AI Models for Cybersecurity
Artificial Intelligence (AI) has become a critical component in the battle against cyber threats. As the volume and complexity of cyber attacks continue to increase, traditional security measures alone are no longer sufficient. Organizations are turning to AI to enhance their cybersecurity strategies and protect against both known and unknown threats. In this article, we will explore the use of AI models in cybersecurity and the advantages they offer.
Key Takeaways:
- AI models are an essential tool in combating cyber threats.
- They provide advanced threat detection and prediction capabilities.
- AI models can handle large datasets and detect anomalies.
- They can automate cybersecurity tasks and reduce response times.
AI models in cybersecurity leverage machine learning algorithms to detect patterns and anomalies in data, enabling them to identify potential threats and vulnerabilities. These models can analyze vast amounts of data in real-time, providing organizations with a proactive approach to cybersecurity.
*By using AI models, organizations can
detect potential threats in *real-time* and take immediate action to mitigate them.”
The capabilities of AI models go beyond traditional security measures. They are designed to continuously learn and adapt from new data to improve their accuracy over time. This allows them to stay ahead of emerging threats and provide organizations with a higher level of protection.
“The adaptability of AI models ensures that organizations can effectively counter evolving threats in the ever-changing landscape of cybersecurity.”
Furthermore, AI models can automate certain tasks that were previously performed manually, such as threat hunting and incident response. This automation not only frees up valuable resources but also minimizes human error and enhances the overall efficiency of cybersecurity operations.
“Automating cybersecurity tasks using AI models can accelerate incident response, reducing potential damage.”
AI models can leverage big data analytics to identify anomalies and deviations from normal behavior. By analyzing vast amounts of data, these models can detect previously unknown threats or attacks that may have gone unnoticed by traditional security solutions. This capability is especially crucial in today’s threat landscape, where attackers constantly evolve their techniques.
“AI models excel at detecting unknown threats through anomaly detection and behavior analysis.”
Improving Cybersecurity with AI Models
Organizations can benefit from implementing AI models into their cybersecurity strategies in several ways:
- Improved Threat Detection: AI models have a higher accuracy rate in identifying known and unknown threats, ultimately enhancing network security.
- Real-time Response: AI models can detect and respond to cyber threats in real-time, minimizing the impact and potential damage.
- Reduced False Positives: By leveraging AI models, organizations can significantly reduce false positive alerts, ensuring that security teams focus on actual threats.
- Automation of Routine Tasks: AI models can simplify and automate various routine cybersecurity tasks, freeing up valuable resources for more critical operations.
- Enhanced Incident Response: By automating incident response, organizations can significantly reduce the response time, limiting the impact of cyber attacks.
Implementing AI models into cybersecurity strategies requires a comprehensive understanding of the organization’s unique security needs. It is essential to select the appropriate AI model and integrate it seamlessly into existing security systems to achieve optimal results.
A Look at the Numbers
Cybersecurity Statistics | |
---|---|
81% | Percentage of organizations that have experienced a cyber attack |
95% | Percentage of cyber attacks that exploit human error |
As the complexity and frequency of cyber attacks continue to rise, organizations must adapt their cybersecurity strategies. AI models provide a powerful tool to strengthen defenses, improve response times, and mitigate potential damages.
Integration and Implementation
Benefits of AI Models in Cybersecurity | Challenges in Implementing AI Models in Cybersecurity |
---|---|
Improved threat detection | Lack of skilled AI professionals |
Real-time response capabilities | Integration with existing security systems |
Reduced false positives | Ensuring data privacy and compliance |
While AI models offer significant advantages in cybersecurity, the integration and implementation process can pose certain challenges. Organizations must address these challenges to fully leverage the potential of AI models in their security infrastructure.
In conclusion, AI models are a crucial component of modern cybersecurity strategies. Their ability to detect, adapt, and automate cybersecurity tasks provides organizations with enhanced protection against evolving threats. By implementing the appropriate AI models and addressing the associated challenges, organizations can significantly strengthen their cybersecurity defenses and stay one step ahead of cyber attackers.
