AI Models and Use Cases
Artificial Intelligence (AI) is revolutionizing the way businesses operate and make decisions. With AI models growing in complexity and sophistication, they can now process massive amounts of data to gain valuable insights and automate tasks. This article will explore the different types of AI models and their use cases in various industries.
Key Takeaways:
- AI models are becoming increasingly complex and powerful.
- They help businesses gain valuable insights and automate tasks.
- AI is transforming industries such as healthcare, finance, and manufacturing.
Understanding AI Models
AI models are algorithms trained on vast datasets to learn from patterns and make predictions or decisions. These models mimic human intelligence by recognizing patterns and making generalizations from the given data. *The accuracy of AI models depends on the quantity and quality of the data they are trained on.*
There are two main types of AI models:
- Supervised learning: In this type of model, algorithms are trained on labeled data, with clear instructions on what the output should be. *Supervised learning models are commonly used in spam email filtering.*
- Unsupervised learning: These models work with unlabeled data and aim to find hidden patterns without any specific instructions. *Unsupervised learning can be used for customer segmentation in marketing.*
Use Cases of AI Models
AI models have a wide range of applications across industries. Let’s explore some interesting use cases:
1. Healthcare
The healthcare industry benefits greatly from AI models. Here are a few examples:
- AI models can diagnose diseases from medical images, such as X-rays and MRI scans, with accuracy rates often surpassing human capabilities.
- They help in predicting patient outcomes and identifying treatments that provide the best results.
- AI models are also useful in drug discovery, assisting scientists in identifying potential new drugs and speeding up the process of development.
2. Finance
AI has transformed the finance industry, enabling faster and more accurate decision-making. Some use cases include:
- AI models can analyze huge volumes of financial data in real-time to detect fraudulent transactions or patterns, enhancing security measures.
- They aid in financial forecasting and investment management, helping predict market trends and manage portfolios more efficiently.
- AI models automate repetitive tasks like document verification and data entry, reducing manual errors and improving operational efficiency.
3. Manufacturing
AI models play a crucial role in optimizing manufacturing processes and improving product quality. Consider the following applications:
Use Case | Benefits |
---|---|
Quality Control | AI models can inspect products in real-time, detecting defects and anomalies with greater accuracy than human inspectors. |
Predictive Maintenance | By analyzing sensor data, AI models can predict when machines are likely to fail, allowing businesses to perform maintenance proactively. |
Supply Chain Optimization | AI models help optimize inventory management and demand forecasting, reducing costs and minimizing stockouts. |
The Future of AI Models
The potential of AI models is vast, and their capabilities continue to evolve. As technology advances, AI models will become even more intelligent and versatile. With ongoing research and development, we can expect further breakthroughs in AI applications across industries.
AI models hold the key to boosting efficiency, accuracy, and decision-making capabilities for businesses worldwide. Leveraging the power of AI, companies can gain a competitive edge and unlock new opportunities.
