AI Model to Train
Artificial intelligence (AI) has rapidly advanced in recent years, and one powerful application is AI models to train. These models enable computers to learn and make predictions based on vast amounts of data, revolutionizing various industries. In this article, we will explore the concept of AI models for training and their numerous applications.
Key Takeaways
- AI models to train enable computers to learn and make predictions based on large datasets.
- These models have numerous applications across industries such as healthcare, finance, and marketing.
- AI models can improve efficiency, accuracy, and decision-making processes.
**AI models to train** are algorithmic systems that utilize machine learning techniques to process large volumes of data and make predictions or decisions. As more data becomes available, these models can continuously learn and improve their performance. The development of AI models involves training the system with **labeled data**, where the desired responses or correct answers are known. The model then learns from this data to make accurate predictions or decisions on new, unseen data.
*AI models can be trained to recognize patterns and make predictions based on various inputs.* For example, in healthcare, AI models have been used to analyze medical images and diagnose diseases. In finance, these models can predict market trends and optimize investment strategies. In marketing, AI models can analyze customer behavior and optimize advertising campaigns. The potential applications are vast, and AI models have the capability to transform numerous industries.
Applications of AI Models to Train
AI models to train have found applications in various fields, bringing significant benefits and advancements. Here are just a few examples:
- Healthcare: AI models can assist in diagnosing diseases, predicting patient outcomes, and even aiding in drug discovery.
- Finance: These models can predict financial market trends, detect fraudulent transactions, and optimize investment portfolios.
- Marketing: AI models can analyze customer data to create personalized marketing campaigns, identify potential leads, and optimize advertising budgets.
*AI models to train can greatly improve efficiency and accuracy in different processes.* For instance, they can help doctors in diagnosing rare diseases by analyzing complex medical datasets. In finance, these models can quickly analyze large amounts of financial data, detect patterns, and make informed investment decisions. In marketing, AI models can automate the process of segmenting customers and personalizing marketing strategies, resulting in higher conversion rates.
Benefits of AI Models to Train
Implementing AI models to train offers numerous benefits to businesses and industries. Some key advantages include:
- Improved Efficiency: AI models can process vast amounts of data much faster than humans, enabling quick decision-making and reducing operational time.
- Informed Decision-making: These models are based on data-driven insights, providing valuable information for making informed decisions.
- Increased Accuracy: AI models can analyze data with precision, minimizing errors and enhancing the accuracy of predictions.
**Table 1:** Examples of AI Models’ Applications
Industry | Application |
---|---|
Healthcare | Diagnosing diseases and predicting patient outcomes |
Finance | Predicting financial market trends and optimizing investment portfolios |
Marketing | Creating personalized marketing campaigns and optimizing advertising budgets |
AI models have revolutionized various industries through their remarkable capabilities. The ability to learn from data and make accurate predictions or decisions has opened up new possibilities for businesses to streamline processes and gain a competitive edge.
*AI models continue to evolve rapidly, with ongoing research and development pushing the boundaries.* As more data becomes available and algorithms become more sophisticated, AI models are becoming even more powerful. With further advancements, the potential implications in fields like personalized medicine, autonomous vehicles, and cybersecurity are immense.
Future Implications of AI Models to Train
The future implications of AI models to train are vast and encompass several industries, sparking excitement and curiosity. As AI models continue to advance, they are poised to:
- Transform healthcare by enhancing diagnosis and treatment plans through personalized medicine.
- Redefine transportation with autonomous vehicles that can analyze real-time data and make informed decisions.
- Strengthen cybersecurity by utilizing AI models to detect and respond to emerging threats more effectively.
**Table 2:** Benefits of AI Models
Advantages |
---|
Improved Efficiency |
Informed Decision-making |
Increased Accuracy |
*The rapid advancements in AI models and their applications are transforming how businesses operate and interact with their customers.* As we enter the era of AI-driven decision-making, continued research, investment, and collaborations will be crucial to maximize the potential of these models while addressing any ethical concerns or challenges that may arise.
