AI Models in Project Cycle
AI models have revolutionized the project management process by providing powerful tools and techniques to enhance decision-making, optimize resource allocation, and improve overall project outcomes. With advances in artificial intelligence, project managers can now leverage AI models throughout the project cycle to gain valuable insights and make informed decisions.
Key Takeaways
- AI models enhance decision-making, resource allocation, and project outcomes.
- Using AI models throughout the project cycle can provide valuable insights.
- AI models automate repetitive tasks, saving time and effort.
- Project managers must consider ethical implications when using AI models.
Throughout the project cycle, AI models play a crucial role in various stages, including project initiation, planning, execution, monitoring, and closure. In project initiation, AI models can assist in evaluating the feasibility of the project by analyzing historical data and predicting success factors.
*AI models utilize advanced algorithms to process large volumes of data and identify patterns that humans may overlook at first glance.
Project Initiation
In project planning, AI models can help with resource allocation, risk assessment, and cost estimation. These models analyze historical project data, organizational resources, and external factors to optimize the allocation of resources and ensure successful project delivery.
Project Planning
**AI models improve the accuracy of cost estimation by analyzing historical project data and accounting for various factors that could impact project costs.
Project Execution
During project execution, AI models assist in automating repetitive tasks, improving efficiency, and enabling real-time monitoring. These models can identify anomalies, predict potential bottlenecks, and suggest optimal solutions.
Project Monitoring
AI Model Benefits | Examples |
---|---|
Automate repetitive tasks | Chatbots for customer support |
Real-time monitoring | Monitoring project progress in real-time |
Anomaly detection | Identifying unusual patterns or behaviors |
During the project closure phase, AI models can provide valuable insights and lessons learned by analyzing data from completed projects. These insights can be used to improve future project outcomes and inform decision-making.
Project Closure
**AI models generate actionable insights by analyzing large volumes of project data, identifying success factors, and determining areas for improvement.
Ethical Considerations
While leveraging AI models in project management offers numerous benefits, project managers must also consider the ethical implications. AI models must be trained on unbiased data to ensure fair decision-making, and their use should prioritize transparency, accountability, and respect for privacy.
Conclusion
AI models have emerged as valuable tools in the project management process, revolutionizing decision-making, resource allocation, and overall project outcomes. By leveraging AI models from project initiation to closure, project managers can gain valuable insights, save time and effort, and make informed decisions. However, ethical considerations should always be at the forefront when implementing AI models in project management.
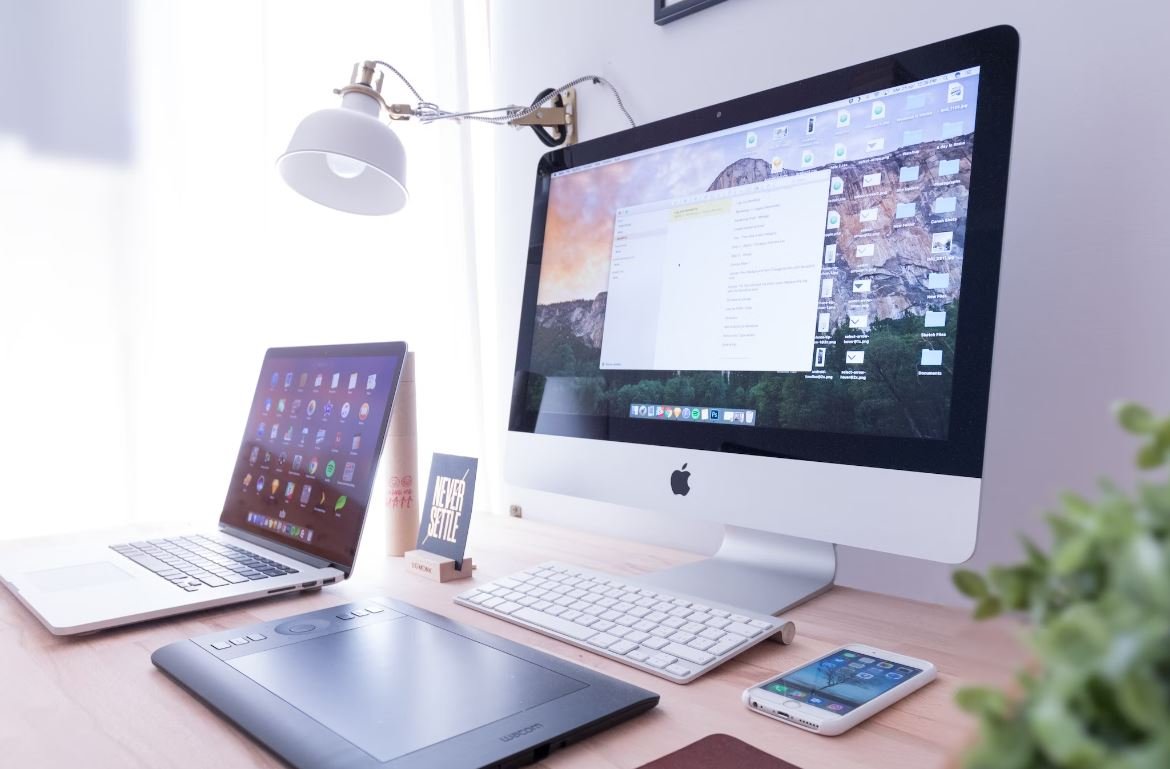
Common Misconceptions
AI Models in Project Cycle
There are several common misconceptions surrounding the use of AI models in the project cycle. One of the main misconceptions is that AI models can replace human decision-making entirely. While AI models can provide valuable insights and automate certain tasks, they are not capable of replicating the complexity of human judgment.
