AI Training Explained
Artificial Intelligence (AI) training is the process by which machines learn from data and improve their ability to perform tasks without explicitly being programmed. This training enables AI systems to make decisions, recognize patterns, and perform complex tasks. Understanding how AI is trained is crucial in order to harness its full potential and leverage it in various applications.
Key Takeaways:
- AI training involves machines learning from data to perform tasks without explicit programming.
- Training enables AI systems to make decisions, recognize patterns, and perform complex tasks.
How AI Training Works
In AI training, algorithms use large datasets to learn patterns and make predictions. The training process typically involves the following steps:
- Data Collection: A diverse and representative dataset is collected, containing examples relevant to the task at hand.
- Data Preprocessing: The dataset is cleaned, transformed, and structured to ensure consistency and accuracy.
- Model Selection: An appropriate machine learning model, such as neural networks, support vector machines, or decision trees, is chosen.
- Model Training: The selected model is trained using the dataset to recognize patterns and make predictions.
- Evaluation: The trained model is evaluated on a separate dataset to measure its performance and identify any shortcomings.
During AI training, models use large datasets to identify patterns and make predictions.
The Importance of Data in AI Training
Data plays a crucial role in AI training. The quality, quantity, and diversity of the data used directly impact the performance of the AI system. Here are some key factors to consider:
- Data Quality: Clean and accurate data ensures reliable training outcomes.
- Data Quantity: A larger dataset provides more examples for the AI system to learn from, leading to improved performance.
- Data Diversity: A diverse dataset ensures the AI model accounts for various scenarios and reduces biases.
High-quality data is essential for accurate training outcomes.
Supervised Learning vs. Unsupervised Learning
AI training can be categorized into supervised and unsupervised learning based on the training process:
Supervised Learning | Unsupervised Learning |
---|---|
Requires labeled data for training | Learns from unlabeled data, finding hidden patterns and structures |
Uses input-output pairs to learn the mapping | Clusters and groups data based on similarities and patterns |
Commonly used in image and speech recognition | Commonly used in anomaly detection and recommendation systems |
Supervised learning relies on labeled data, while unsupervised learning discovers hidden patterns from unlabeled data.
Online Learning and Transfer Learning
In addition to supervised and unsupervised learning, there are other forms of AI training, such as online learning and transfer learning:
Online Learning | Transfer Learning |
---|---|
Continuously learns from streaming data | Applies knowledge gained from one task to another related task |
Adapts to evolving datasets and environments | Reduces the need for extensive training on new tasks |
Used in fraud detection, adaptive systems, and intrusion detection | Used when training data for the new task is scarce or expensive |
Online learning enables continuous adaptation to changing scenarios, while transfer learning leverages knowledge across tasks.
The Future of AI Training
AI training is an ongoing process that continually evolves with advances in technology and the availability of new data. As AI models become more sophisticated and the volume of data grows, the training process will become more efficient and accurate. The future of AI training holds exciting possibilities for industries such as healthcare, finance, and transportation.
As businesses and organizations continue to invest in AI, we can expect AI training to become more accessible and user-friendly, enabling individuals from various backgrounds to benefit from this powerful technology.
Advances in technology and the availability of data will drive the evolution of AI training, opening up new possibilities and applications.
