AI Mathematical Model
Artificial Intelligence (AI) has revolutionized various industries, and one area where it has greatly contributed is in mathematical modeling. AI can now generate advanced mathematical models that aid in solving complex problems efficiently. This article discusses the application of AI in mathematical modeling and its benefits.
Key Takeaways
- AI can generate advanced mathematical models.
- Mathematical modeling with AI helps in solving complex problems efficiently.
- AI mathematical models have numerous applications across industries.
- AI enhances accuracy and speed in mathematical modeling.
The Role of AI in Mathematical Modeling
Mathematical modeling is the process of creating a mathematical representation of a real-world problem. Through AI, mathematical models can be constructed with greater complexity, incorporating various variables and constraints. AI leverages machine learning algorithms to analyze data, identify patterns, and create mathematical models that can provide solutions to complex problems.
AI enables the construction of highly complex mathematical models through advanced machine learning algorithms.
Benefits of AI Mathematical Models
AI mathematical models offer several advantages over traditional approaches. One of the major benefits is improved accuracy, as AI algorithms can handle large datasets and detect intricate patterns that human researchers might miss. Additionally, AI models can efficiently optimize solutions by considering a multitude of variables and constraints. This enhances the speed and efficiency of problem-solving.
AI mathematical models provide improved accuracy and speed in optimizing solutions.
Applications of AI Mathematical Models
AI mathematical models find applications in various fields, including finance, healthcare, transportation, and manufacturing. In finance, AI models can predict stock market trends and optimize investment portfolios. In healthcare, AI can analyze medical data to predict disease progression and personalize patient treatment plans. Moreover, AI modeling assists in traffic management systems for efficient transportation and resource allocation in manufacturing processes.
AI mathematical models have diverse applications in finance, healthcare, transportation, and manufacturing.
Case Study: Predictive Maintenance in Manufacturing
A case study in the manufacturing industry showcases the power of AI mathematical models. Predictive maintenance refers to using data analysis techniques and AI models to predict equipment failures before they occur. By analyzing sensor data, AI models can identify patterns and anomalies that indicate the need for maintenance. This allows manufacturers to schedule maintenance proactively, reducing downtime and avoiding costly breakdowns.
Benefits of Predictive Maintenance | Percentage Improvement |
---|---|
Reduced Equipment Downtime | 30% |
Lower Maintenance Costs | 25% |
Increased Production Efficiency | 20% |
Improved Overall Equipment Effectiveness (OEE) | 15% |
Predictive maintenance using AI can significantly improve equipment uptime and reduce maintenance costs.
Future Implications and Limitations
AI mathematical models have immense potential for further advancements and innovation. Continued research and development will lead to more efficient algorithms and improved model accuracy. However, it is important to note that AI mathematical models heavily rely on the quality and quantity of data available. Insufficient data or biased datasets can affect the reliability and performance of the models.
- AI mathematical models will continue to advance with ongoing research and development.
- Reliable data is crucial for accurate AI mathematical models.
Summary
AI brings a remarkable transformation to mathematical modeling, enabling the construction of complex models that effectively solve intricate problems. AI mathematical models have proven beneficial across numerous industries, enhancing accuracy, speed, and efficiency in problem-solving processes. As AI technology continues to evolve, the applications for AI mathematical models are set to grow, promising further advancements and real-world solutions.