- AI models are tools to support decision-making, not replace it
- AI models have limitations and can be biased
- Human oversight is necessary to ensure the ethical use of AI models
Another common misconception is that AI models are completely objective and unbiased. However, AI models are trained on existing data, which may contain inherent biases. These biases can be reinforced and perpetuated by the model, leading to biased outcomes. It is crucial to carefully select and preprocess training data to mitigate biases in AI models.
- AI models can inherit and perpetuate biases in the data they are trained on
- Data preprocessing techniques can be used to mitigate biases in AI models
- Ongoing monitoring and evaluation are needed to detect and address biases in AI models
Additionally, some people believe that AI models are a one-size-fits-all solution that can be easily applied to any project. However, AI models require customization and fine-tuning to specific project requirements. Different projects have unique characteristics, and AI models need to be tailored accordingly.
- AI models need customization and fine-tuning to fit specific project requirements
- Domain expertise is crucial in designing and implementing AI models
- Regular updates and maintenance are necessary to keep AI models relevant and effective
Another misconception is that AI models always provide accurate predictions or solutions. While AI models can be powerful tools for analysis and forecasting, they are not infallible. Accuracy depends on the quality of input data, model selection, and appropriate application. It is important to consider the uncertainties and potential errors associated with AI models.
- AI models are not always 100% accurate and can make errors
- Validation and testing are crucial to assess the reliability of AI models
- Human expertise is essential in interpreting and contextualizing AI model outputs
Lastly, some individuals think that AI models can be simply implemented without considering the ethical implications. However, the ethical use of AI models is essential to ensure fairness, transparency, and accountability. Ethical considerations should be integrated into the entire project cycle to avoid unintended consequences and potential harm.
- Ethical guidelines and frameworks should be established for AI model implementation
- Transparency and interpretability of AI models are crucial for trust and accountability
- Ongoing ethical assessments should be conducted to address emerging ethical dilemmas
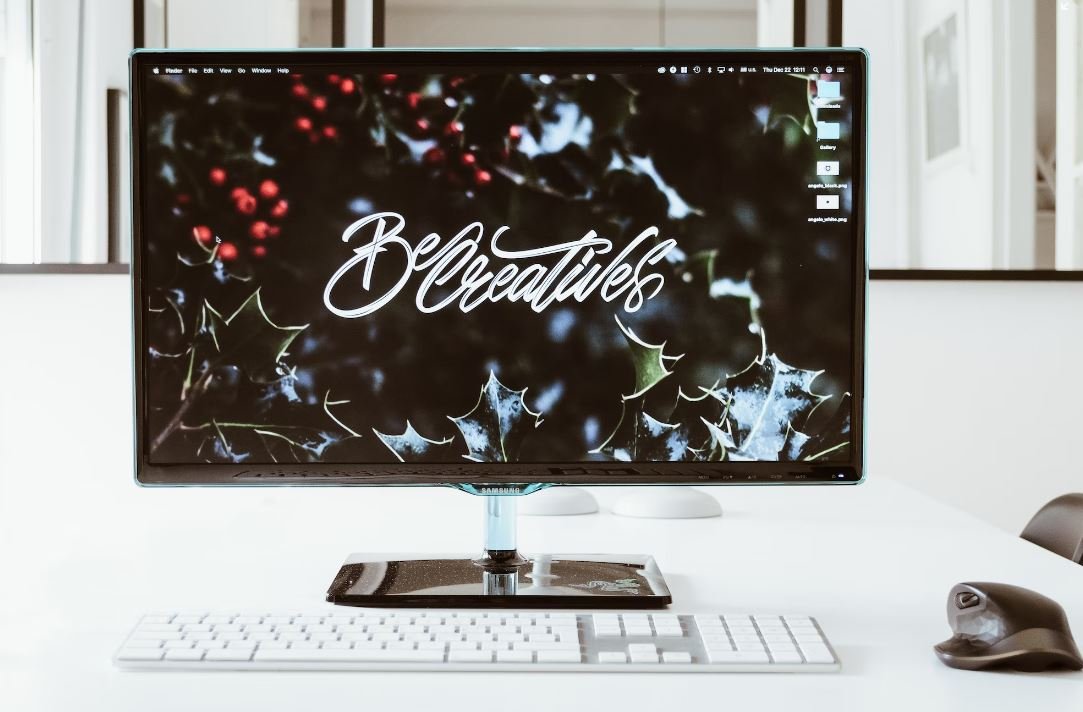
In today’s rapidly evolving world, artificial intelligence (AI) has become an integral part of numerous industries and sectors. From healthcare to finance, AI models are being employed to enhance efficiency, accuracy, and decision-making processes. This article delves into various AI models utilized throughout the project cycle and their significant impact. Ten tables are presented below, showcasing fascinating data and informative details on each topic.