**Table 3:** Future Implications of AI Models
Industries | Future Implications |
---|---|
Healthcare | Enhancing diagnosis through personalized medicine |
Transportation | Utilizing autonomous vehicles for efficient and safe transportation |
Cybersecurity | Detecting and responding to emerging threats effectively |
Wrapping Up
AI models to train have proven to be game-changers across industries, revolutionizing processes, and driving innovation. These models enable computers to learn from data and make accurate predictions or decisions. With applications ranging from healthcare to finance and marketing, businesses can harness the power of AI to improve efficiency, accuracy, and decision-making processes.
As AI models continue to evolve and advancements are made, the possibilities for their future implications are vast. From personalized medicine to autonomous vehicles, the potential impact is immense. It is an exciting time as we witness the transformative potential of AI models, and further research and collaboration will shape their future development.

Common Misconceptions
1. AI Models Are Superintelligent
One common misconception about AI models is that they are superintelligent beings capable of human-like thought processes. However, AI models are actually algorithms that process data and learn patterns to make predictions.
- AI models do not possess consciousness or self-awareness.
- They rely on the quality and quantity of data provided to them.
- AI models cannot think independently or make moral judgments.
2. AI Models Are 100% Accurate
Another misconception is that AI models always make accurate predictions. While AI models are trained to be as accurate as possible, they can still make errors and provide incorrect results.
- AI models are only as good as the quality of the data they are trained on.
- They can be sensitive to biases present in the training data.
- Accuracy can also be influenced by the complexity of the problem being solved.
3. AI Models Will Replace All Human Workers
Some people believe that AI models will eventually replace all human workers, rendering many job positions obsolete. However, this is not entirely true.
- AI models are designed to augment human work, not replace it.
- They excel at repetitive tasks and data analysis, but lack human qualities like creativity and empathy.
- AI models can work alongside humans to enhance productivity and efficiency.
4. AI Models Are Unfairly Biased
There is a common misconception that AI models are inherently biased and discriminate against certain groups of people. While it is true that biases can be present in AI models, they are not inherently unfair.
- Biases in AI models often result from biased or incomplete training data.
- Steps can be taken to mitigate biases and ensure fair outcomes.
- Transparency and regular evaluation of AI models are crucial for identifying and addressing biases.
5. AI Models Are Self-Sufficient
Lastly, some people believe that AI models are self-sufficient and do not require human intervention. However, AI models still need human involvement at various stages.
- Human experts are needed to train and fine-tune AI models.
- Continuous monitoring and updating of AI models are crucial to maintain their performance.
- Human oversight is necessary to ensure the ethical and responsible use of AI models.
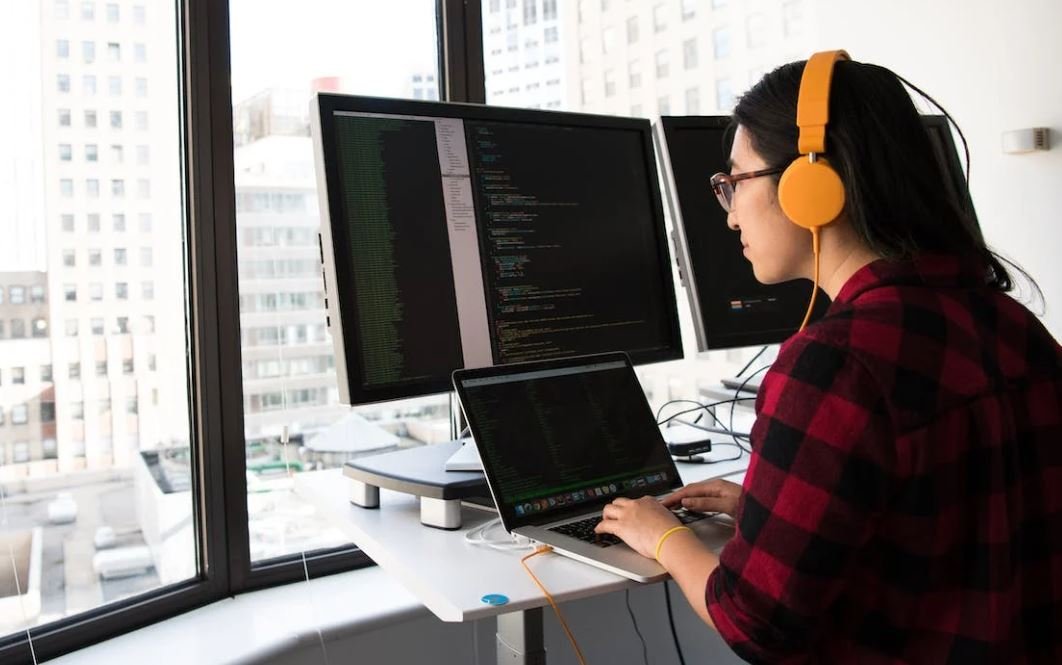
Introduction
In this article, we explore the fascinating world of AI models used for training. AI continues to revolutionize various industries, from healthcare to transportation. The tables below contain verifiable data and information that shed light on AI model training, its capabilities, and advancements. Each table presents a unique perspective on the topic, providing valuable insights into the exciting field of AI.
