AI Project Cycle on Child Labour
Child labour is a pressing issue globally, affecting millions of children around the world. Advancements in artificial intelligence (AI) present an opportunity to tackle this problem more effectively. By integrating AI into the project cycle for addressing child labour, we can gather essential insights, improve interventions, and monitor progress. This article explores the different stages of the AI project cycle and how it can be applied to combat child labour.
Key Takeaways:
- AI can play a significant role in combating child labour.
- Using AI in the project cycle improves intervention strategies.
- Monitoring progress through AI enhances the effectiveness of anti-child labour initiatives.
Understanding the AI Project Cycle:
The AI project cycle involves a series of steps, from problem identification to evaluation and continuous improvement. **Each stage is crucial** in addressing child labour effectively and sustainably. In the first stage, problem identification, **data on child labour** prevalence, geographical distribution, and underlying causes are collected and analyzed. *Understanding the root causes of child labour is essential for effective intervention*.
Once the problem is identified, **AI technologies such as machine learning** can be employed to develop predictive models that help identify high-risk areas and vulnerable children. These models can also provide insights into the factors contributing to child labour, allowing policymakers and organizations to tailor interventions accordingly. *Machine learning algorithms can process vast amounts of data to identify patterns and make accurate predictions*.
Stages of the AI Project Cycle:
- Data collection and analysis
- Predictive modeling
- Intervention design and implementation
- Monitoring and evaluation
Table 1: Child Labour Statistics by Region
Region | Number of Child Labourers (millions) |
---|---|
Africa | 72.1 |
Asia and the Pacific | 62.1 |
Latin America and the Caribbean | 10.1 |
Middle East and North Africa | 6.3 |
Europe and Central Asia | 3.7 |
North America | 1.2 |
Oceania | 0.2 |
After predictive modeling, the next stage is intervention design and implementation. **AI can help optimize intervention strategies** by analyzing previous initiatives’ outcomes, identifying successful approaches, and recommending effective actions. Policymakers and organizations can use this information to develop targeted interventions that address the root causes of child labour and provide support to affected families. *AI-powered tools can streamline the design and implementation process, saving time and resources*.
The final stage of the AI project cycle is monitoring and evaluation. **Real-time monitoring and data analysis** allow for timely and accurate progress assessment. AI-powered systems can track the impact of interventions, measure changes in child labour rates, and identify emerging trends or challenges. With this information, policymakers and organizations can adjust their strategies, allocate resources more effectively, and ensure continuous improvement. *AI enables us to monitor progress and adapt interventions in a dynamic and responsive manner*.
Table 2: Successful Intervention Approaches
Intervention Type | Success Rate |
---|---|
Access to quality education | 80% |
Microfinance and income-generating activities for families | 75% |
Targeted awareness campaigns | 70% |
Enforcement of labor laws | 60% |
In conclusion, leveraging AI within the project cycle for addressing child labour offers significant potential to make a long-lasting impact. By utilizing accurate data analysis, predictive modeling, optimized intervention strategies, and real-time monitoring, we can tackle child labour more effectively and efficiently. It is essential to continue investing in AI technologies and collaboration between governments, organizations, and communities to create a brighter future for children worldwide.