1. AI Model Adoption Rates by Industry:
This table demonstrates the varying adoption rates of AI models in different industries, highlighting sectors that have embraced AI to a greater extent.
2. AI Model Performance Comparison:
Explore how different AI models perform in specific tasks or functions, presenting their accuracy or success rates.
3. Resource Allocation with AI Models:
This table outlines how AI models aid in optimized resource allocation, showcasing the tangible benefits it brings to project planning and execution.
4. Impact of AI Models on Budget:
Discover how AI models can impact the budget of a project, analyzing cost savings or additional expenses associated with their implementation.
5. Risk Mitigation with AI Models:
Explore the role AI models play in risk mitigation, evaluating the reduction of potential risks during project execution.
6. AI Models in Project Timeline Management:
This table illustrates the use of AI models to manage project timelines efficiently, demonstrating how they contribute to meeting deadlines.
7. Project Success Rate with AI Models:
Evaluate the success rates of projects utilizing AI models, highlighting the correlation between AI integration and project outcomes.
8. AI Model Impact on Team Collaboration:
Discover how AI models facilitate collaboration among team members, enhancing communication, productivity, and collective decision-making.
9. AI Models in Quality Control:
Explore the impact of AI models on maintaining and improving the quality of project deliverables, ensuring fewer errors and deviations.
10. User Satisfaction with AI Models:
This table showcases user satisfaction ratings when AI models are integrated into project processes, emphasizing their positive impact on stakeholders.
In conclusion, AI models have become indispensable in the project cycle, transforming the way projects are executed across various industries. These tables provide a glimpse into the vast potential and benefits AI models offer, from resource allocation and risk mitigation to timelines management and team collaboration. As technology continues to advance, the integration of AI models in project management will undoubtedly play a crucial role in achieving better outcomes and improving overall project success.
AI Models in Project Cycle – Frequently Asked Questions
What are AI models in project cycle?
An AI model is a mathematical representation of a real-world problem that can be used to make predictions, classify data, or generate insights. In the project cycle, AI models can be employed to automate tasks, improve efficiency, and assist in decision-making.
How are AI models developed?
AI models are developed through a process that involves data collection, preprocessing, feature engineering, algorithm selection, training, evaluation, and deployment. It requires expertise in machine learning techniques and programming skills.
What types of AI models are commonly used in projects?
Common types of AI models used in projects include regression models, classification models, clustering models, neural networks, support vector machines, decision trees, and ensemble models.
What are the benefits of using AI models in project cycle?
Using AI models in the project cycle can lead to improved efficiency, increased accuracy, faster decision-making, cost savings, and better utilization of resources. It can also uncover patterns and insights from large datasets that might not be readily apparent to humans.
What challenges may arise when implementing AI models in a project cycle?
Some challenges that may arise when implementing AI models in a project cycle include finding and preparing high-quality data, choosing the right algorithms and techniques, dealing with bias or ethical concerns, ensuring model interpretability, and managing scalability and deployment issues.
How can one evaluate the performance of an AI model?
The performance of an AI model can be evaluated using various metrics such as accuracy, precision, recall, F1-score, area under the receiver operating characteristic (ROC) curve, and mean squared error. Cross-validation techniques and comparing against baselines or benchmarks can also provide insights into the model’s performance.
What is transfer learning in AI models?
Transfer learning is a technique where a pre-trained AI model, which has been trained on a large dataset, is used as a starting point for a new task or problem. It can save time and resources by leveraging the knowledge learned from previous tasks.
Are AI models biased?
AI models can be biased if the training data used to develop them contains biased or unfair patterns. Biases may arise due to limitations in the training dataset or unintentional biases introduced by humans involved in the model development process. It is important to carefully consider and mitigate biases in AI models.
How can AI models be deployed in real-world projects?
To deploy AI models in real-world projects, they need to be integrated into existing systems or software, and appropriate infrastructure must be in place to handle the computational requirements. It is also important to continuously monitor and update the model as new data becomes available to maintain optimal performance.
What are some real-world examples of AI models in project cycle?
Real-world examples of AI models in project cycles include predicting customer churn, optimizing supply chain management, fraud detection, sentiment analysis, recommendation systems, image recognition, and natural language processing applications.
“`
Please note that this HTML code is meant to be a reference and may need to be modified or styled according to your specific needs.