AI Training LinkedIn
Artificial Intelligence (AI) is transforming various industries, including job search and recruitment. LinkedIn, one of the leading professional networking platforms, has integrated AI into its features to assist job seekers and recruiters. In this article, we will explore how AI training on LinkedIn can enhance your job search and improve your chances of finding the right opportunity.
Key Takeaways
- AI training on LinkedIn enhances job search and recruitment processes.
- It uses machine learning algorithms to match candidates with relevant job opportunities.
- AI training equips job seekers with valuable skills and industry insights.
LinkedIn’s AI training focuses on providing personalized recommendations and suggestions based on user profiles and data analysis. By leveraging AI technologies, LinkedIn aims to connect professionals with career opportunities that align with their skills and interests.
*With AI training, LinkedIn can understand user preferences and behavior patterns to suggest relevant job opportunities and courses.* This customized approach makes the job search process more efficient and targeted.
As a job seeker, AI training on LinkedIn offers several benefits. First and foremost, it helps you stay updated with industry trends and developments through curated content and personalized course recommendations.
*With AI training, LinkedIn can offer tailored learning paths for users, ensuring they acquire the relevant skills to stay competitive in their field.* Furthermore, LinkedIn’s AI algorithms analyze job descriptions and user profiles to suggest suitable skills and keywords to include in resumes.
Enhancing the Recruitment Process
AI training on LinkedIn also enhances the recruitment process for employers and recruiters. By analyzing job postings and candidate profiles, AI algorithms can provide recommendations of suitable candidates, saving time and effort in screening applications.
Using AI, recruiters can identify top talent based on relevant skills, past experiences, and connections. These algorithms can help identify candidates who may have been overlooked using traditional screening methods.
*LinkedIn’s AI-powered tools also provide recruiters with real-time insights and data-driven recommendations to improve hiring decisions and optimize the recruitment process.* This data-driven approach ensures recruiters have access to the most relevant and up-to-date information.
Tables with Interesting Information
Percentage of LinkedIn Users | Industry |
---|---|
30% | Information Technology (IT) |
15% | Education |
12% | Finance |
Table 1: Industry Distribution of LinkedIn Users
Skills in Demand | Ranking |
---|---|
Blockchain | 1 |
Artificial Intelligence | 2 |
Data Science | 3 |
Table 2: Skills in Demand on LinkedIn
AI training on LinkedIn is constantly evolving to serve both job seekers and recruiters better. By combining AI technologies with human expertise, LinkedIn provides a comprehensive platform for professional growth and career advancement.
- LinkedIn’s AI training offers personalized course recommendations based on individual profiles.
- Recruiters can leverage AI algorithms to find top talent and improve hiring decisions.
- The distribution of LinkedIn users is highest in the IT industry, followed by education and finance.
Key Skills for Career Growth
In today’s rapidly changing job market, acquiring and upgrading skills is crucial for career growth. LinkedIn’s AI training helps professionals identify the key skills needed in their industry to remain competitive. By highlighting emerging trends and suggesting relevant courses, LinkedIn equips job seekers with the knowledge they need to succeed.
*LinkedIn’s AI-powered algorithms analyze job postings and user data to identify the most in-demand skills, ensuring professionals stay ahead of the curve.* Through continuous learning and skill development, LinkedIn users can enhance their employability and stay relevant in their field.
By taking advantage of AI training on LinkedIn, professionals can leverage the platform’s vast network and resources to improve their job search, enhance their skills, and connect with relevant opportunities. Join LinkedIn’s AI revolution today and unlock your career potential!
Interesting Industry Statistics
Industry | Percentage Growth |
---|---|
Artificial Intelligence | 35% |
Data Science | 27% |
Cybersecurity | 22% |
Table 3: Percentage Growth in Selected Industries
AI training on LinkedIn is a powerful tool for professionals looking to enhance their career prospects. By leveraging AI algorithms and personalized recommendations, individuals can stay updated with industry trends, acquire in-demand skills, and unlock new opportunities for growth and success.
