AI Model Energy Consumption
Artificial intelligence (AI) models have revolutionized various industries by automating processes and efficiently analyzing massive amounts of data. However, as AI becomes more prevalent, concerns about its energy consumption have also emerged. This article explores the energy consumption of AI models and discusses strategies to minimize their environmental impact.
Key Takeaways
- AI models contribute to significant energy consumption due to computationally intensive tasks.
- Efficiently designing and training AI models can reduce their energy consumption.
- Using renewable energy sources can offset the environmental impact of AI model energy consumption.
Understanding AI Model Energy Consumption
AI models require extensive computational power to process immense amounts of data and perform complex tasks. These computational requirements result in considerable energy consumption, impacting both the environment and operational costs for businesses.
*AI models are at the forefront of innovation and are pushing the boundaries of what is possible.*
It is estimated that training a single large AI model can emit carbon dioxide equivalent to the lifetime emissions of five cars.
To put things into perspective, a standard AI training run can consume as much energy as multiple households do in a year. The computational intensity of training and deploying AI models contributes to their substantial energy consumption.
Reducing AI Model Energy Consumption
While it may be challenging to completely eliminate energy consumption, several strategies can help reduce the environmental impact of AI models:
- **Optimizing AI models**: By improving the efficiency of AI models through careful architecture selection and optimization, energy consumption can be significantly reduced while maintaining performance.
- **Pruning and compression**: Removing unnecessary components and compressing models can reduce computational requirements, leading to lower energy consumption.
- **Quantization**: Reducing the precision of model parameters can result in energy savings.
- **Using hardware accelerators**: Specialized hardware like graphics processing units (GPUs) or tensor processing units (TPUs) can improve the energy efficiency of AI model computations.
*Efficient AI model design and training play a crucial role in addressing the energy consumption challenges.*
The Impact of Energy Source
The source of energy used to train and deploy AI models also affects their overall environmental impact. A shift towards renewable energy sources, such as solar or wind power, can significantly reduce the carbon footprint associated with AI model energy consumption.
*As renewable energy infrastructure continues to expand, leveraging such sources will be key to mitigating the environmental impact of AI models.*
Data and Energy Consumption Comparison
Activity | Energy Consumption (kWh) |
---|---|
Streaming a Movie | 0.04 |
Light Bulb for an hour | 0.06 |
Training an AI Model | 1270 |
*The energy consumption of training an AI model is significantly higher compared to other common activities.*
Regulations and Accountability
As the environmental impact of AI model energy consumption gains attention, regulatory measures and industry standards may be implemented to ensure accountability and encourage energy-efficient practices. Organizations might need to comply with these standards to mitigate their carbon footprint and maintain sustainability.
