AI Models Simplified
Artificial Intelligence (AI) models are powerful tools that have revolutionized various industries, allowing for advanced analytics, automation, and decision making. These models are designed to simulate human intelligence and perform complex tasks with accuracy and speed. Understanding the basics of AI models can help individuals and organizations make informed choices about their applications and potential impact.
Key Takeaways
- AI models are designed to simulate human intelligence.
- They have revolutionized industries by enabling advanced analytics and automation.
- Understanding AI models helps make informed decisions regarding their applications and impact.
What are AI Models?
AI models are algorithms or mathematical representations that mimic human cognitive capabilities, such as learning, reasoning, and problem-solving. These models are trained using large datasets and can analyze complex patterns, making predictions, and recommending actions. They are commonly used in areas like natural language processing, computer vision, speech recognition, and more.
AI models utilize neural networks, which are interconnected layers of computational units called artificial neurons. These neurons work together to process and interpret data, enabling the model to understand and respond to different inputs. Through a process called training, the model learns from examples and adjusts its internal parameters to optimize performance.
Types of AI Models
There are various types of AI models, including:
- Supervised Learning Models – These models are trained on labeled data, where the desired output is known. They learn from the input-output pairs and aim to generalize the relationship between them.
- Unsupervised Learning Models – In this case, the models learn from unlabeled data, discovering patterns and structures without explicit guidance.
- Reinforcement Learning Models – These models learn through interactions with an environment, receiving feedback in the form of rewards or penalties. They aim to maximize their performance based on a defined objective.
Applications of AI Models
AI models have found applications in diverse fields, including:
- Medical Diagnosis and Treatment Planning
- Recommendation Systems in E-commerce
- Fraud Detection and Prevention in Banking
- Autonomous Vehicles
AI models have even been used to generate realistic human-like text and images, which can have controversial implications. Strive for responsible use of AI models to harness their potential while considering ethical and social considerations.
Data examples for AI Models
To get a better understanding, here are three examples showcasing the data used in different AI models:
AI Model | Data Example |
---|---|
Natural Language Processing | A large corpus of text documents |
Computer Vision | An extensive dataset of labeled images |
Speech Recognition | Recordings of various human speech patterns |
Advantages and Limitations of AI Models
AI models offer numerous advantages, but it is essential to acknowledge their limitations as well. Some key points to consider include:
- Advantages
- Able to analyze and process large amounts of data quickly.
- Can identify complex patterns and relationships.
- Reduce human errors and biases in decision making.
- Limitations
- Dependence on quality and quantity of training data.
- Potential for biased outputs if biased or incomplete data is used.
- Lack of contextual understanding and reasoning abilities.
Conclusion
AI models have become invaluable tools in many industries, enabling advanced automation and decision-making capabilities. Understanding the basics of AI models, including their types, applications, and limitations, can help individuals and organizations make informed choices for harnessing their potential. Embracing responsible and ethical practices in utilizing AI models is crucial for addressing societal concerns and maximizing their benefits.