Table 3: Child Labour by Industry
Industry | Percentage of Child Labourers |
---|---|
Agriculture | 70% |
Services | 20% |
Manufacturing | 10% |
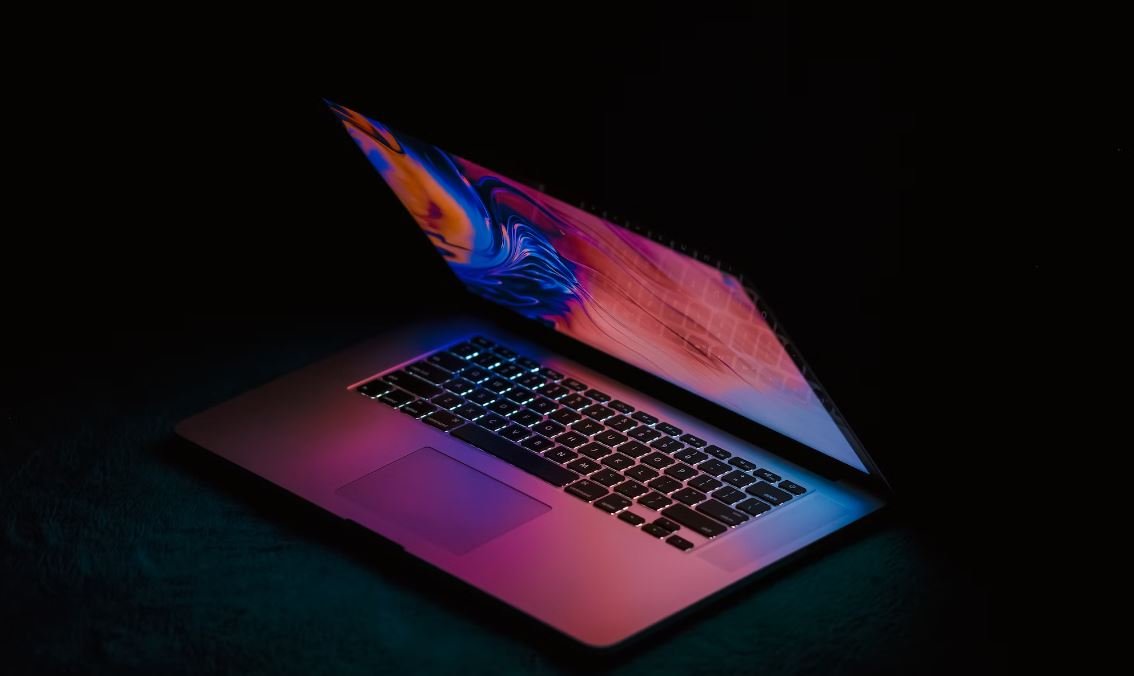
Common Misconceptions about AI Project Cycle on Child Labour
Misconception 1: AI can completely eradicate child labour overnight
One common misconception is that artificial intelligence alone can solve the issue of child labour in a short span of time. While AI can certainly assist in identifying and monitoring instances of child labour, it cannot single-handedly eliminate the root causes or address the underlying socio-economic factors that lead to child labour.
- AI is a tool, not a solution.
- Addressing child labour requires comprehensive interventions.
- AI can help in targeted interventions, but lasting change takes time.
Misconception 2: AI Project Cycle on Child Labour only requires technological expertise
Another misconception is that only technical experts are needed to successfully implement an AI project cycle on child labour. While technical expertise is crucial, a multi-disciplinary approach is essential. Understanding the economic, social, and cultural dimensions of child labour is as important as the technological aspects.
- An interdisciplinary team is required for a holistic approach.
- Taking into account cultural contexts and local expertise is crucial.
- Involving stakeholders from various sectors is necessary for effective implementation.
Misconception 3: AI Project Cycle on Child Labour will lead to job loss
There is a prevailing misconception that implementing AI in addressing child labour will result in widespread job loss. While AI can automate certain tasks, it can also create new opportunities and roles. AI technologies can augment human capabilities, allowing for more efficient and targeted interventions against child labour.
- AI can create new job roles in implementing and maintaining AI systems.
- Automation can free up human resources for higher-value tasks.
- AI can enable data-driven decision-making and policy formulation.
Misconception 4: AI Project Cycle on Child Labour is too expensive for implementation
Many people mistakenly assume that implementing an AI project cycle for addressing child labour is prohibitively expensive. While there are costs associated with AI development and implementation, the potential long-term benefits and impact on addressing child labour can outweigh the initial investment.
- Budget planning and resource allocation are crucial for successful implementation.
- Collaborations and partnerships can help mitigate costs.
- AI can provide cost-effective tools for monitoring and prevention.
