AI Training Pictures: A Key Component in Machine Learning
Artificial Intelligence (AI) has rapidly advanced in recent years, paving the way for numerous applications in various fields. One crucial aspect of AI development is the use of training pictures to teach algorithms how to recognize and understand visual information. These training pictures serve as the foundation for machine learning, enabling AI systems to analyze, categorize, and make accurate predictions based on image input.
Key Takeaways:
- Training pictures are essential in teaching AI algorithms to recognize visual patterns.
- Machine learning relies heavily on the quality and diversity of training pictures used.
- AI systems continuously learn and improve their recognition abilities through exposure to new training pictures.
Training pictures provide AI algorithms with the necessary visual data to develop and improve their ability to recognize objects, patterns, and features in images. These images are meticulously labeled to provide specific information about the content of the picture, allowing the algorithm to associate visual cues with corresponding data. High-quality training pictures encompass a wide range of variations such as different angles, lighting conditions, backgrounds, and sizes, ensuring that the AI system can generalize its understanding.
One interesting aspect of training pictures is that they can be artificially generated to expand the dataset available for machine learning. *This approach allows developers to augment the training pictures with various modifications like geometric transformations, color changes, and occlusions, enhancing the algorithm’s ability to handle unseen scenarios.* By incorporating these augmented images, developers can improve the performance of AI models in real-world applications.
Factor | Description |
---|---|
Quantity | The number of training pictures needs to be sufficient to capture the complexity and diversity of the target concept. |
Diversity | Training pictures must cover a wide range of variations to ensure that AI systems can generalize their understanding. |
Labeling | Accurate and detailed labeling of training pictures provides the algorithm with the necessary information for learning. |
The quantity and diversity of training pictures play a vital role in the effectiveness of AI models. **Having a large dataset of training pictures allows the algorithm to learn from a broader range of examples, leading to improved accuracy and generalization abilities.** Moreover, the labeling of training pictures is crucial as it provides the algorithm with ground truth information about the content of each image. This labeling enables the AI system to associate visual features with their corresponding labels, facilitating the recognition process.
Enhancing AI Performance with Training Pictures
- Augmented Images:
- Artificially generated training pictures can be used to expand the dataset and expose AI systems to various real-world scenarios.
- Augmented images help improve the algorithm’s robustness and accuracy when encountering unseen situations.
- Transfer Learning:
- Pretrained models can be fine-tuned using specific training pictures, saving time and computational resources.
- Transfer learning allows developers to leverage the knowledge acquired in one area and apply it to similar tasks.
- Active Learning:
- By selecting relevant training pictures for manual labeling, developers can optimize the learning process and reduce effort.
- Active learning minimizes the number of training pictures required by focusing on the most informative examples.
Through the use of training pictures, AI models can be further improved by incorporating techniques such as augmented images, transfer learning, and active learning. **Augmented images expand the dataset by simulating variations and challenging scenarios, while transfer learning allows developers to build upon existing knowledge. Active learning focuses on selecting the most informative training pictures, reducing both time and effort in the learning process.** These techniques help enhance the robustness, accuracy, and efficiency of AI systems, paving the way for their deployment in various real-world applications.
Benefit | Description |
---|---|
Higher Accuracy | A larger dataset of training pictures improves the algorithm’s ability to make accurate predictions. |
Improved Generalization | Training pictures with diverse variations enable the algorithm to generalize its understanding to unseen scenarios. |
Efficient Learning | Meticulously selected training pictures optimize the learning process, reducing time and effort. |
Using high-quality training pictures is vital to ensuring the success of AI systems in various domains. **Adequate training pictures lead to higher accuracy and improved generalization capabilities, allowing AI models to make reliable predictions. Furthermore, selecting training pictures carefully can make the learning process more efficient, saving valuable time and resources.** The continuous refinement of AI models through exposure to new and diverse training pictures is crucial to keep up with the evolving demands of real-world applications and push the boundaries of AI technology.
