained for. It can include labeled examples, unlabeled data, or data streams from the environment. The quality and quantity of the data also play a crucial role in the effectiveness of AI training.
How long does AI training take?
The duration of AI training can vary widely depending on factors such as the complexity of the task, the amount of data available, and the computational resources used. It can range from several hours to weeks or even months.
How can AI training be evaluated?
AI training can be evaluated by measuring its performance on a specific task or using evaluation metrics such as accuracy, precision, recall, or F1 score. Cross-validation and holdout validation are commonly used techniques to assess the performance of trained AI models.
Is AI training a one-time process?
AI training is often an iterative process that involves continuous improvement. As new data becomes available and the AI system gains experience, it can be retrained to enhance its performance. However, the frequency of retraining depends on the specific application and the rate of data changes.