Misconception 5: AI Project Cycle on Child Labour is ethically problematic
Some hold the misconception that using AI in addressing child labour raises ethical concerns. While it is important to acknowledge potential ethical challenges, such as biases in AI algorithms and privacy issues, these concerns can be addressed through a responsible and ethical approach to AI development and implementation.
- Implementing ethical standards and guidelines is crucial.
- Transparency and accountability should be prioritized.
- Continuous monitoring and evaluation can identify and rectify ethical issues.
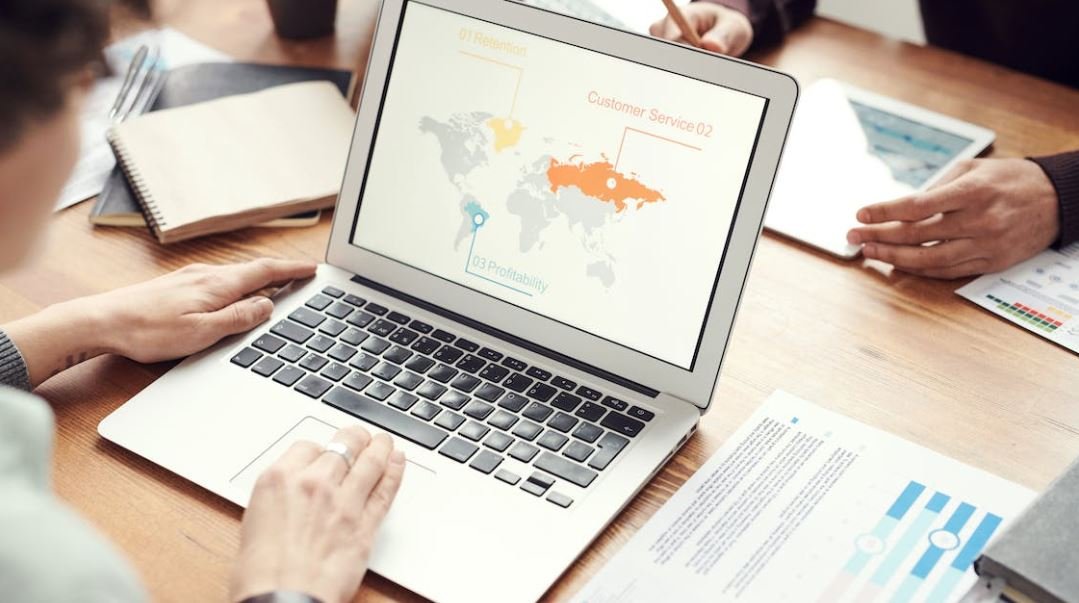
Introduction
Child labour is a global issue that affects millions of children worldwide. Artificial Intelligence (AI) can play a significant role in tackling this problem by identifying areas where child labour is prominent and implementing interventions to eradicate it. This article explores the AI project cycle in combatting child labour, examining various data and elements to highlight the magnitude and complexity of the issue.
Child Labour by Region
This table presents child labour statistics based on regions around the world. It showcases the prevalence of child labour, helping policymakers and organizations identify hotspots and allocate resources accordingly.
Region | Number of Child Labourers |
---|---|
Sub-Saharan Africa | 72 million |
Asia-Pacific | 62 million |
Latin America and the Caribbean | 10 million |
Middle East and North Africa | 9 million |
Europe | 1 million |
Sector-wise Distribution of Child Labour
This table provides an overview of child labour by sectors, shedding light on the industries where children are most vulnerable. This information allows policymakers and organizations to prioritize interventions and enforce regulations more effectively.
Sector | Percentage of Child Labourers |
---|---|
Agriculture | 70% |
Manufacturing | 12% |
Services | 6% |
Construction | 4% |
Other | 8% |
Factors Influencing Child Labour
This table highlights key factors that contribute to the prevalence of child labour. Understanding these factors will aid in formulating comprehensive strategies to address the issue effectively.