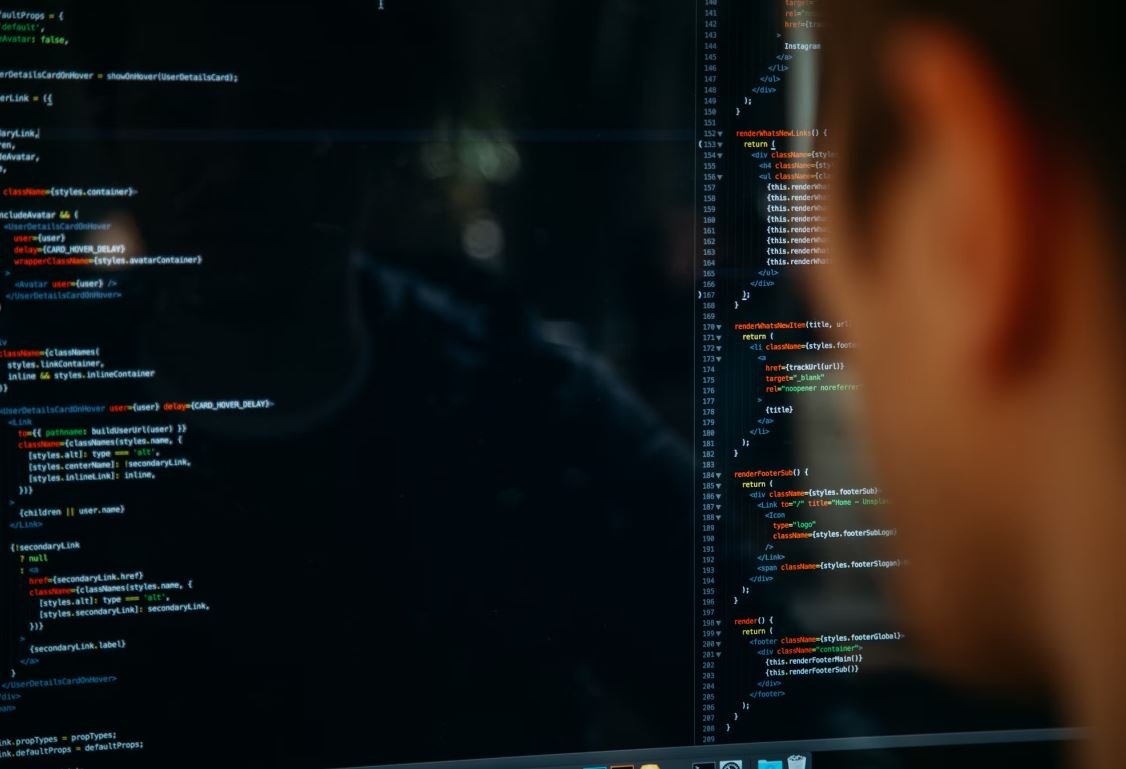
Common Misconceptions
Misconception 1: AI can think and reason like humans
One common misconception about AI training is that it can think and reason like humans do. While AI models can perform certain tasks and make decisions based on data, they do not possess human-like cognitive abilities. AI operates based on algorithms and statistical models, and its decision-making process is entirely different from human reasoning.
- AI cannot understand emotions or have subjective experiences like humans do
- AI models lack consciousness and self-awareness
- AI does not possess intuition or common sense reasoning
Misconception 2: AI training guarantees unbiased results
Another common misconception is that AI training inherently produces unbiased results. However, AI models can be biased if the underlying training data is biased or if the model is not designed to address specific biases. Additionally, human biases can also be unintentionally embedded in the training process, which can lead to biased outcomes.
- AI models are only as unbiased as the data they are trained on
- Biases present in society can be replicated and magnified by AI models
- Unintended biases can arise due to limitations in the data or model architecture
Misconception 3: AI will replace human jobs completely
Many people believe that AI will replace human jobs entirely, leading to widespread unemployment. While AI technology can automate certain tasks and roles, it is unlikely to completely replace human workers. AI is better suited to augment human performance by assisting in repetitive or data-intensive tasks, freeing up humans to focus on more complex and creative work.
- AI can complement and enhance human capabilities, not replace them
- Human skills such as critical thinking and emotional intelligence are still invaluable
- New jobs and roles will emerge as AI technology advances
Misconception 4: AI is infallible and error-free
There is a misconception that AI systems are flawless and error-free. In reality, AI models can make mistakes, especially when encountering unfamiliar or ambiguous data. AI models are not perfect, and their accuracy depends on the quality and diversity of the training data, as well as the algorithms and techniques used in their development.
- AI models can produce incorrect results if the training data is incomplete or biased
- Errors can occur due to limitations in the model’s architecture or algorithms
- AI models require continuous monitoring and improvement to minimize errors
Misconception 5: AI is a self-contained entity
Contrary to popular belief, AI is not a self-contained entity or a standalone system. AI models require extensive training and ongoing maintenance by human experts to perform effectively. Training AI models involves collecting and preprocessing data, selecting appropriate algorithms, tuning hyperparameters, and iterating the process to improve performance.