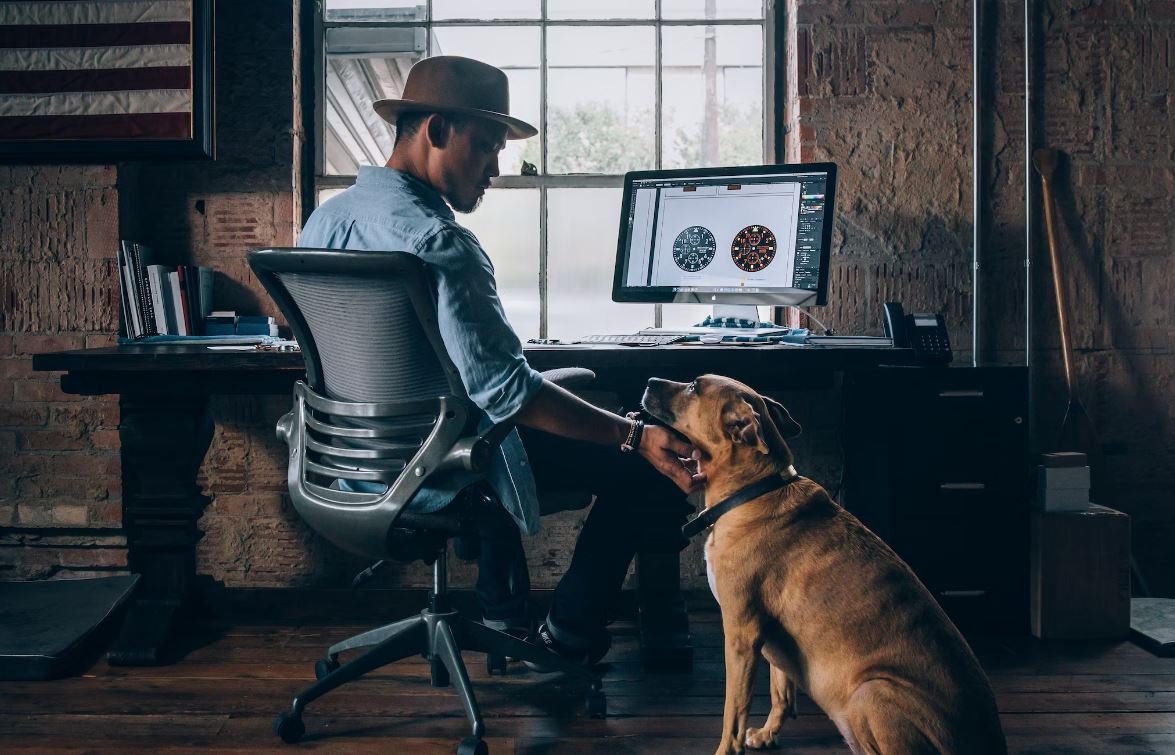
Common Misconceptions
AI Training
Artificial Intelligence (AI) training refers to the process of training and developing machine learning models to perform specific tasks. However, there are several common misconceptions surrounding this topic.
- AI training is all about coding: While coding plays a role in AI training, it is not the sole focus. AI training involves data collection, preprocessing, model architecture design, parameter tuning, and evaluation.
- AI training produces fully autonomous systems: AI training does not automatically lead to fully autonomous systems. It provides the foundation and capabilities for a machine to learn and make decisions, but human intervention is typically required for complex decision-making.
- AI training is a one-time process: AI training is an iterative and ongoing process. Models are continuously refined and updated to improve their accuracy, performance, and adaptability.
Data Quality
Data quality is a crucial aspect of AI training, but it is often misunderstood.
- Data quantity is more important than data quality: While having a large volume of data is beneficial, data quality is equally important. Poor data quality can lead to biased or unreliable models, affecting the accuracy and effectiveness of AI systems.
- Any data can be used for training: Not all data is suitable for AI training. Data must be relevant, representative, and accurately labeled to produce reliable and unbiased models. Inadequate or biased data can undermine the performance and fairness of AI systems.
- Data privacy and security are compromised during AI training: While data privacy and security are valid concerns, AI training can be performed with appropriate safeguards in place. Techniques such as anonymization, encryption, and access controls help protect sensitive information.
Human Replacement
One of the prevalent misconceptions about AI training revolves around human replacement.