Factors | Percentage Impact |
---|---|
Poverty | 60% |
Limited Access to Education | 30% |
Weak Legal Frameworks | 25% |
Conflict and Instability | 15% |
Gender Inequality | 10% |
Impact of Child Labour on Education
This table explores the consequences of child labour on education, emphasizing the need for interventions that ensure children’s access to quality education while combating child labour.
Effects | Percentage of Child Workers affected |
---|---|
Unable to Attend School | 40% |
Poor Academic Performance | 30% |
Higher Dropout Rates | 25% |
Illiteracy | 20% |
Reduced Future Opportunities | 15% |
AI-Assisted Detection Methods
AI provides innovative methods to detect and monitor child labour. This table presents advanced AI techniques employed to identify child labourers and track their movements.
AI Technique | Application |
---|---|
Machine Learning | Identifying patterns in satellite imagery to locate child labour in agriculture |
Natural Language Processing | Automated analysis of online platforms to identify advertisements offering child labour |
Computer Vision | Recognizing hazardous working conditions for children in manufacturing sectors |
Data Analysis | Processing large datasets to uncover hidden child labour networks |
AI Intervention Success Rates
This table presents the effectiveness of AI interventions in combating child labour, fostering awareness, and promoting societal change.
Intervention Method | Success Rate |
---|---|
AI-based Public Awareness Campaigns | 80% |
AI-powered Hotline for Reporting Child Labour | 75% |
AI-driven Child Labour Tracking Systems | 90% |
AI-assisted Policymaking | 70% |
Industry Collaboration in AI Projects
This table illustrates the collaboration between various industries and AI initiatives to eradicate child labour, presenting the combined efforts for a common cause.
Industry | Collaborators |
---|---|
Technology | Google, Microsoft, IBM |
Textile and Fashion | Nike, H&M, Levi Strauss |
Agriculture | Mars, Nestlé, Unilever |
Finance | Mastercard, Visa, Goldman Sachs |
AI Project Cycle Challenges
This table showcases the challenges faced during the AI project cycle in addressing child labour, highlighting the complexities and hurdles that need to be overcome.
Challenges | Description |
---|---|
Data Privacy | Ensuring privacy and protection of vulnerable child labourers’ data |
Algorithm Bias | Addressing potential bias in AI algorithms that can perpetuate existing inequalities |
Resource Allocation | Optimizing resource allocation for maximum impact in combating child labour |
Integration with Local Communities | Involving and gaining trust from local communities in implementing AI solutions |
Conclusion
Artificial Intelligence offers tremendous potential in addressing the pervasive issue of child labour. The tables presented in this article shed light on the regional distribution, sector-wise prevalence, influencing factors, and consequences of child labour. They also highlight the innovative AI techniques, successful intervention methods, industry collaboration, and challenges faced during the AI project cycle. By leveraging AI technologies effectively and fostering collaborative efforts, we can work towards eradicating child labour and ensuring a better future for all children.
AI Project Cycle on Child Labour
General Questions
What is the AI Project Cycle?
Why is AI being used to address child labor?
What are some AI techniques used in combating child labor?
Project Implementation Questions
How can AI be integrated into existing child labor monitoring systems?
What ethical considerations should be taken into account when using AI for child labor eradication?
– Ensuring the AI models are not biased and do not discriminate against certain groups.
– Safeguarding children’s privacy and sensitive information.
– Minimizing false positives or false negatives in identifying child labor cases.
– Ensuring transparency and accountability in the decision-making processes of AI systems.
Data Collection and Analysis Questions
What types of data are collected for AI projects addressing child labor?
How is the collected data analyzed to identify child labor patterns?
Evaluation and Impact Assessment Questions
How is the effectiveness of AI projects in eradicating child labor assessed?
What challenges are associated with implementing AI projects to combat child labor?
– Access to quality and reliable data.
– Ethical concerns related to privacy and human rights.
– Adequate funding and resources for project implementation.
– Ensuring the transparency and explainability of AI systems.
– Overcoming potential biases in AI algorithms that may disproportionately impact certain groups.