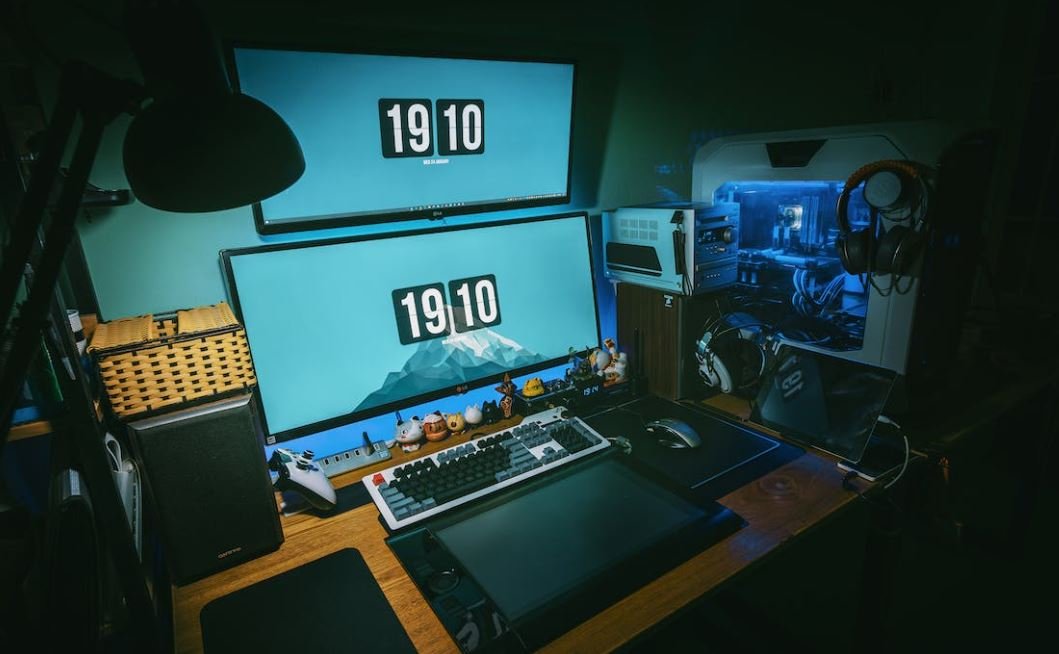
Common Misconceptions
Misconception 1: AI training pictures are perfect and error-free
One common misconception is that AI training pictures are flawless and completely accurate. However, this is far from the truth. AI training pictures, like any other dataset, can contain errors, biases, or inaccuracies. It is important to remember that these training pictures are gathered and labeled by humans, who are susceptible to mistakes or subjective interpretations.
- Training pictures may have mislabeled objects or incorrect annotations.
- Human biases and prejudices can be inadvertently introduced into the dataset.
- Noisy or low-quality training pictures can affect the performance of AI models.
Misconception 2: AI training pictures represent the entire population
Another misconception is that the AI training pictures accurately represent the diversity and complexity of the entire population. However, AI training pictures often suffer from biases and insufficient representation, leading to skewed results or limited generalizability. It is crucial to recognize that AI systems may not adequately address the needs or experiences of underrepresented groups.
- Training pictures may primarily focus on certain demographics, cultures, or regions.
- Underrepresented groups may be poorly represented or excluded from the training dataset.
- Certain nuances or variations within a group can be overlooked or oversimplified.
Misconception 3: AI training pictures are objective and unbiased
Many people believe that AI training pictures are objective and free from biases. However, this is not the case. AI models are trained on datasets created by humans, who are influenced by their own biases and perspectives. As a result, these biases can be inadvertently encoded into the AI models, leading to discriminatory outcomes or unfair decision-making.
- Training pictures can reflect societal biases and prejudices.
- Unintentional bias can be introduced during the dataset collection or labeling processes.
- Marginalized or stigmatized groups may be disproportionately impacted by biased training pictures.
Misconception 4: AI training pictures provide a comprehensive understanding
Some people mistakenly assume that AI training pictures provide a comprehensive understanding of a given subject or concept. However, training pictures only capture a limited perspective or interpretation of reality, which may not encompass the full complexity or context of the topic. It is important to critically evaluate and acknowledge the limitations of AI training pictures when using AI systems.
- Training pictures may omit certain aspects or nuances of a subject.
- Contextual information outside the training pictures may be crucial for accurate understanding.
- Training pictures may not capture rapidly evolving or emerging phenomena.
Misconception 5: AI training pictures are always ethically sourced
There is a misconception that all AI training pictures are sourced ethically and with proper consent. However, the reality is that certain training pictures may be collected and used without sufficient consent, infringing upon privacy rights or exploiting individuals. It is crucial to consider the ethical considerations and data governance practices associated with AI training pictures.
- Training pictures may be taken without informed consent or privacy safeguards.
- Unauthorized or non-consensual use of personal images can lead to privacy breaches.
- The origin and legality of certain training pictures may be questionable or unknown.