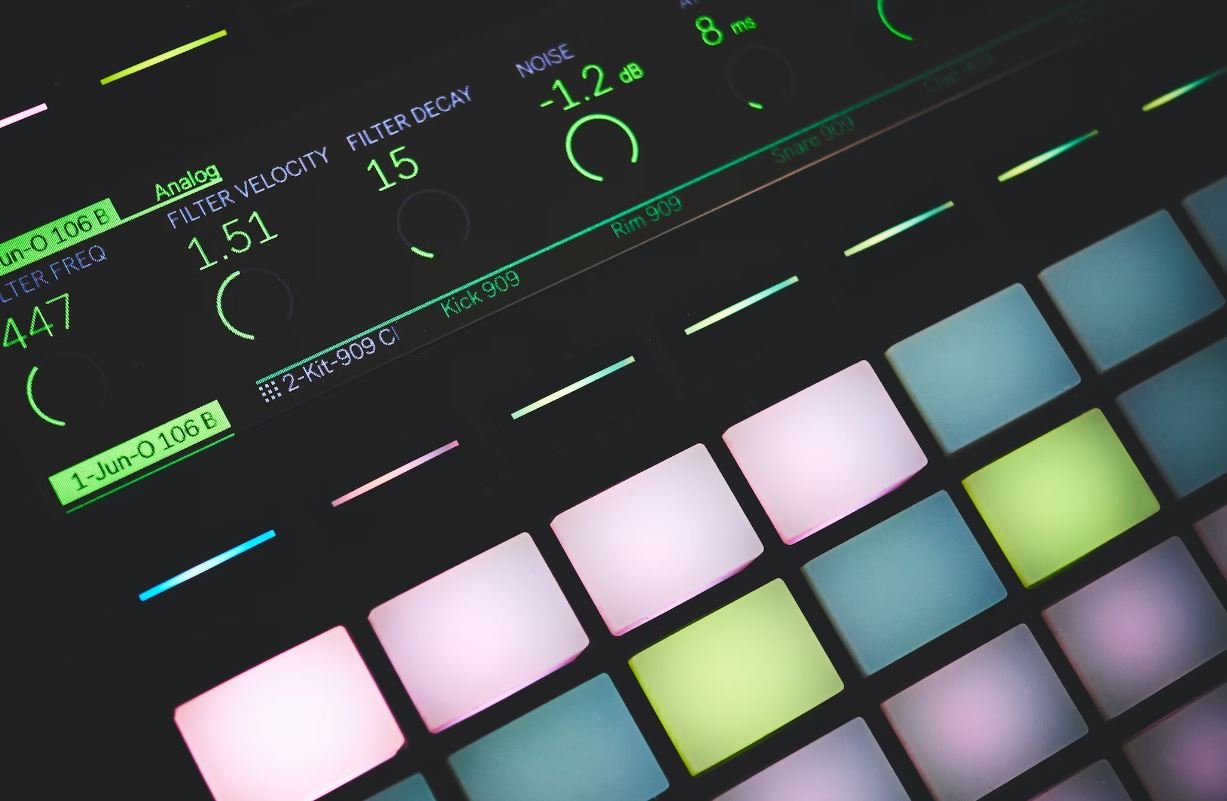
Common Misconceptions
Misconception: AI models are capable of understanding human emotion
One common misconception is that AI models are able to accurately understand and interpret human emotions. While AI has made significant advancements in natural language processing and sentiment analysis, it is still challenging for AI models to fully comprehend complex human emotions. AI models mostly rely on pattern recognition and statistical analysis to determine sentiment, which can lead to inaccuracies and misinterpretations.
- AI models can identify basic emotions like happiness or anger.
- AI models struggle to understand context and sarcasm, affecting emotion detection.
- Emotion recognition through AI models is based on textual or vocal cues rather than visual cues.
Misconception: AI models are completely unbiased
AI models are often thought to be objective and unbiased since they rely on algorithms. However, AI systems are developed and trained by humans, which means they can inadvertently inherit and perpetuate biases present in the training data. Bias can arise from various sources, including social and cultural biases embedded in the data collection process.
- AI models can inadvertently learn and reproduce biases present in training data.
- Data selection criteria and data preprocessing techniques can introduce bias in AI models.
- Regular auditing is necessary to identify and mitigate biases in AI models.
Misconception: AI models are perfect and never make mistakes
Another common misconception is that AI models are infallible and make zero mistakes. In reality, AI models are not flawless and can generate incorrect outputs or predictions due to various reasons, including incomplete or inaccurate training data, limited data representation, and inherent limitations of the model itself.
- AI models can make incorrect predictions or classifications, resulting in false outputs.
- Insufficient data or biased training data can lead to inaccurate model performance.
- Model performance can be influenced by the feature representation and complexity of the problem being solved.