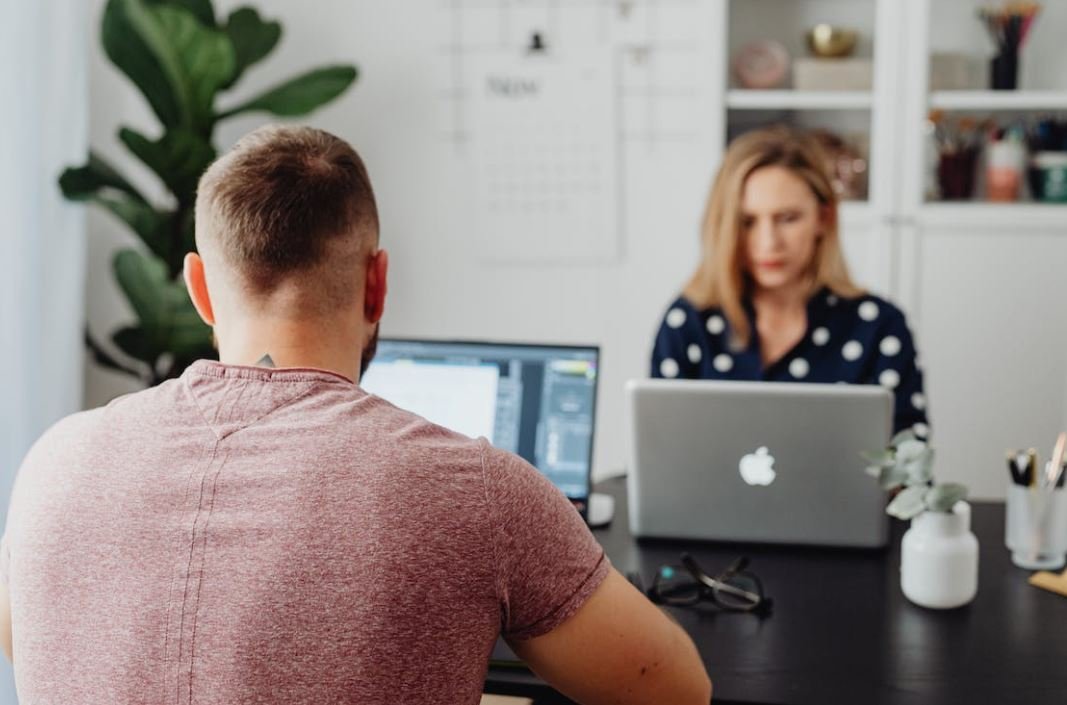
Common Misconceptions
Misconception 1: AI models can think and reason like humans
One of the most common misconceptions about AI models is that they have the ability to think and reason like humans. However, AI models are not capable of true understanding or consciousness. They are trained to perform specific tasks using pre-defined algorithms and statistical models.
- AI models rely on patterns and statistical correlations rather than true comprehension of the data.
- AI models lack the ability to generalize knowledge beyond their training data.
- AI models do not possess emotions or subjective experiences like humans.
Misconception 2: AI models are unbiased and fair
Many people believe that AI models are unbiased and fair since they are trained using large datasets. However, AI models can inherit biases from the data they are trained on or from the biases of their creators.
- AI models can perpetuate existing biases present in the data, such as gender or racial biases.
- AI models may not account for the ethical implications of their predictions or decisions.
- AI models require careful evaluation and ongoing monitoring to ensure fairness and avoid unintended consequences.
Misconception 3: AI models will replace human jobs completely
There is a common misconception that AI models will completely replace human jobs in the future. While AI models can automate certain tasks, they are more likely to augment human capabilities rather than replace them entirely.
- AI models excel at repetitive and data-driven tasks, but lack human-like creativity and adaptability.
- AI models are often built to work alongside humans, assisting in decision-making or streamlining processes.
- AI models are more likely to create new jobs and change the nature of existing roles rather than eliminate them.
Misconception 4: All AI models are the same
Another misconception is that all AI models are the same and can be used interchangeably. In reality, AI models can vary significantly in their architectures, performance, and suitability for specific use cases.
- AI models are designed to solve specific problems and may not perform well or generalize to other tasks.
- AI models can be classified into different types, such as machine learning, deep learning, or natural language processing models.
- AI models require customization and fine-tuning to address specific business needs and achieve desired outcomes.
Misconception 5: AI models are fully autonomous
While AI models can perform complex tasks autonomously, they still require human oversight and intervention. AI models are not completely independent decision-makers and should be monitored and controlled by humans.
- AI models may make errors or produce unexpected outputs that need to be corrected by human intervention.
- AI models can be susceptible to adversarial attacks, where deliberate manipulation of inputs can cause incorrect or harmful outputs.
- AI models need continual monitoring to ensure they are aligned with ethical, legal, and regulatory standards.