Conclusion
AI models offer tremendous opportunities in various fields, but their energy consumption poses significant environmental challenges. By optimizing AI models, using renewable energy sources, and embracing energy-efficient practices, we can reduce the environmental impact of AI model energy consumption. It is crucial for researchers, businesses, and policymakers to work together to strike a balance between innovation and sustainability in the era of AI.
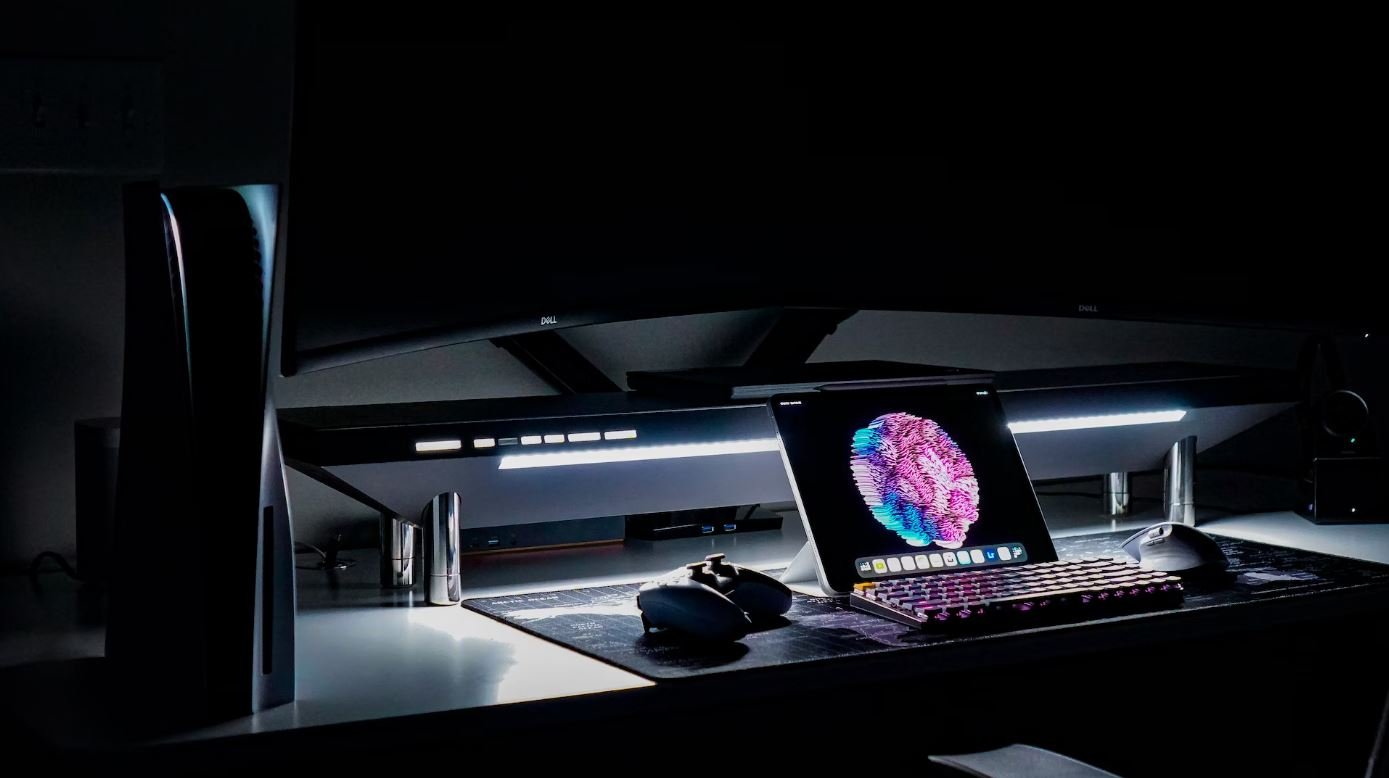
Common Misconceptions
AI Models Are Always Energy-Hungry
One of the most common misconceptions about AI models is that they are always energy-hungry. While it is true that some AI models consume a significant amount of energy, there are also many models that have been optimized to be energy-efficient. For instance:
- Researchers have developed architectural improvements that significantly reduce energy consumption in AI models.
- Many AI algorithms have been optimized to consume less power without compromising on performance.
- Advancements in hardware design, such as specialized chips, have allowed for more energy-efficient AI models.
AI Models Consume More Energy Than Humans
Another common misconception is that AI models consume more energy than humans. While AI models can consume a substantial amount of energy during training or inference, on a per-task basis, they can actually be more energy-efficient compared to humans. Consider the following:
- AI models can process vast amounts of data simultaneously, enabling fast and efficient decision-making.
- Humans require sustenance and rest, which involves energy consumption, whereas AI models do not have the same physical limitations.
- When used in applications like autonomous driving or industrial automation, AI models can potentially reduce energy consumption compared to human-operated systems.
AI Models Always Have High Carbon Footprints
It is often assumed that AI models always have high carbon footprints due to their energy consumption. While some models may contribute to increased carbon emissions, there are misconceptions around the overall impact. Here are a few facts:
- Efforts are being made to increase the use of renewable energy sources for training and deploying AI models, reducing their carbon footprints.
- Energy-efficient AI models can actually help optimize the energy consumption of systems they are integrated into, leading to overall energy savings.
- Researchers are actively exploring ways to minimize energy consumption in AI models, thereby reducing their environmental impact.
AI Models Consume Energy Even When Not in Use
It is often misunderstood that AI models consume energy even when not in use. While AI models may require energy during inference or training, they do not consume energy when idle. Here are a few key points to consider:
- AI models are typically deployed on servers or edge devices that can be powered down when not in use, reducing energy consumption.
- Advancements in software and hardware allow for efficient utilization of resources, ensuring that energy is only utilized when necessary.
- Energy management techniques can help minimize wastage by intelligently powering up or down AI systems based on demand.