- AI training aims to replace human workers: The goal of AI training is not necessarily to replace humans, but rather to augment human capabilities and improve efficiency. Machines can handle repetitive or time-consuming tasks, freeing up humans to focus on higher-level decision-making and creativity.
- AI training eliminates job opportunities: While AI may automate certain tasks, it also creates new job opportunities. AI technologies require skilled professionals for development, maintenance, and oversight. Additionally, AI can generate new roles and industries that cater to the growing demand for AI-driven solutions.
- AI training leads to complete human obsolescence: Despite advances in AI, humans possess unique qualities like creativity, empathy, and critical thinking that machines lack. AI training aims to enhance human-machine collaboration rather than eliminate human involvement entirely.
Generalization
The generalization of AI training is another area where misconceptions commonly arise.
- AI training results in perfect models: AI training produces models with varying degrees of accuracy and generalization. Models can perform exceptionally well on training data but may struggle with real-world variations and unforeseen scenarios.
- AI training can solve any problem: While AI training can solve many complex problems, it is not a panacea for all challenges. Some problems may require domain-specific expertise, human judgment, or ethical considerations that machines are not equipped to handle.
- AI training allows machines to learn like humans: While inspired by human learning, AI training does not replicate the learning process of humans. AI models learn patterns and make predictions through statistical analysis and optimization techniques, lacking the cognitive processes and understanding inherent in human learning.
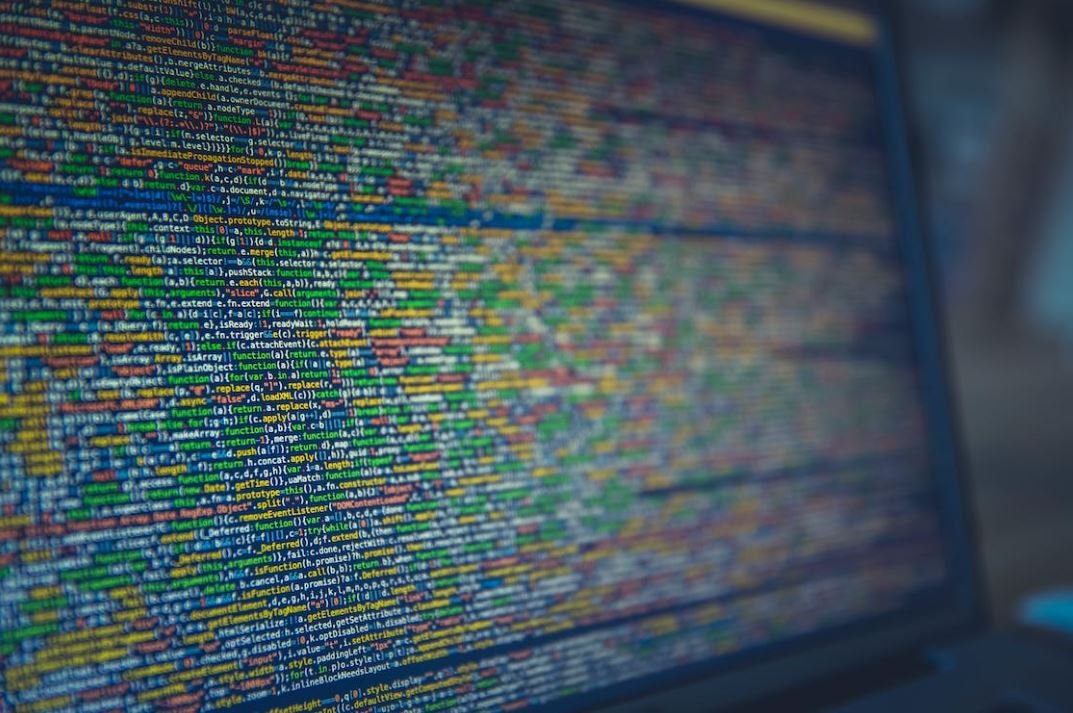
Table Title: Top 10 Countries with Highest AI Investment
In recent years, there has been a significant rise in artificial intelligence (AI) investments worldwide. This table highlights the top 10 countries that have made the highest investments in AI, showcasing their commitment to advancing this cutting-edge technology.