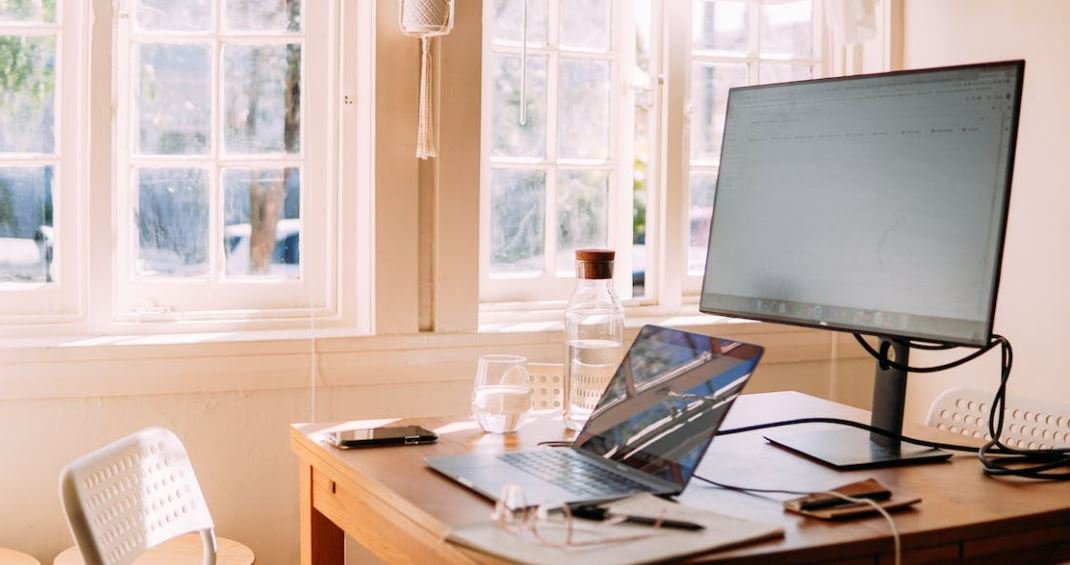
Common Misconceptions
AI Models for Cybersecurity
There are several common misconceptions surrounding the use of AI models for cybersecurity. Some people have unrealistic expectations or misunderstandings about the capabilities and limitations of these models. It is important to debunk these misconceptions to have a better understanding of how AI can be effectively utilized in the field of cybersecurity.
- A misconception is that AI models can completely eliminate all cybersecurity threats, making human involvement unnecessary. This is not true as AI models are not foolproof and can also be vulnerable to attacks. Additionally, human expertise is crucial to understand the context and make critical decisions in cybersecurity.
- AI models are often thought to replace the need for security professionals altogether. However, this is not the case as AI models can only assist in certain aspects, such as detecting patterns and anomalies, but they cannot replace the human intuition and decision-making abilities that come with experience and domain knowledge.
- Another common misconception is that AI models can accurately predict all future threats and vulnerabilities. AI models can analyze large amounts of data to identify patterns, but they rely on historical data to make predictions. As cyber threats constantly evolve, it is impossible for AI models to predict all unknown and emerging threats accurately.
It is also important to understand that AI models are not inherently biased or discriminatory. However, there is a misconception that AI models for cybersecurity can reinforce bias in threat detection and prevention. In reality, any biases in AI models are a result of the data used to train them. Proper data selection and ongoing monitoring can help mitigate biases and ensure fairness and inclusivity in cybersecurity practices.
- Assuming that AI models can be implemented instantly and seamlessly is another misconception. Implementing AI models for cybersecurity requires thorough planning, integration with existing systems, and continuous monitoring and improvement. It is a time-consuming and ongoing process that necessitates collaboration between cybersecurity experts and data scientists.