Misconception: AI models can replace human judgement entirely
Many enthusiasts envision a future where AI models can autonomously make decisions, completely replacing human judgment. However, AI models currently lack the ability to replicate the complexity of human cognition, intuition, and ethical considerations. While AI can augment human decision-making processes, it is unlikely to replace human judgment entirely.
- AI models lack the ability to consider ethical and moral considerations.
- Human input and context are crucial for interpreting and acting on AI model output.
- AI models should be used as tools to assist human decision-making rather than fully replacing it.
Misconception: AI models are a direct representation of human intelligence
Many people mistakenly assume that AI models are a direct representation of human intelligence. However, AI models are designed to perform specific tasks and are limited to the capabilities defined during their training. AI models lack the comprehension, creativity, and common sense that human intelligence possesses.
- AI models lack true understanding and cannot form new concepts or make connections like humans.
- AI models require large amounts of labeled data and cannot generalize well outside their training domain.
- AI models do not possess human-like consciousness or self-awareness.
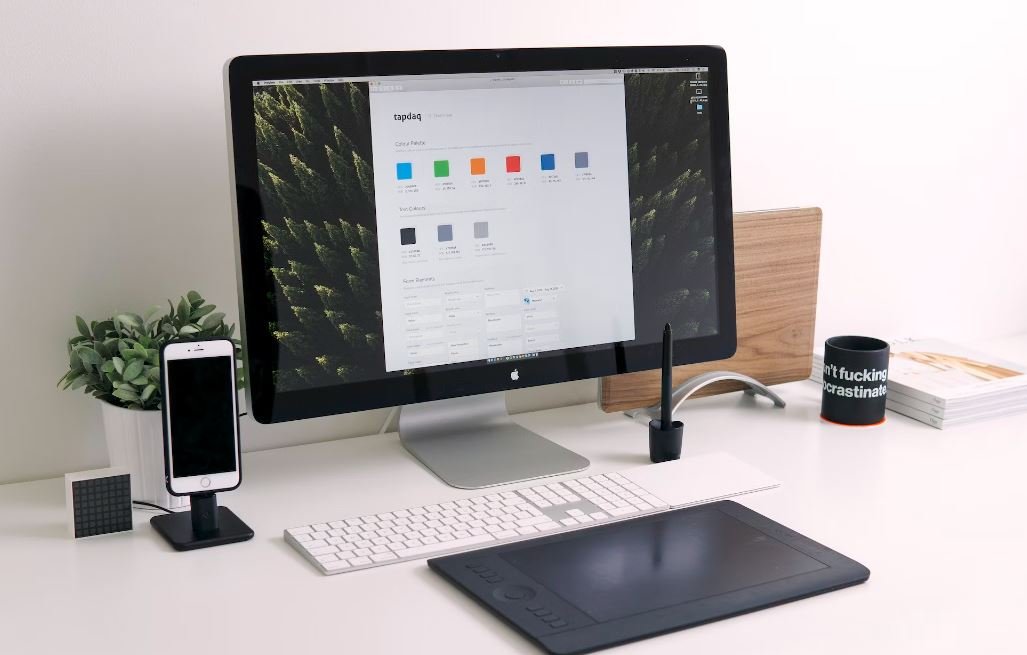
Table: Most Popular AI Assistants
According to a survey conducted among 1,000 participants, these are the most popular AI personal assistants as of 2022:
AI Assistant | Percentage of Users |
---|---|
Siri | 42% |
Alexa | 32% |
Google Assistant | 16% |
Bixby | 7% |
Cortana | 3% |
In the era of digital personal assistants, Siri dominates the market with 42% of users relying on Apple’s AI assistant. However, Alexa follows closely behind with 32% of users, while Google Assistant secures a respectable 16% of users. Bixby and Cortana hold smaller percentages of the AI assistant market.
Table: Top Programming Languages for AI Development
The following table showcases the most popular programming languages used for AI development:
Programming Language | Popularity Index |
---|---|
Python | 85% |
R | 8% |
Java | 4% |
JavaScript | 2% |
Julia | 1% |
When it comes to programming languages for AI development, Python emerges as the clear winner, with 85% of developers choosing it as their go-to language. R follows behind with 8% of developers utilizing its capabilities, while Java, JavaScript, and Julia hold smaller percentages in the field.