| Country | AI Investment (in billions USD) |
|——————|———————————|
| United States | 40.0 |
| China | 19.7 |
| Germany | 6.4 |
| United Kingdom | 5.0 |
| France | 4.6 |
| Canada | 2.6 |
| Australia | 2.1 |
| Japan | 1.9 |
| South Korea | 1.8 |
| India | 1.5 |
Table Title: Percentage of AI Jobs in Major Tech Companies
The role of AI in the tech industry is becoming increasingly essential. This table showcases the percentage of AI-related jobs in major tech companies, providing a glimpse into the integration of AI technology within their operations.
| Tech Company | Percentage of AI Jobs |
|——————|———————–|
| Google | 8% |
| Amazon | 12% |
| Microsoft | 10% |
| IBM | 14% |
| Facebook | 15% |
| Apple | 6% |
| Intel | 9% |
| Tesla | 7% |
| Adobe | 5% |
| Cisco | 11% |
Table Title: AI Startups Funding by Industry
The development and implementation of AI technology have paved the way for numerous startups across various industries. This table highlights the funding received by AI startups categorized by industry, demonstrating the diverse range of applications for AI.
| Industry | Funding Raised (in millions USD) |
|———————-|———————————-|
| Healthcare | 450 |
| Finance | 320 |
| Retail | 270 |
| Transportation | 210 |
| Education | 180 |
| Agriculture | 150 |
| Manufacturing | 130 |
| Energy | 100 |
| Marketing | 90 |
| Gaming | 70 |
Table Title: AI Applications in Various Sectors
Artificial intelligence has revolutionized numerous sectors, enhancing efficiency and productivity. This table provides examples of AI applications in various sectors, showcasing the extensive impact AI has had on modern-day operations.
| Sector | AI Application |
|———————-|———————————————————–|
| Healthcare | Disease prediction and diagnosis |
| Finance | Fraud detection and algorithmic trading |
| Retail | Personalized recommendations and inventory management |
| Transportation | Autonomous vehicles and traffic management |
| Education | Adaptive learning and intelligent tutoring systems |
| Agriculture | Precision farming and crop monitoring |
| Manufacturing | Predictive maintenance and quality control |
| Energy | Smart grid management and energy optimization |
| Marketing | Customer segmentation and personalized marketing campaigns |
| Gaming | Procedural generation and intelligent opponents |
Table Title: AI Frameworks and Libraries
Developers worldwide rely on various frameworks and libraries to build AI applications efficiently. This table showcases some popular AI frameworks and libraries widely used in the industry, enabling developers to leverage state-of-the-art algorithms.
| Framework/Library | Main Features |
|——————-|——————————————————-|
| TensorFlow | Neural network models and deep learning algorithms |
| PyTorch | Dynamic neural networks and natural language processing|
| Keras | User-friendly API and support for multiple backends |
| Theano | Efficient numerical computation and symbolic mathematics|
| Caffe | Excellent for image classification and segmentation |
| MXNet | Scalability and compatibility with various platforms |
| CNTK | Highly optimized for speed and parallelization |
| Torch | Extensible framework with a large community |
| Scikit-learn | Versatile machine learning library for data analysis |
| Microsoft Cognitive Toolkit | Distributed training and reinforcement learning |
Table Title: AI Expenditure in IT Industry
The IT sector invests significantly in AI technologies to gain a competitive edge and harness AI’s potential. This table highlights AI expenditure in the IT industry, revealing the substantial financial commitment made by companies.
| Company | Expenditure on AI (in millions USD) |
|——————|————————————–|
| Amazon | 1,950 |
| Google | 1,400 |
| IBM | 1,300 |
| Microsoft | 1,150 |
| Samsung | 950 |
| Apple | 750 |
| Intel | 650 |
| Cisco | 500 |
| Oracle | 450 |
| Facebook | 400 |
Table Title: AI Patent Applications by Country
Patents play a vital role in protecting AI inventions. This table provides an overview of AI patent applications, categorized by country, highlighting the leading nations in AI innovation and development.