- Lastly, a common misconception is that AI models can replace the need for user awareness and education in cybersecurity. While AI models can assist in detecting and mitigating threats, user knowledge and diligence are still crucial in preventing social engineering attacks, phishing attempts, and other user-dependent vulnerabilities.
- Some people also assume that AI models can autonomously make accurate decisions without human intervention. However, in the field of cybersecurity, human expertise and decision-making are essential. AI models can provide insights and recommendations, but final decisions and actions should be made by skilled security professionals.
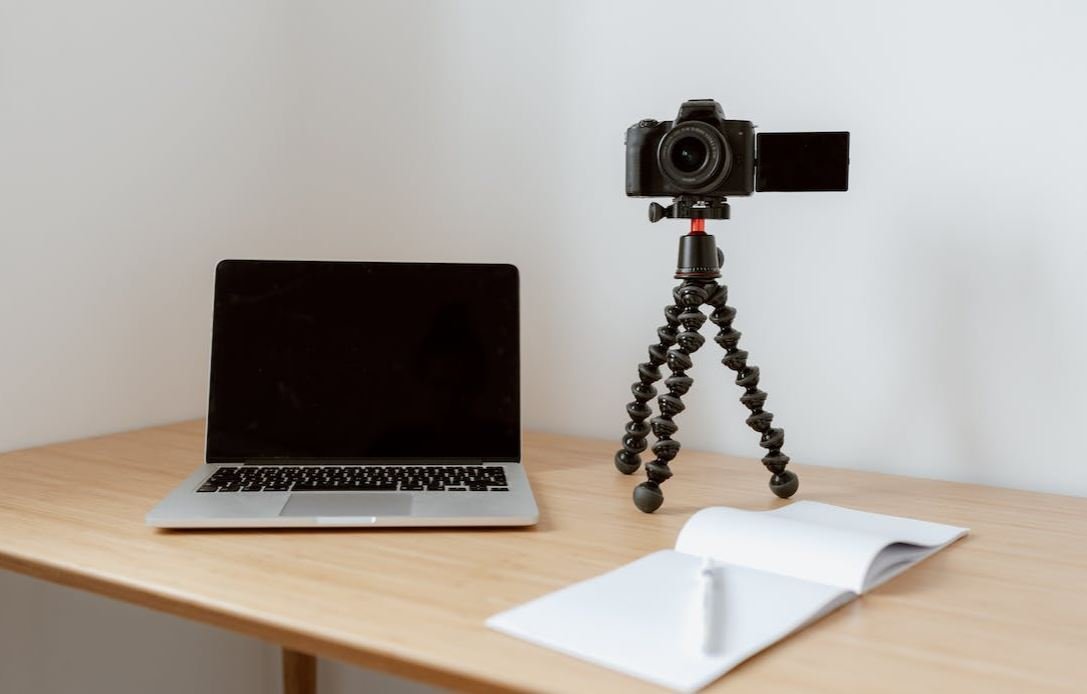
Introduction
Artificial Intelligence (AI) models have revolutionized the cybersecurity landscape, enhancing our ability to combat evolving threats and protect sensitive data. This article explores ten fascinating elements that highlight the effectiveness and potential of AI models in cybersecurity.
1. Ransomware Attacks
Ransomware attacks have increased exponentially in recent years, causing significant financial losses. AI models have proven highly effective in detecting and preventing these attacks, reducing the impact on individuals and organizations alike.
2. Phishing Detection
AI models excel at identifying and preventing fraudulent emails, significantly reducing the success rate of phishing attempts. By analyzing various factors such as email content, sender information, and user behavior, AI models can accurately classify emails as either legitimate or malicious.
3. Malware Detection
Traditional signature-based antivirus software can struggle to keep up with the constantly evolving landscape of malware. AI models utilize advanced machine learning algorithms to efficiently detect and mitigate new and sophisticated malware strains, bolstering overall cybersecurity measures.