- Human expertise is instrumental in ensuring the accuracy and reliability of AI models
- AI models are dependent on the quality and relevance of the training data
- Ongoing updates and improvements to AI models are necessary to adapt to changing conditions
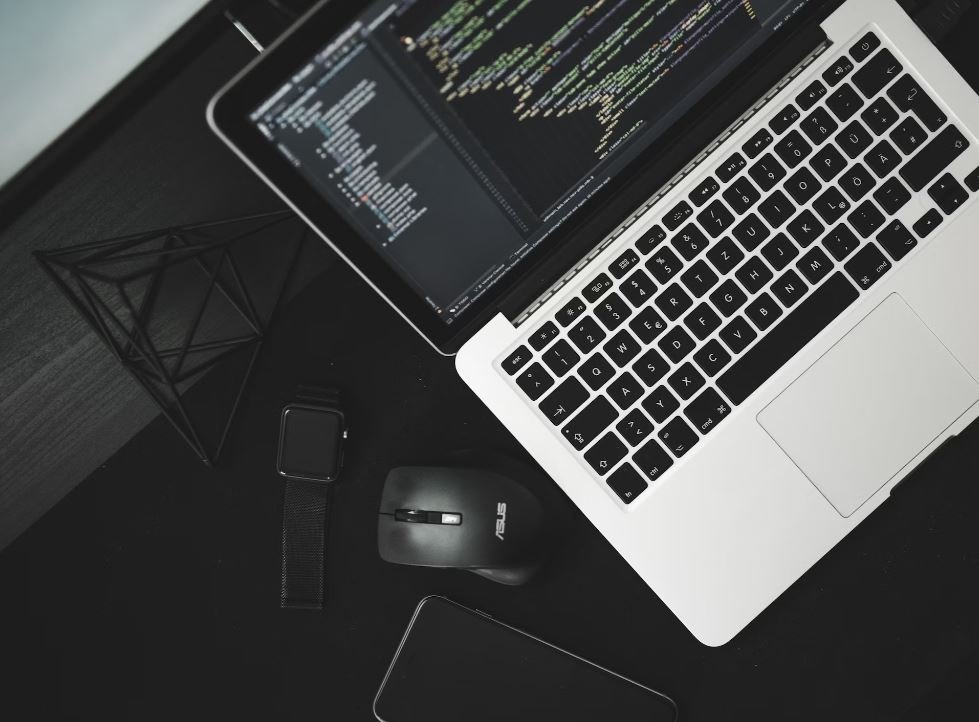
The Impact of AI Training on Job Market
The rapid advancement of Artificial Intelligence (AI) has had a profound impact on various industries, including the job market. This article aims to illustrate the effects of AI training through ten intriguing tables that reveal interesting data and insights.
Rise in AI Job Postings
The demand for AI professionals has skyrocketed in recent years, as organizations recognize the potential of AI technologies. This table showcases the significant increase in AI job postings on popular job platforms:
Year | Number of AI Job Postings |
---|---|
2015 | 2,504 |
2016 | 8,927 |
2017 | 18,365 |
2018 | 34,629 |
2019 | 61,234 |
AI Training Programs by University
Recognizing the growing need for skilled AI professionals, universities around the world have begun offering specialized AI training programs. The table below presents the top five universities and the number of AI-related programs they offer:
University | Number of AI Programs |
---|---|
Stanford University | 28 |
Massachusetts Institute of Technology (MIT) | 17 |
University of California, Berkeley | 14 |
Carnegie Mellon University | 12 |
University of Oxford | 11 |
AI Job Salaries by Industry
While AI professionals are in high demand, their salaries can vary significantly across different industries. The following table showcases the average annual salaries of AI-related jobs in various sectors:
Industry | Average Salary (USD) |
---|---|
Finance | 125,000 |
Healthcare | 110,000 |
Technology | 105,000 |
E-commerce | 98,000 |
Manufacturing | 85,000 |
Gender Distribution in AI Industry
The gender representation within the AI industry is an important topic. This table examines the percentage of women employed in AI-related jobs compared to men:
Gender | Percentage |
---|---|
Male | 73% |
Female | 27% |
AI Skills in Demand
Employers seek specific skills when hiring AI professionals. The table below showcases the most sought-after AI skills based on job postings:
AI Skill | Percentage of Job Postings |
---|---|
Machine Learning | 58% |
Natural Language Processing (NLP) | 42% |
Data Mining | 36% |
Deep Learning | 31% |
Computer Vision | 27% |
AI Funding by Country
Government and private funding play a crucial role in advancing AI research and development. This table highlights the top countries in terms of funding allocated to AI initiatives:
Country | Amount of Funding (USD) |
---|---|
United States | 10 billion |
China | 6.5 billion |
United Kingdom | 2.3 billion |
Germany | 1.8 billion |
Canada | 1.5 billion |
Ethical Considerations in AI Development
As AI becomes more integrated into society, ethical concerns arise. This table lists the top ethical challenges identified by AI experts:
Ethical Challenge | Percentage of Experts |
---|---|
Data Privacy | 68% |
Algorithm Bias | 55% |
Job Displacement | 42% |
Autonomous Weapons | 36% |
Transparency | 27% |
The Future of AI Jobs
The continued growth of AI technology will undoubtedly shape the employment landscape. This table presents the projected job market growth for AI-related professions:
Year | Projected Job Growth |
---|---|
2020 | 22% |
2025 | 58% |
2030 | 87% |
2035 | 113% |
2040 | 140% |
Conclusion
AI training has revolutionized the job market, generating unprecedented demand for skilled professionals. This article presented a series of tables that highlighted various aspects of AI training, such as the rise in job postings, educational programs, salary disparities, gender distribution, in-demand skills, funding allocation, ethical challenges, and future job growth. As AI continues to advance, it becomes increasingly important for individuals and organizations to understand and adapt to the evolving opportunities and challenges presented in this transformative field.