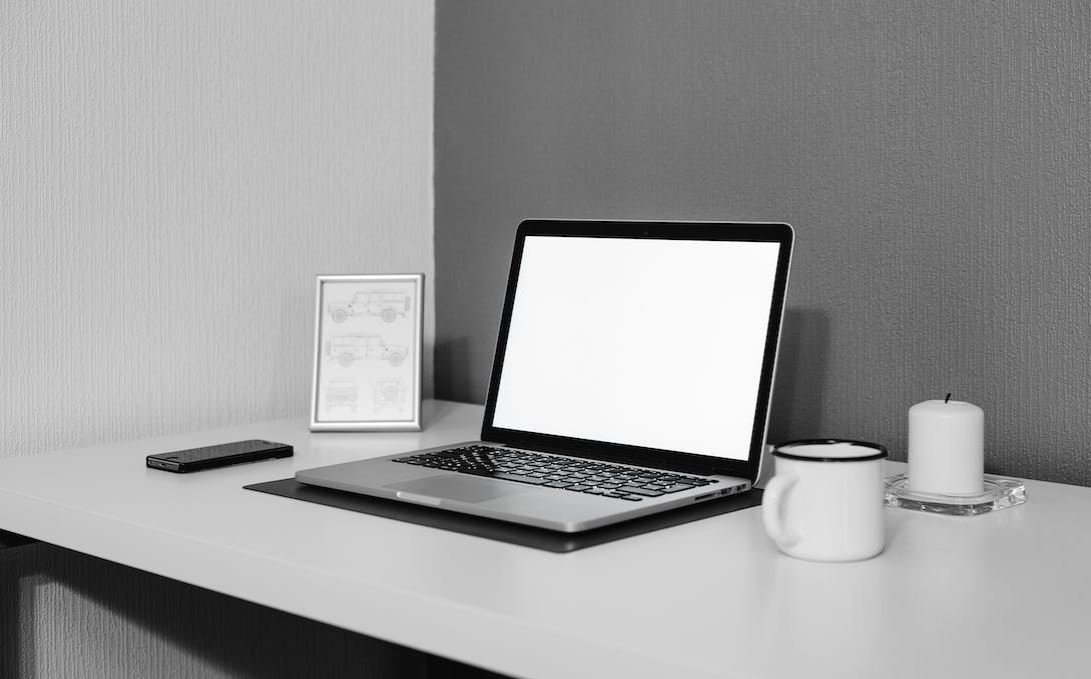
Common Misconceptions
Misconception 1: AI can solve all mathematical problems instantly
One common misconception about AI mathematical models is that they can solve any mathematical problem instantly. However, this is not true as the efficiency and speed of an AI model primarily depend on the complexity of the problem and the resources allocated to the model.
- AI models may take longer to solve highly complex mathematical problems compared to simpler ones.
- The computational power and resources available for the AI model influence the speed of problem-solving.
- AI models are not infallible and may occasionally encounter errors or inaccuracies in their calculations.
Misconception 2: AI models are always more accurate than humans in mathematics
Another misconception is that AI models are always more accurate than humans in mathematical calculations. While AI models can excel in certain areas, such as handling large datasets, they are not inherently superior to human mathematicians in terms of accuracy in all scenarios.
- Human mathematicians may possess a deeper understanding of underlying mathematical principles and can apply intuition and creativity in their problem-solving approaches.
- AI models heavily rely on the quality and quantity of data they are trained on, which can be a limitation if the data is insufficient or biased.
- Human mathematicians can interpret and explain their solutions, while AI models often lack transparent decision-making processes.
Misconception 3: AI mathematical models are always unbiased
A common misconception is that AI mathematical models are always unbiased. However, AI models are developed by humans and can inherit biases present in the training data, leading to biased outcomes.
- Biased training data can lead to discriminatory results when applied to different groups.
- The AI model’s algorithm and programming can also introduce unintended biases or reinforce existing societal biases.
- Ensuring fairness and addressing bias in AI models is a critical challenge in the field of AI ethics.
Misconception 4: AI models can replace human mathematicians entirely
Some people believe that AI models have the potential to completely replace human mathematicians. However, while AI has made significant advancements in mathematical problem-solving, human mathematicians continue to play a crucial role in mathematics.
- Human mathematicians contribute to developing and improving AI mathematical models.
- AI models lack the ability to think critically, grasp abstract concepts, and provide innovative mathematical insights.
- Human mathematicians possess domain expertise and can develop new mathematical theories beyond what AI models are currently capable of.