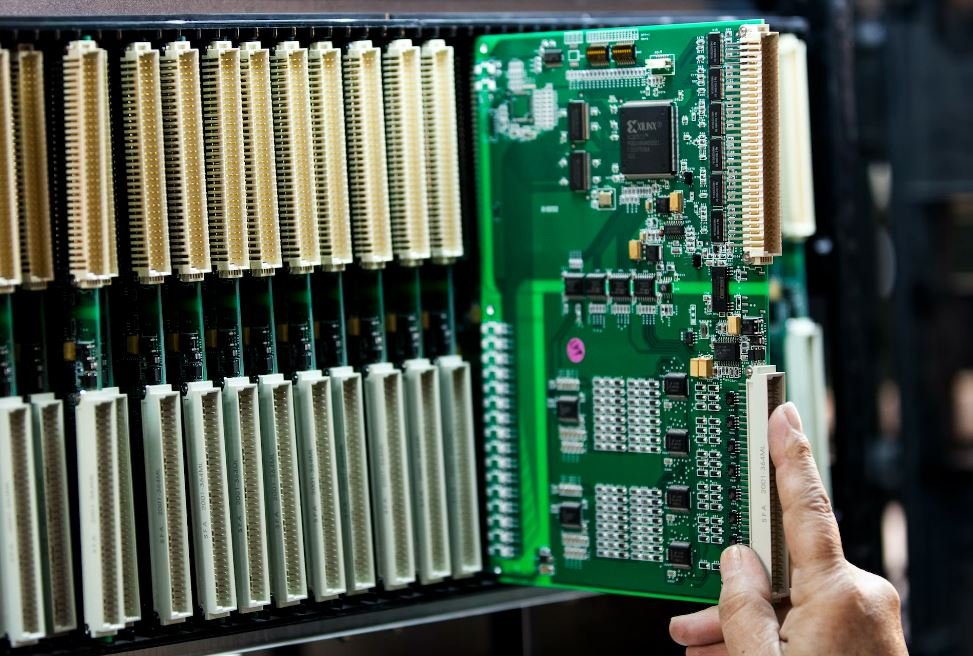
AI Models and Use Cases
Artificial Intelligence (AI) has revolutionized many industries, impacting everything from customer service to healthcare. AI models have the ability to process vast amounts of data and make decisions or predictions based on patterns and algorithms. In this article, we explore various use cases of AI and the remarkable results it has achieved.
Enhancing Customer Service
AI-powered virtual assistants have opened new possibilities for customer service. By analyzing customer data and behavior patterns, these virtual assistants provide personalized recommendations and offer immediate assistance, greatly enhancing the overall customer experience.
Improving Healthcare Diagnosis
AI models trained on millions of medical records have shown incredible accuracy in diagnosing diseases. By analyzing symptoms and medical data, these models can predict illnesses with higher accuracy rates than human doctors, potentially saving lives and improving healthcare outcomes.
Streamlining Supply Chain Management
AI algorithms can optimize supply chain operations, predicting demand fluctuations and enabling proactive inventory management. This results in improved efficiency, reduced costs, and better customer satisfaction due to shorter delivery times and increased availability of products.
Preventing Fraud and Cyber Attacks
AI models can identify suspicious activities in real-time, significantly improving fraud detection and prevention. By continuously analyzing data patterns, these models can detect fraudulent transactions or abnormal behaviors, providing early warning signs and protecting businesses and individuals from cyber threats.
Automating Financial Analysis
AI models excel at analyzing financial data and making predictions. They can automate financial analysis tasks, such as risk assessment, investment portfolio optimization, and market trend predictions. By leveraging AI in these areas, organizations can make more informed and profitable decisions.
Optimizing Energy Consumption
AI models can analyze energy consumption patterns and optimize energy usage in various industries. They can detect inefficiencies, recommend changes to reduce energy waste, and predict peak demand, allowing for more efficient allocation of resources and reducing environmental impact.