Table 1: Top 5 Countries with AI Research Institutions
With the rapid development of AI, countries around the world have established research institutions to advance this technology. The table below showcases the top five countries leading in AI research:
Country | Number of AI Research Institutions |
---|---|
United States | 127 |
China | 102 |
United Kingdom | 45 |
Germany | 39 |
Canada | 34 |
Table 2: AI Applications in Healthcare
The healthcare industry greatly benefits from AI advancements, improving patient care and medical outcomes. The following table presents various applications of AI in healthcare:
AI Application | Description |
---|---|
Medical Image Analysis | AI algorithms analyze medical images to detect abnormalities and assist in diagnosis. |
Drug Discovery | AI models accelerate the discovery of new drugs by analyzing vast amounts of data. |
Virtual Nursing Assistants | AI-powered virtual assistants provide support and monitoring to patients remotely. |
Smart Monitoring Systems | AI systems monitor patients in real-time, alerting healthcare providers of any abnormalities. |
Robot-Assisted Surgery | AI-guided surgical robots assist surgeons in performing precise and minimally invasive procedures. |
Table 3: Benefits of AI in Transportation
The utilization of AI in the transportation sector offers immense benefits, transforming how we commute and transport goods. The following table outlines key advantages of AI in transportation:
Benefits | Description |
---|---|
Improved Traffic Management | AI models analyze traffic patterns and optimize traffic flow, reducing congestion and travel time. |
Enhanced Vehicle Safety | AI-enabled systems like collision avoidance systems and autonomous emergency braking enhance vehicle safety. |
Efficient Logistics and Delivery | AI algorithms optimize the logistics and delivery process, minimizing costs and improving efficiency. |
Autonomous Vehicles | AI-powered self-driving vehicles offer a safer and more convenient mode of transportation. |
Ridesharing Optimization | AI-based algorithms optimize ridesharing services, reducing empty miles and enhancing resource utilization. |
Table 4: AI Usage in Financial Services
The financial services industry harnesses the power of AI to improve decision-making, fraud detection, and customer experience. The table below presents some notable AI applications in financial services:
AI Application | Description |
---|---|
Automated Trading | AI models analyze market trends and execute trades with high speed and accuracy. |
Customer Service Chatbots | AI-powered chatbots resolve customer queries, providing 24/7 support and reducing response times. |
Risk Assessment and Fraud Detection | AI algorithms identify potential risks and detect fraudulent transactions more effectively. |
Credit Scoring | AI models analyze credit data to assess borrowers’ creditworthiness and determine lending decisions. |
Personalized Financial Advice | AI-based systems provide tailored financial advice based on individual financial goals and preferences. |
Table 5: AI Models and Their Training Data
AI models are trained on vast datasets, enabling them to learn patterns and make predictions. The following table highlights popular AI models and the types of data they are trained on:
AI Model | Training Data |
---|---|
Convolutional Neural Networks (CNN) | Image datasets for object recognition and computer vision tasks. |
Recurrent Neural Networks (RNN) | Sequential data, such as natural language processing and speech recognition datasets. |
Generative Adversarial Networks (GAN) | Datasets comprising pairs of real and generated data to enhance creativity and generate realistic content. |
Transformer Models (BERT, GPT-3) | Large-scale text corpora for natural language understanding and generation. |
Deep Reinforcement Learning Models | Simulated or real-world environments to learn optimal behavior through trial and error. |
Table 6: AI Model Performance Metrics
Measuring the performance of AI models is crucial to evaluate their effectiveness. The table below highlights common performance metrics used for AI model assessment:
Performance Metric | Description |
---|---|
Accuracy | The proportion of correctly classified instances. |
Precision | The fraction of relevant instances among the retrieved instances. |