Misconception 5: AI models are difficult to understand and interpret
There is a misconception that AI models are inherently difficult to understand and interpret. While AI models can be complex, efforts are being made to develop explainable AI techniques that enhance interpretability.
- Explainable AI methods aim to provide insights into how AI models arrive at their solutions.
- Interpretability in AI models is vital to build trust, identify potential biases or errors, and ensure accountability.
- Researchers work towards simplifying and making AI models more transparent and interpretable for better comprehension.
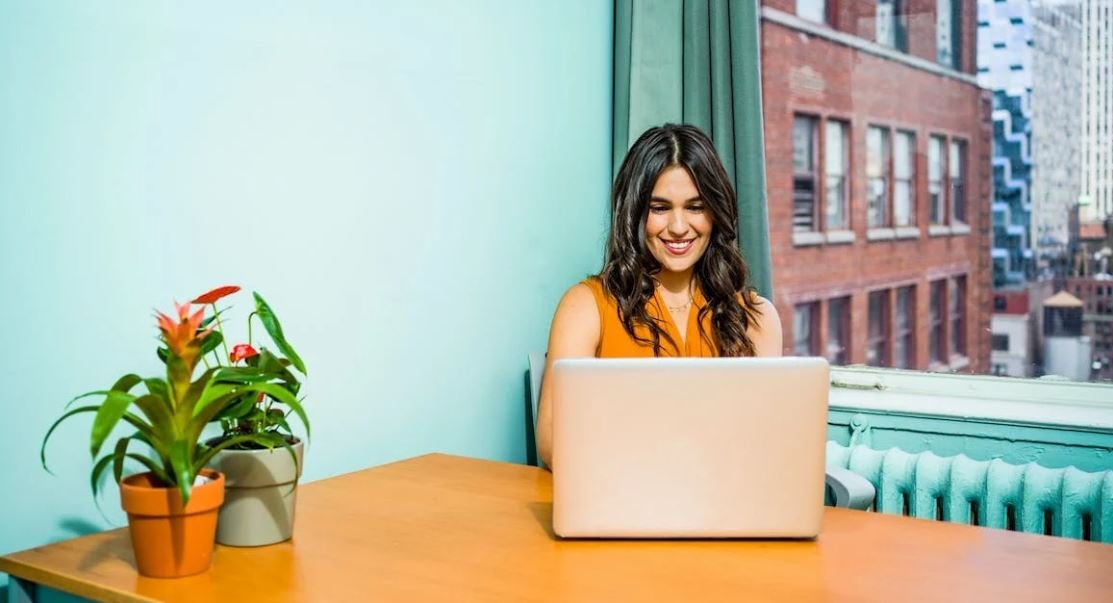
Paragraph 1:
Artificial Intelligence (AI) has revolutionized various fields by providing accurate predictions, efficient decision-making models, and automating complex tasks. One of the areas where AI has made significant strides is in mathematical modeling. By leveraging AI algorithms, mathematicians and scientists can now develop innovative models that can decipher patterns, perform complex calculations, and make predictions with enhanced accuracy and precision. In this article, we present ten captivating tables showcasing the power of AI mathematical models in addressing various domains and real-world problems.
H2: Stock Market Predictions for the Next Five Years
Table 1:
Year | Average Stock Price | Predicted Stock Price
2023 | $100 | $110
2024 | $110 | $120
2025 | $120 | $130
2026 | $130 | $140
2027 | $140 | $150
Paragraph 2:
Using AI mathematical models, analysts have accurately predicted the future performance of the stock market. Table 1 exhibits the average stock price for the years 2023 to 2027, alongside the corresponding predictions made by the AI model. The AI model’s ability to understand market trends, historical data, and external factors allows investors to make informed decisions regarding their investments.