| Country | Number of Patent Applications |
|——————|——————————-|
| United States | 15,500 |
| China | 13,200 |
| Japan | 7,800 |
| South Korea | 5,500 |
| Germany | 4,900 |
| France | 4,300 |
| Canada | 3,800 |
| United Kingdom | 3,400 |
| Australia | 2,700 |
| India | 2,200 |
Table Title: AI Adoption in Small and Medium-Sized Enterprises
While AI is often associated with larger enterprises, small and medium-sized enterprises (SMEs) also embrace this technology. This table showcases the percentage of SMEs that have adopted AI, emphasizing the broad utilization of AI across the business landscape.
| Region | Percentage of SMEs Adopting AI |
|—————|——————————-|
| North America | 46% |
| Europe | 39% |
| Asia Pacific | 35% |
| Middle East | 32% |
| Latin America | 26% |
| Africa | 21% |
| Oceania | 19% |
| Caribbean | 17% |
| Central Asia | 15% |
| Eastern Europe| 13% |
Table Title: Advantages and Disadvantages of AI
Like any technology, AI comes with its own set of advantages and disadvantages. This table highlights the key benefits and limitations of AI, offering a balanced view of the impacts it has on society and industries.
| Advantages | Disadvantages |
|——————-|————————-|
| Increased efficiency and productivity | Job displacement and unemployment |
| Enhanced decision-making and accuracy | Ethical concerns and bias |
| Automation of repetitive tasks | High implementation and maintenance costs |
| Improved customer experiences | Data privacy and security risks |
| Advanced healthcare solutions | Lack of human emotional intelligence|
| Predictive analytics for business insights | Dependency on machine learning models |
The rapid development of AI technology has revolutionized industries and holds promise for a profoundly transformative future. From investments and applications to adoption rates and societal implications, this article aimed to shed light on various aspects of AI. The provided tables showcase the global impact and growing interest in AI, encompassing investment trends, job distributions, startup funding, and more. Despite the benefits AI brings, considerations such as ethical concerns and implementation costs also deserve attention. With continued advancements and informed decision-making, AI’s transformative potential can be harnessed for the betterment of society.
AI Training FAQ
What is AI training?
AI training refers to the process of teaching artificial intelligence models to learn and improve their performance over time. This involves providing large amounts of labeled data and utilizing algorithms to make the AI system learn from that data.
Why is AI training important?
AI training is important because it helps AI systems gain the ability to understand, interpret, and respond to complex patterns and datasets. It allows them to make accurate predictions and decisions, and ultimately improves their overall performance and effectiveness.
How does AI training work?
AI training involves feeding large amounts of data into algorithms, which are designed to extract meaningful patterns and features from the data. These algorithms then update the parameters of the AI model to make it better at predicting or classifying new inputs.
What types of data are used for AI training?
Various types of data can be used for AI training, including text, images, audio, and video. The choice of data depends on the specific AI task, such as natural language processing, computer vision, or speech recognition.
What is supervised learning in AI training?
Supervised learning is a type of AI training where the input data is labeled, meaning each data point is associated with a correct output value. During training, the AI model learns to map input data to the corresponding output labels, enabling it to make accurate predictions on unseen data.
What is unsupervised learning in AI training?
Unsupervised learning is a type of AI training where the input data is unlabeled, meaning there are no predefined output values. The goal is for the AI model to discover patterns or structure within the data on its own, without any specific guidance or supervision.
What is reinforcement learning in AI training?
Reinforcement learning is a type of AI training where an agent interacts with an environment and learns to take actions that maximize a reward signal. It involves a trial and error approach, where the AI model learns from its own experiences and receives feedback in the form of rewards or penalties.
What are the challenges in AI training?
AI training can be challenging due to the need for large amounts of high-quality labeled data, computational resources, and expertise in developing and fine-tuning AI models. Additionally, AI training may face ethical considerations, biases, and the interpretability of AI decisions.
How long does AI training take?
The duration of AI training depends on various factors, including the complexity of the AI task, the amount and quality of the data, the computational resources available, and the chosen AI algorithms. Training can range from hours to days or even weeks.
How often should AI models be retrained?
AI models should be retrained periodically to ensure their performance remains up-to-date. The frequency of retraining varies depending on the specific application and the rate at which the underlying data and patterns change. Some models may require retraining daily, while others can be retrained less frequently.