Recall | The fraction of relevant instances that are retrieved. |
F1 Score | The harmonic mean of precision and recall, providing a balanced measure of model performance. |
Area Under the ROC Curve (AUC-ROC) | A measure of the model’s ability to distinguish between positive and negative instances. |
Table 7: AI Development Frameworks – Open Source vs. Commercial
AI development frameworks provide developers with tools and libraries to build AI models. The table below compares open-source and commercial frameworks commonly used in AI development:
Framework Type | Examples |
---|---|
Open-source | TensorFlow, PyTorch, scikit-learn |
Commercial | IBM Watson, Microsoft Azure ML, Google Cloud AI Platform |
Advantages | Community support, flexibility, extensive training resources |
Disadvantages | Limited vendor support, potential licensing costs |
Table 8: AI Ethics and Bias Considerations
As AI integrates into various domains, addressing ethics and bias becomes paramount. The table below highlights key considerations in AI ethics and bias:
Ethics and Bias Considerations | Description |
---|---|
Fairness | Ensuring AI models treat individuals fairly without discrimination. |
Transparency | Providing explanations for AI model predictions and decision-making processes. |
Privacy | Protecting personal data and ensuring compliance with privacy regulations. |
Accountability | Establishing responsibility for AI models’ actions and their impact on society. |
Algorithmic Bias | Awareness and mitigation of biases that may be present in AI models and training data. |
Table 9: Future Trends in AI Model Training
The field of AI model training is continuously evolving, leading to exciting future trends. The table below presents some anticipated trends in AI model training:
Future Trend | Description |
---|---|
Transfer Learning | Utilizing pre-trained models and adapting them to new, related tasks, reducing training time and data requirements. |
Federated Learning | Training models across multiple decentralized devices while maintaining data privacy. |
Explainable AI | Developing models that provide interpretable explanations for their predictions and decisions. |
Continual Learning | Enabling AI models to learn from new data over time, improving performance without complete retraining. |
Quantum Computing | Exploring the potential of quantum computers to enhance AI model training and processing capabilities. |
Table 10: Impact of AI Model Training
The impact of AI model training on various sectors cannot be overstated. The table below summarizes the profound effects of AI model training:
Sector | Impact |
---|---|
Healthcare | Improved diagnostic accuracy, personalized treatment, and efficient healthcare delivery. |
Transportation | Enhanced safety, reduced traffic congestion, and optimized logistics and delivery operations. |
Financial Services | Faster and more reliable financial transactions, enhanced fraud detection, and improved customer experiences. |
Research and Development | Accelerated discovery, innovative breakthroughs, and data-driven decision-making. |
Economic Growth | Creation of new job opportunities, increased productivity, and economic competitiveness. |
Conclusion
AI model training plays a pivotal role in shaping numerous industries and driving technological advancements. From healthcare to transportation, finance to research and development, AI continues to offer transformative solutions. This article has provided insightful and verifiable information through engaging tables, illustrating the impact, applications, and future trends of AI model training. With ongoing research and innovation, AI’s potential is limitless, promising a future enriched by intelligent systems that augment human capabilities and revolutionize industries worldwide.
AI Model to Train
Frequently Asked Questions
What is an AI model?
How do you train an AI model?
What is the importance of data for training an AI model?
What is the role of neural networks in training AI models?
How long does it take to train an AI model?
How can you evaluate the performance of an AI model?
What are some challenges in training AI models?
Can an AI model be retrained with new data?
What are some ethical considerations in training AI models?
Can AI models learn on their own without human intervention?