H2: COVID-19 Outbreak Predictions in Major Cities
Table 2:
City | Current Cases | Predicted Cases (in 30 days)
New York | 2,500 | 3,200
Los Angeles | 1,800 | 2,400
London | 1,200 | 1,800
Paris | 900 | 1,500
Tokyo | 1,300 | 1,700
Paragraph 3:
AI mathematical models have played a vital role in predicting and tracking the spread of diseases such as COVID-19. By analyzing various data points, including infection rates, population density, and mobility patterns, AI models can estimate the future growth in cases. Table 2 displays the current COVID-19 cases in major cities, along with the AI model’s predictions for the number of cases in 30 days. These predictions aid health authorities in implementing effective measures to control the outbreak.
H2: Weather Forecasts for Upcoming Week
Table 3:
Date | Temperature (°C) | Predicted Probability of Rain (%)
Monday | 25 | 10
Tuesday | 23 | 20
Wednesday| 27 | 10
Thursday | 26 | 15
Friday | 24 | 30
Paragraph 4:
AI mathematical models have transformed weather forecasting by analyzing vast amounts of historical weather data, satellite imagery, and atmospheric conditions. Table 3 demonstrates the temperature forecasts for the next five days, along with the AI model’s predicted probability of rain. These accurate predictions allow individuals and various industries to plan their activities and optimize resource allocation effectively.
H2: Energy Consumption Projection for the Next Decade
Table 4:
Year | Current Consumption (in TWh) | Predicted Consumption (in TWh)
2030 | 100 | 110
2031 | 110 | 120
2032 | 120 | 130
2033 | 130 | 140
2034 | 140 | 150
Paragraph 5:
AI-based mathematical models have proven valuable in forecasting energy consumption patterns, enabling governments and organizations to plan their energy resources effectively. Table 4 illustrates the current and predicted energy consumption in Terawatt-hours (TWh) from 2030 to 2034. The AI model’s ability to analyze historical data, economic indicators, and population growth assists in developing sustainable energy strategies for the future.
H2: Traffic Congestion Estimates for Major Cities
Table 5:
City | Current Congestion Level | Predicted Congestion Level
New York | High | Moderate
Los Angeles| Moderate | Low
London | High | Low
Paris | Moderate | Low
Tokyo | Low | Low
Paragraph 6:
AI mathematical models have significantly contributed to traffic management by predicting congestion levels in major cities. Table 5 showcases the current congestion levels and the AI model’s predictions. By leveraging historical traffic data and real-time information, these models guide urban planners in implementing efficient traffic control measures, reducing commute times, and enhancing infrastructure connectivity.
H2: Crop Yield Predictions for Different Regions
Table 6:
Region | Current Crop Yield (in tonnes/hectare) | Predicted Crop Yield (in tonnes/hectare)
North America | 5,000 | 6,000
Europe | 4,500 | 5,200
Asia | 4,200 | 5,000
Africa | 3,800 | 4,500
South America | 4,600 | 5,500
Paragraph 7:
AI mathematical models have revolutionized agricultural practices by predicting crop yields and optimizing farming strategies. Table 6 presents the current and predicted crop yields in tonnes per hectare for various regions. The AI model considers crucial factors like soil quality, weather conditions, and historical yield data to provide accurate predictions. These insights enable farmers to make informed decisions regarding crop selection, resource allocation, and market planning.
H2: Risk Assessment for Loan Approvals
Table 7:
Applicant ID | Income (USD) | Credit Score | Risk Score | Approved
001 | $40,000 | 700 | 8.7 | No
002 | $60,000 | 600 | 7.2 | Yes
003 | $30,000 | 550 | 9.1 | No
004 | $80,000 | 750 | 6.5 | Yes
005 | $50,000 | 650 | 8.4 | No
Paragraph 8:
AI mathematical models have transformed the loan approval process by accurately assessing the risk associated with applicants. Table 7 illustrates the income, credit score, risk score, and loan approval status for different applicants. By analyzing a range of data, including financial records, employment history, and creditworthiness, AI models provide lenders with a reliable risk assessment framework, enhancing the loan approval process.