Personalizing Advertising Campaigns
With AI, advertisers can tailor their campaigns to specific audiences by analyzing user preferences, behaviors, and demographic data. This increases the likelihood of reaching the right customers with personalized messages, leading to higher engagement and conversion rates.
Enhancing Autonomous Vehicles
AI technology is crucial for the development of autonomous vehicles. AI models process sensor data and make split-second decisions to navigate and respond to changing road conditions. By continuously learning from their surroundings, autonomous vehicles become safer and more efficient.
Improving Agricultural Yield
With AI models, farmers can optimize crop yield and prevent disease outbreaks. These models analyze variables such as weather data, soil conditions, and pest patterns to provide real-time insights and recommendations. This leads to increased productivity and more sustainable farming practices.
Assisting in Natural Language Processing
AI models have made significant advancements in natural language processing, enabling machines to understand and respond to human language. This has resulted in improved voice assistants, chatbots, and language translation tools, making communication between humans and machines more seamless.
In conclusion, AI models have emerged as powerful tools across a range of industries, transforming processes, optimizing efficiency, and improving decision-making capabilities. By harnessing the potential of AI, organizations can unlock new possibilities and achieve unprecedented results.
Frequently Asked Questions
What are AI models?
An AI model is a mathematical representation or algorithm that enables computers to learn from and make predictions or decisions without being explicitly programmed. It analyzes large amounts of data to find patterns, make predictions, or perform specific tasks.
How are AI models trained?
AI models are trained using machine learning techniques. This involves providing the model with a large dataset and allowing it to learn from the patterns and relationships found in the data. The model goes through an iterative process, adjusting its algorithm and parameters until it can produce accurate predictions or decisions.
What are some popular AI model architectures?
Some popular AI model architectures include convolutional neural networks (CNNs) for image recognition, recurrent neural networks (RNNs) for sequence and time-series data, and transformer models for natural language processing (NLP) tasks. Each architecture is designed to excel in specific domains.
What are the practical use cases of AI models?
AI models have a wide range of practical use cases across various industries. They can be used for image and object recognition, speech and natural language processing, recommendation systems, fraud detection, autonomous vehicles, drug discovery, and much more. The possibilities are vast and constantly expanding.
How accurate are AI models?
The accuracy of AI models varies depending on the complexity of the task and the amount and quality of the training data. In many cases, AI models can achieve high levels of accuracy, surpassing human performance in specific domains. However, it’s important to continually evaluate and fine-tune models to maintain their accuracy and address any biases they might have.
What challenges do AI models face?
AI models face several challenges, including the need for large amounts of labeled data for training, potential biases in the data that can affect the model’s decisions, interpretability and explainability of the model’s decisions, and ethical considerations such as privacy and fairness.
How can AI models be evaluated?
AI models can be evaluated using various metrics depending on the task they are designed to perform. Common evaluation metrics include accuracy, precision, recall, F1 score, and area under the curve (AUC). It’s also important to consider the model’s performance on different subsets of data to ensure its generalizability.
How can AI models be deployed in real-world applications?
To deploy AI models in real-world applications, they need to be integrated into the existing software infrastructure. This involves incorporating the models into an application or system, ensuring scalability and efficiency, handling data input and output, and continuously monitoring and updating the models to maintain their performance.
What are the ethical considerations of AI models?
Ethical considerations of AI models include issues such as privacy, transparency, fairness, and accountability. AI models should be designed to respect user privacy and protect sensitive data. They should also be transparent in their decision-making process, providing explanations for their outputs. Fairness is crucial to ensure that AI models do not reinforce biases or discriminate against certain groups. Finally, developers and organizations should be accountable for the actions and consequences of AI models.
How can AI models be improved?
AI models can be improved through continuous monitoring, evaluation, and update cycles. This involves gathering feedback from users, evaluating the model’s performance, identifying areas of improvement, and retraining or fine-tuning the model to address any limitations or biases. Collaborative efforts and ethical considerations can also contribute to the improvement of AI models.