4. Network Intrusion Detection
AI models provide exceptional capabilities in spotting anomalous network behavior that could indicate a potential cyber attack. By analyzing network traffic patterns and identifying deviations from regular activity, these models can promptly alert security teams to address potential threats.
5. Behavioral Biometrics
Utilizing AI models, behavioral biometrics can be applied to verify user authenticity through unique behavioral patterns. By analyzing factors such as typing speed, mouse movements, and swipe patterns on touchscreens, these models can improve authentication processes and detect potential unauthorized access attempts.
6. Bot Detection
In the era of automated attacks, AI models are instrumental in recognizing and blocking malicious bots. By examining user behavior, mouse movements, and other patterns, these models can differentiate between human users and automated bots, reducing the risk of unauthorized access and data theft.
7. User Anomaly Detection
AI models can identify anomalous user behavior, enabling rapid detection of compromised accounts or insider threats. By analyzing patterns such as login times, IP addresses, and usage patterns, these models can alert security teams to potential security breaches and limit the extent of damage.
8. Vulnerability Assessment
AI models excel at detecting security vulnerabilities in software and systems. By analyzing code and identifying potential weaknesses, these models contribute to the development of more secure applications and infrastructure, reducing the likelihood of successful cyber attacks.
9. Threat Intelligence Analysis
AI models can process vast amounts of threat intelligence data, extracting valuable insights and identifying patterns that human analysts may overlook. By providing actionable intelligence, these models enable security teams to proactively respond to emerging threats.
10. Incident Response Optimization
Through real-time analysis of security event data, AI models can streamline incident response efforts. By automating routine tasks, these models free up security personnel’s time, allowing them to focus on more complex and critical cybersecurity issues.
Conclusion
The potential of AI models in cybersecurity is immense. These ten elements showcase the significant impact AI has on enhancing detection, prevention, and response to cyber threats. By leveraging AI models, organizations can bolster their defenses, protect sensitive data, and stay one step ahead in the ever-evolving world of cybersecurity.
Frequently Asked Questions
What is an AI model?
An AI model is a program or algorithm designed to simulate human intelligence and make predictions or decisions based on patterns and data.
How can AI models be used in cybersecurity?
AI models can be used in cybersecurity to detect and prevent various types of cyber threats, such as malware, phishing attacks, and network intrusions.
What kind of data do AI models for cybersecurity analyze?
AI models analyze a wide range of data sources, including network traffic logs, system logs, user behavior data, and malware samples, to identify potential security risks.
Do AI models replace human analysts in cybersecurity?
No, AI models are designed to augment human analysts in cybersecurity. They help in analyzing large volumes of data and identifying patterns that humans might miss.
How accurate are AI models in detecting cyber threats?
The accuracy of AI models in detecting cyber threats varies depending on the specific model and the quality of the training data. However, advanced AI models can achieve high accuracy rates.
Can AI models adapt to new and evolving cyber threats?
Yes, AI models can adapt to new and evolving cyber threats by continuously learning from new data and updating their algorithms. This allows them to stay effective in detecting new threats.
Are AI models vulnerable to attacks by hackers?
While AI models can be vulnerable to attacks, steps can be taken to secure them. Techniques such as secure model training, robust data validation, and monitoring for adversarial attacks help mitigate these risks.
Can AI models provide real-time cybersecurity monitoring?
Yes, AI models can provide real-time cybersecurity monitoring by continuously analyzing incoming data and alerting security teams in case of any suspicious or anomalous activities.
Are there any limitations to using AI models in cybersecurity?
Like any technology, AI models have limitations. They may struggle with detecting sophisticated zero-day attacks or may produce false positives. Human oversight and validation are still crucial.
What are the challenges in implementing AI models for cybersecurity?
Implementing AI models in cybersecurity requires addressing challenges such as the need for quality training data, interpretability of model outputs, scalability, and regulatory compliance.