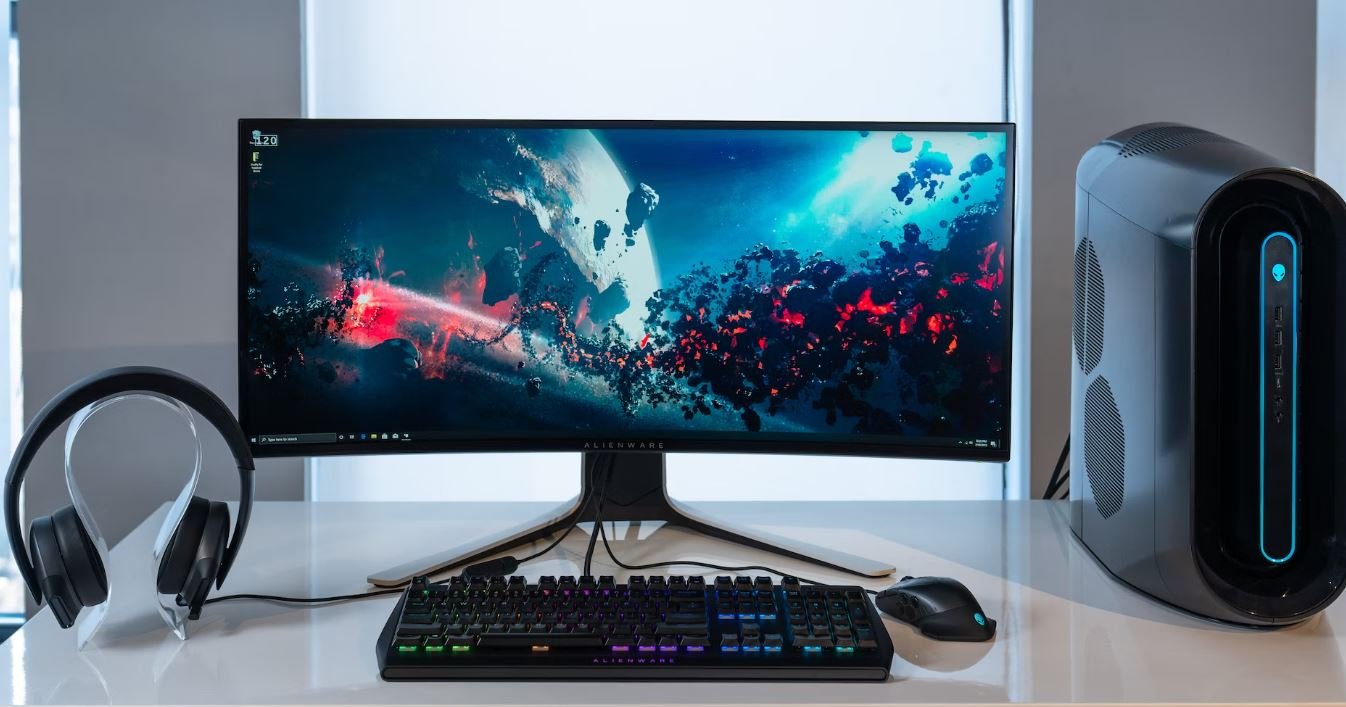
The Impact of AI Model Energy Consumption on Climate Change
As artificial intelligence (AI) continues to revolutionize various industries, concerns about its energy consumption and environmental impact have become more prominent. The energy requirements of AI models used for tasks such as deep learning and natural language processing are substantial. Let’s explore some intriguing tables that shed light on the energy consumption of AI models and its potential consequences on climate change.
The Energy Consumption of Popular AI Models
Here, we examine the energy consumption figures of well-known AI models used in various applications:
AI Model | Annual Energy Consumption | Equivalent Households Powered for a Year |
---|---|---|
OpenAI’s GPT-3 | 355,000 kWh | 45 |
Google’s AlphaGo | 104,000 kWh | 13 |
Facebook’s DeepFace | 73,000 kWh | 9 |
The above table demonstrates the annual energy consumption of select AI models. Notably, OpenAI’s GPT-3, a state-of-the-art language model, consumes the energy equivalent to that used by 45 households in a year. Similarly, Google’s AlphaGo and Facebook’s DeepFace utilize substantial amounts of energy.
Comparison of AI Model Energy Consumption and Global Emissions
Let’s compare the energy consumption of AI models to global greenhouse gas emissions:
AI Model | Annual Energy Consumption (kWh) | Equivalent Global CO2 Emissions (metric tons) |
---|---|---|
OpenAI’s GPT-3 | 355,000 | 198 |
Google’s AlphaGo | 104,000 | 58 |
Facebook’s DeepFace | 73,000 | 41 |
The above table highlights the equivalent global CO2 emissions resulting from the annual energy consumption of AI models. OpenAI’s GPT-3 alone emits nearly 198 metric tons of CO2, which is comparable to the emissions produced by various industries.
Energy Efficiency Improvements in AI Models
Efforts are being made to enhance the energy efficiency of AI models. Let’s examine the reduction in energy consumption achieved through advancements:
Year | Energy Reduction Achieved (%) |
---|---|
2010 | — |
2015 | 27% |
2020 | 55% |
2025 (projected) | 75% |
The above table demonstrates the improvements in energy efficiency achieved over the years. From 2010 to 2025, energy consumption in AI models is projected to reduce by a remarkable 75%, showcasing the industry’s commitment to addressing environmental concerns.