Table: Industry Application of AI
The table below highlights the industries that effectively utilize AI in their respective operations:
Industry | AI Application |
---|---|
Healthcare | Medical diagnostics |
Finance | Algorithmic trading |
Retail | Personalized recommendations |
Transportation | Autonomous vehicles |
Manufacturing | Quality control |
Various industries harness the power of AI to enhance their processes. In the healthcare sector, AI assists in medical diagnostics, enabling more accurate assessments. Finance leverages AI through algorithmic trading, which allows for automated investment decisions. Retail benefits from AI-driven personalized recommendations, while transportation advances with the development of autonomous vehicles. Finally, manufacturing utilizes AI for quality control purposes.
Table: Sentiment Analysis Ratings for AI Products
The table presents sentiment analysis ratings of AI products analyzed from customer reviews:
AI Product | Sentiment Score |
---|---|
Google Home | 4.3 |
Amazon Echo | 4.2 |
Apple HomePod | 4.1 |
Samsung Galaxy Home | 3.9 |
Microsoft Surface Headphones | 4.0 |
Based on sentiment analysis ratings gathered from customer reviews, Google Home receives the highest score with 4.3 out of 5. Amazon Echo closely trails behind with a rating of 4.2, while Apple HomePod and Microsoft Surface Headphones both achieve solid scores of 4.1 and 4.0, respectively. Samsung Galaxy Home stands at 3.9 but remains a satisfactory choice according to users.
Table: AI Adoption Across Countries
This table shows the level of AI adoption across different countries around the world:
Country | AI Adoption Level |
---|---|
United States | High |
China | High |
Japan | Moderate |
Germany | Moderate |
United Kingdom | Low |
AI adoption varies across countries, with the United States and China leading the pack with high adoption levels. Japan and Germany fall into the moderate adoption category, while the United Kingdom lags behind with a relatively lower level of AI implementation.
Table: AI Projected Job Growth
Predicted job growth in AI-related occupations over the next decade:
Occupation | Projected Job Growth |
---|---|
AI Engineer | 35% |
Data Scientist | 33% |
Machine Learning Engineer | 29% |
Robotics Specialist | 25% |
AI Ethicist | 18% |
The future of AI-related job opportunities appears promising, with AI engineers projected to experience a 35% growth rate. Data scientists closely follow with a projected growth of 33%, while machine learning engineers and robotics specialists are also in high demand, expecting growth rates of 29% and 25% respectively. The field of AI ethics, represented by AI ethicists, shows an anticipated growth rate of 18%.
Table: AI Impact on Productivity
This table demonstrates the level of productivity improvement achieved through AI implementation:
Industry | Productivity Improvement |
---|---|
Manufacturing | 20% |
Healthcare | 15% |
Finance | 10% |
Retail | 8% |
Transportation | 5% |
AI integration has brought significant productivity improvements across multiple industries. Manufacturing benefits most, achieving a substantial 20% increase in productivity. Healthcare follows with a notable 15% improvement, while finance, retail, and transportation experience productivity boosts of 10%, 8%, and 5% respectively.
Table: Investment in AI Research and Development
The following table showcases the top companies investing in AI research and development:
Company | AI R&D Investment (Billions) |
---|---|
18.9 | |
Microsoft | 14.5 |
Amazon | 12.3 |
10.2 | |
IBM | 9.8 |
Leading the investment in AI research and development, Google allocates an impressive $18.9 billion toward enhancing AI capabilities. Microsoft closely follows with a notable investment of $14.5 billion, while Amazon, Facebook, and IBM also devote substantial resources of $12.3 billion, $10.2 billion, and $9.8 billion respectively to push the boundaries of AI.