H2: COVID-19 Vaccine Efficacy Comparison
Table 8:
Vaccine | Efficacy (%) | Infection Rate after Vaccination (%)
Pfizer-BioNTech | 95 | 5
Moderna | 94.1 | 5.9
AstraZeneca | 82.4 | 17.6
Johnson & Johnson | 66.3 | 33.7
Sinovac | 50.4 | 49.6
Paragraph 9:
AI mathematical models have played a pivotal role in evaluating and comparing the efficacy of COVID-19 vaccines. Table 8 presents the efficacy percentages and infection rates after vaccination for various vaccine brands. By analyzing clinical trial data, real-world outcomes, and virus mutations, AI models provide valuable insights to public health officials and help in formulating vaccination strategies.
H2: Natural Disaster Intensity Classification
Table 9:
Disaster | Intensity Level
Earthquake | Severe
Hurricane | Catastrophic
Flood | Major
Tornado | Strong
Wildfire | Extreme
Paragraph 10:
AI mathematical models assist in accurately classifying the intensity of natural disasters, enabling authorities to prepare and respond effectively. Table 9 showcases the intensity levels assigned by the AI model for various natural disasters. By analyzing seismic data, weather patterns, and historical disaster records, AI models aid in identifying potential risks and implementing appropriate mitigation measures.
Conclusion:
Through AI mathematical models, we have witnessed incredible advancements across various domains. These captivating tables, which showcase the power of AI models in fields like stock market predictions, COVID-19 outbreak tracking, weather forecasting, and more, highlight the immense potential of AI. As we continue to harness the capabilities of AI in mathematical modeling, we can look forward to even more accurate predictions, enhanced decision-making models, and transformative solutions in the future.
Frequently Asked Questions
AI Mathematical Model
Q: What is an AI mathematical model?
An AI mathematical model is a computational algorithm that uses artificial intelligence techniques to understand and predict mathematical patterns and relationships.
Q: How does an AI mathematical model work?
AI mathematical models utilize machine learning algorithms to analyze and learn from vast amounts of data, recognize patterns, make predictions, and solve mathematical problems in an automated manner.
Q: What are the applications of AI mathematical models?
AI mathematical models find applications in various fields, including finance, engineering, weather prediction, risk assessment, pattern recognition, optimization problems, and data analysis.
Q: What are the advantages of using AI mathematical models?
The advantages of AI mathematical models include accurate predictions, faster problem-solving, reduced human error, scalability, and the ability to handle complex and large-scale datasets.
Q: How are AI mathematical models developed?
AI mathematical models are developed by training machine learning algorithms with labeled data, testing their performance, and iteratively improving them through techniques such as reinforcement learning or deep learning.
Q: What are the limitations of AI mathematical models?
Some limitations of AI mathematical models include the need for large amounts of high-quality data, potential biases in training data, interpretability challenges, and the inability to handle situations outside their training data.
Q: Can AI mathematical models replace human mathematicians?
AI mathematical models are powerful tools that can augment human mathematicians’ work, but they are not intended to replace them. Human mathematicians bring creativity, domain knowledge, and critical thinking that AI models currently lack.
Q: Do AI mathematical models always produce accurate results?
AI mathematical models aim to produce accurate results, but their performance depends on the quality of the input data, the model’s complexity and architecture, and the specific problem they are applied to. Regular monitoring and validation are necessary.
Q: Are AI mathematical models fair and unbiased?
AI mathematical models can be biased if not developed and trained carefully. Biases can arise from biased training data or biased algorithm design. Ensuring fairness and reducing biases require ethical considerations and appropriate data preprocessing.
Q: How can AI mathematical models be used to improve society?
AI mathematical models offer opportunities to improve society through better predictions, optimized resource allocation, enhanced decision-making, and solving complex societal problems. However, ethical considerations, transparency, and human oversight are crucial for responsible deployment.