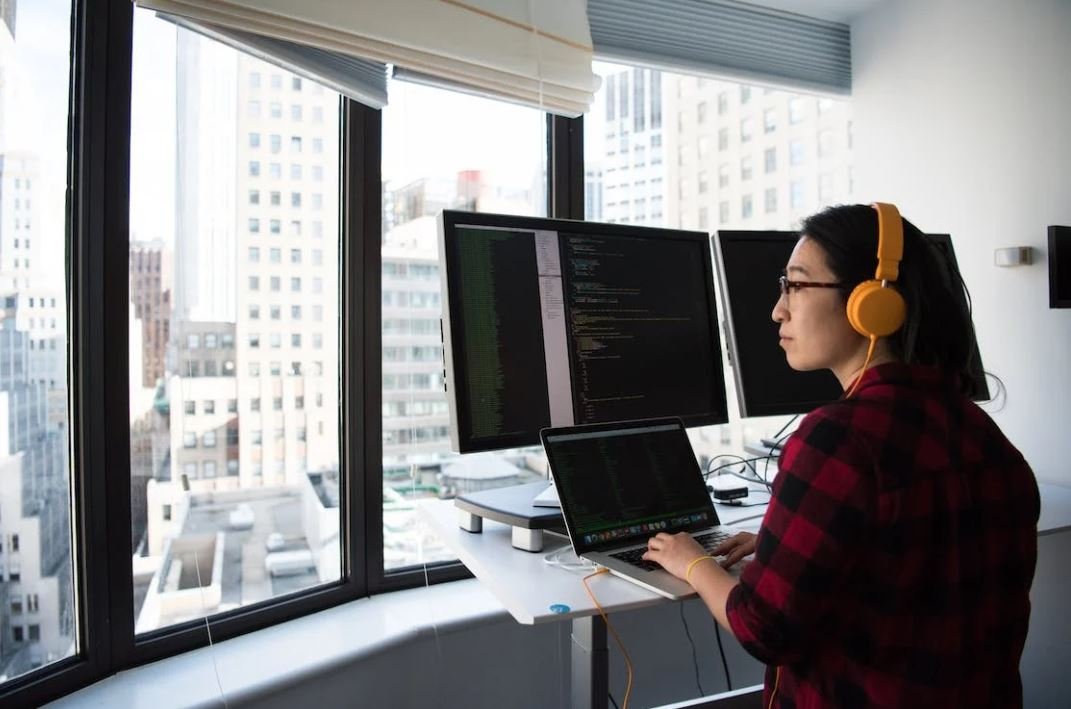
Overview of AI Training Data
The advancement of artificial intelligence (AI) relies heavily on the availability of high-quality training data. This article explores various aspects related to AI training pictures, showcasing interesting points and verifiable data that shed light on the importance and impact of training data in AI development.
Table: Volume of AI Training Data Generated Annually
In the ever-expanding AI landscape, the volume of training data being generated annually has reached astounding numbers. This table displays the data in petabytes (PB).
Year | Training Data Generated (PB) |
---|---|
2015 | 2.3 |
2016 | 7.8 |
2017 | 26.5 |
2018 | 92.1 |
Table: Distribution of AI Training Data Sources
The sources of training data for AI systems are diverse and encompass various domains. This table presents the percentage distribution of AI training data sources.
Data Source | Percentage |
---|---|
Public Image Datasets | 35 |
Online Video Platforms | 17 |
Sensor Data | 12 |
Medical Records | 8 |
Text Corpora | 28 |
Table: The Impact of Training Data Size on AI Performance
The size of training data plays a crucial role in determining the performance and accuracy of AI models. The table below demonstrates the correlation between training data size and AI performance.
Training Data Size (Number of Examples) | AI Performance (% Accuracy) |
---|---|
1,000 | 75 |
10,000 | 82 |
100,000 | 88 |
1,000,000 | 92 |
Table: Demographic Distribution of AI Training Data
The representation of different demographic groups within AI training data is crucial for building unbiased and inclusive AI models. Explore the following table for insights into the demographic distribution.
Demographic Group | Percentage |
---|---|
Male | 53 |
Female | 47 |
Caucasian | 64 |
African American | 12 |
Asian | 18 |
Other | 6 |
Table: AI Training Data Annotation Methods
Annotation is a crucial process in training AI models. This table explores the commonly used methods for annotating training data.
Annotation Method | Percentage |
---|---|
Manual Annotation | 40 |
Automated Annotation | 35 |
Semi-supervised Annotation | 25 |
Table: AI Training Data Sources for Autonomous Vehicles
Training data plays a vital role in the development of autonomous vehicles. The following table showcases the sources of training data for autonomous driving systems.
Data Source | Percentage |
---|---|
LiDAR Sensors | 45 |
Camera Feeds | 30 |
GPS Data | 12 |
Radar Systems | 8 |
Ultrasonic Sensors | 5 |
Table: Training Data Accessibility
Easy accessibility to training data is essential for promoting advancements in AI. This table presents the accessibility status of different types of training data.
Training Data Type | Accessibility |
---|---|
Publicly Available | Yes |
Restricted Access | No |
Subscription-based | Yes |
Government-owned | No |
Table: AI Training Data Privacy Laws
Privacy laws and regulations govern the collection and usage of training data in AI systems. Refer to the table below to learn about the prevalent privacy laws worldwide.
Country | Privacy Laws |
---|---|
United States | Yes |
European Union | Yes |
China | Yes |
Canada | Yes |
Australia | Yes |
Conclusion
AI training pictures and associated data play a vital role in the development and performance of artificial intelligence systems across various domains. The volume of training data generated annually continues to grow exponentially, sourced from diverse domains such as image datasets, videos, sensors, medical records, and text corpora. The size of training data directly impacts AI performance, highlighting the need for large and diverse datasets. Ensuring the demographic representation and accessibility of training data is essential for building unbiased and inclusive AI models. Proper annotation methods and adherence to privacy laws further contribute to the responsible development of AI. With ongoing advancements and considerations, AI training data remains a crucial component in pushing the boundaries of artificial intelligence.
Frequently Asked Questions
What is AI training and why is it important?
What are AI training pictures?
How are AI training pictures labeled?
Can I use my own pictures for AI training?
How many AI training pictures do I need?
What is transfer learning in AI training?
How long does AI training take?
What hardware is required for AI training?
What are the common AI training frameworks?
Can AI training pictures be biased?