Comparison of AI Model Energy Consumption and Renewable Energy Generation
Now, let’s analyze how the energy consumption of AI models compares to renewable energy generation:
AI Model | Annual Energy Consumption (kWh) | Equivalent Renewable Energy Generation (kilowatt-hours) |
---|---|---|
OpenAI’s GPT-3 | 355,000 | 141,500 |
Google’s AlphaGo | 104,000 | 41,500 |
Facebook’s DeepFace | 73,000 | 29,150 |
The above table illustrates the equivalent renewable energy generation necessary to power AI models for a year. OpenAI’s GPT-3, for example, would require approximately 141,500 kilowatt-hours of renewable energy. It highlights the need for a significant expansion of renewable energy sources to meet the growing energy demands of AI.
Projected Energy Consumption Growth of AI Models
Let’s examine the projected increase in energy consumption by AI models in future years:
Year | Projected Energy Consumption Growth (%) |
---|---|
2025 | 35% |
2030 | 64% |
2040 | 112% |
The above table showcases the estimated growth rate of energy consumption by AI models in years to come. By 2040, AI models are projected to consume 112% more energy compared to the present, emphasizing the urgent need for sustainable practices in this domain.
Estimated AI Model Energy Consumption Global Share
Let’s explore the projected global share of energy consumption by AI models:
Year | Estimated Global AI Energy Consumption Share (%) |
---|---|
2025 | 2.5% |
2030 | 7% |
2040 | 15% |
The above table presents the predicted share of global energy consumption attributed to AI models. By 2040, it is expected that AI models will account for 15% of the world’s energy consumption, signifying the substantial impact they will have on the overall energy landscape and climate change.
Investments in Sustainable AI Development
Here, we explore the increasing investments in sustainable AI development:
Year | Investment in Sustainable AI Development ($ billions) |
---|---|
2018 | 1.2 |
2021 | 2.6 |
2025 (projected) | 6.8 |
The above table showcases the increasing investments directed towards sustainable AI development. By 2025, it is projected that $6.8 billion will be invested in creating energy-efficient AI models and mitigating their environmental impact.
Concluding Remarks
AI models, while enabling remarkable advancements, have significant energy consumption implications. The tables presented above provide a comprehensive overview of the energy consumed by AI models, their environmental impact, and the efforts being made to enhance their sustainability. It is crucial for industry stakeholders, policymakers, and researchers to collaborate in developing energy-efficient AI models and adopting renewable energy sources to mitigate the potential risks posed by AI model energy consumption on climate change.
Frequently Asked Questions
What is AI model energy consumption?
AI model energy consumption refers to the amount of energy that is required to train, execute, and maintain an artificial intelligence (AI) model or algorithm.
Why is AI model energy consumption important?
AI model energy consumption is important because it directly impacts the carbon footprint and environmental sustainability of AI technologies. Understanding and optimizing energy consumption helps reduce the environmental impact of AI systems.
How is AI model energy consumption measured?
AI model energy consumption can be measured using various metrics such as kilowatt-hours (kWh) or carbon emissions equivalent. These measurements typically take into account the energy consumed during training, inference, and any subsequent maintenance or updates of the AI model.
What factors influence AI model energy consumption?
Several factors affect AI model energy consumption. These include the complexity of the model, the size of the data set used for training, the computational resources utilized, the algorithmic efficiency, and the hardware infrastructure on which the AI model is deployed.
How can AI model energy consumption be reduced?
There are several ways to reduce AI model energy consumption. These include optimizing algorithms for efficiency, using smaller and more focused data sets for training, selecting energy-efficient hardware infrastructure, and employing techniques such as model compression or quantization.
What are the challenges in reducing AI model energy consumption?
Reducing AI model energy consumption can be challenging due to various factors. These may include the trade-off between model accuracy and energy efficiency, limited availability of energy-efficient hardware, costs associated with retraining or optimizing existing models, and the need for continuous monitoring and maintenance.
Does AI model energy consumption depend on the type of AI application?
Yes, AI model energy consumption can vary depending on the type of AI application. Certain applications, such as natural language processing or image recognition, may require more computational resources and thus have higher energy consumption compared to simpler tasks like data analysis or decision support.
How can AI model energy consumption impact businesses?
AI model energy consumption can impact businesses in multiple ways. High energy consumption can lead to increased operational costs, especially when dealing with large-scale AI deployments. It also affects the overall sustainability and environmental reputation of businesses, influencing customer perception and regulatory compliance.
Are there regulations or standards for AI model energy consumption?
Currently, there are no universal regulations or standards specifically addressing AI model energy consumption. However, organizations and industry bodies are actively exploring and advocating for sustainable AI practices, which may eventually result in standards or guidelines related to energy-efficient AI model development and usage.
Where can I find resources for optimizing AI model energy consumption?
Various resources, including research papers, industry guidelines, and development frameworks, provide information on optimizing AI model energy consumption. Additionally, collaborating with AI experts, energy consultants, or technology vendors can offer insights and assistance in the efficient use of AI technologies while minimizing energy consumption.