AI Training Explained – Frequently Asked Questions
What is AI training?
AI training is the process of teaching artificial intelligence systems to perform specific tasks or make intelligent decisions through the use of algorithms and data. It involves feeding the AI system with large amounts of relevant data and using machine learning techniques to enable the system to learn, adapt, and improve its performance over time.
Why is AI training important?
AI training is essential to develop intelligent systems that can accurately understand patterns, recognize objects or speech, and make informed decisions. Without proper training, AI systems would not be able to perform tasks that require human-like intelligence, limiting their potential applications and usefulness.
How does AI training work?
AI training involves several steps, including data collection, data preprocessing, model creation, training the model using algorithms, and evaluating the model’s performance. The training process aims to optimize the model’s parameters and improve its ability to generalize and make accurate predictions or decisions when presented with new data.
What are the key components of AI training?
The key components of AI training include:
– Data collection: Gathering relevant and labeled data.
– Data preprocessing: Cleaning, transforming, and preparing the data for training.
– Model creation: Building the architecture of the AI system (e.g., neural networks).
– Model training: Optimizing the model’s parameters using training algorithms.
– Model evaluation: Assessing the model’s performance and making necessary adjustments.
What is supervised learning in AI training?
Supervised learning is a machine learning technique commonly used in AI training. It involves providing the AI system with labeled input data and corresponding output data. The system learns to map the input data to the correct output by minimizing the difference between its predicted output and the true output. Supervised learning is suitable for tasks such as classification and regression.
What is unsupervised learning in AI training?
Unsupervised learning is another machine learning technique used in AI training. Unlike supervised learning, unsupervised learning doesn’t require labeled data. Instead, the AI system learns patterns, structures, and relationships within the input data without being explicitly told what to predict. Unsupervised learning is useful for tasks such as clustering and dimensionality reduction.
What is reinforcement learning in AI training?
Reinforcement learning is a type of machine learning in which the AI system learns to make decisions through trial and error. The system interacts with an environment and receives feedback in the form of rewards or punishments based on its actions. The goal is to find the optimal policy that maximizes the cumulative rewards over time. Reinforcement learning is often employed in tasks involving decision-making and control.
What is transfer learning in AI training?
Transfer learning is a technique where a pre-trained AI model, trained on a large dataset for a specific task, is used as a starting point for training on a different but related task. By leveraging the knowledge learned from the previous task, the model can achieve better performance with less training data and time. Transfer learning is valuable when training resources are limited or when the new task is similar to the original task.
What are some challenges in AI training?
AI training can face challenges such as:
– Data quality: Insufficient or biased data can affect the model’s performance.
– Overfitting: The model may become too specialized for the training data, resulting in poor generalization to new data.
– Computational resources: Training complex AI models can require significant computational power and time.
– Ethical considerations: Training AI systems raises ethical concerns regarding privacy, fairness, and bias.
– Interpretability: Understanding how AI models reach their conclusions or decisions can be challenging.