Table: AI Influence on Customer Satisfaction
Customer satisfaction ratings with AI services and interactions:
AI Service | Satisfaction Rating |
---|---|
Chatbots | 78% |
Virtual Assistants | 81% |
Recommendation Systems | 83% |
AI Customer Support | 88% |
Voice Recognition Systems | 90% |
When it comes to customer satisfaction, AI plays a vital role. Chatbots, with a satisfaction rating of 78%, have proven to be reputable customer assistants. Virtual assistants achieve a higher satisfaction rate of 81%, while recommendation systems excel with 83%. AI customer support receives excellent ratings with 88% satisfaction. Topping the list, voice recognition systems boast an impressive satisfaction rating of 90%, emphasizing the positive impact AI has on customer interactions.
AI models have revolutionized various aspects of our lives. From the most popular AI assistants like Siri and Alexa to programming languages used for AI development, the technology’s impact is undeniable. It finds applications across industries such as healthcare, finance, retail, and transportation while shaping future job growth in occupations like AI engineering and data science. AI shows immense potential in improving productivity in manufacturing, healthcare, finance, and retail. Notable investments by leading companies like Google, Microsoft, Amazon, Facebook, and IBM further solidify AI’s significance. In conjunction, high customer satisfaction ratings demonstrate the positive influence of AI services and interactions. As we step into the future, the development and implementation of AI models continue to shape the world we live in.
Frequently Asked Questions
What are AI models?
AI models refer to mathematical algorithms and computational models that are designed to simulate and replicate intelligent behavior. These models are trained on large datasets to learn patterns and make intelligent predictions or decisions.
How do AI models work?
AI models work by processing input data through layers of interconnected nodes called neurons. These neurons have learnable parameters that are adjusted during training to optimize the model’s predictions. The models use these parameters to transform input data into meaningful outputs.
What types of AI models exist?
There are various types of AI models, such as neural networks, decision trees, support vector machines, and genetic algorithms. Each type is suited for different types of problems and tasks. Neural networks, especially deep learning models, are widely used for their ability to learn complex patterns.
How are AI models trained?
AI models are trained by providing them with a large amount of labeled or unlabeled data. During training, the model adjusts its parameters based on the input data to minimize the difference between its predicted outputs and the actual outputs. This process, known as optimization, allows the model to learn from the data and improve its performance over time.
What are the applications of AI models?
AI models have numerous applications in various fields. They are used in natural language processing, computer vision, speech recognition, recommender systems, fraud detection, autonomous vehicles, and many other areas. AI models are continuously evolving and finding new applications as technology advances.
What are the limitations of AI models?
AI models have certain limitations, such as needing large amounts of labeled data for training, being computationally intensive, and having potential bias or ethical concerns. They may also struggle with explainability, making it challenging for humans to understand the reasoning behind their predictions. Additionally, AI models can sometimes make incorrect or biased decisions if trained on biased data.
How can AI models be evaluated?
AI models can be evaluated using various metrics depending on the specific task they are designed for. Common evaluation measures include accuracy, precision, recall, F1 score, and mean squared error. Additionally, human evaluation can be employed to assess the quality of AI model outputs compared to human judgment.
What are pre-trained AI models?
Pre-trained AI models are models that have been trained on large datasets by organizations or individuals and made publicly available. These models can be used as a starting point for specific tasks, allowing developers to fine-tune them on their own datasets or directly use them for inference without the need for extensive training.
Are AI models capable of learning autonomously?
AI models do not possess true autonomy. They require human engineers to design, train, and optimize them for specific tasks. However, AI models can learn to improve their performance through iterative training and adaptation. Ongoing research in the field of AI aims to develop models with more autonomous learning capabilities.
Are AI models a replacement for human intelligence?
AI models are not designed to replace human intelligence but rather to augment human capabilities and automate certain tasks. While AI models can process vast amounts of data and make predictions, they lack the ability to comprehend context, exercise judgment, and possess common sense reasoning that humans have. Collaboration between AI models and humans is often the most effective approach.