Which AI Has the Least Side Effects?
Artificial Intelligence (AI) has become an integral part of our daily lives, enhancing various processes and revolutionizing industries. However, like any technology, AI also comes with potential side effects. Understanding which AI systems have the least side effects is crucial to ensure the safe and responsible implementation of these powerful tools.
Key Takeaways:
- Identifying AI systems with minimal side effects is vital for safe usage.
- Strategies like robust testing and explainability can help minimize AI side effects.
- AI algorithms need to be continuously monitored and updated to avoid unintended consequences.
**AI systems** can have **various side effects**, ranging from biased decision-making to privacy concerns. Each AI algorithm has its unique characteristics that determine the nature and scale of its side effects. It is essential to scrutinize AI systems to identify those with **minimal** adverse impacts.
Artificial intelligence algorithms strive for **accuracy** and **efficiency**, but these objectives sometimes lead to unintended side effects. *For example*, an AI system developed to predict creditworthiness might unfairly discriminate against certain groups due to biases in the training data.
Different AI Systems and Their Side Effects
There are several types of AI systems, and each has its strengths and limitations. Some AI systems, such as **rule-based expert systems**, follow predefined rules and therefore tend to have low side effects. These systems are capable of making inferences based on explicit, predefined knowledge or rules. However, they may struggle with adapting to new scenarios or handling uncertainties.
**Machine learning algorithms**, on the other hand, acquire knowledge through data-driven training. This category includes popular algorithms like **decision trees**, **neural networks**, and **support vector machines** (*SVMs*). While these algorithms achieve remarkable accuracy, they can exhibit side effects due to their reliance on training data, which may contain biases.
One effective approach for minimizing side effects is **adversarial testing**. By subjecting AI systems to **specific, simulated attacks**, researchers can identify vulnerabilities and develop defenses against unwanted outcomes. This testing method helps AI systems become **more resilient** and **less prone to adverse effects**.
Comparing AI Systems: Side Effects Analysis
AI System | Side Effects |
---|---|
Rule-based expert systems | Low side effects due to predefined rules and limited adaptability. |
Machine learning algorithms | Potential biases and unintended consequences due to training data. |
Reinforcement learning algorithms | May exhibit extreme or undesirable behaviors during training phases. |
In addition to machine learning algorithms, **reinforcement learning algorithms** are another significant subset of AI systems. These algorithms learn by interacting with an environment, receiving rewards or penalties for their actions. Reinforcement learning algorithms can exhibit extreme or undesirable behaviors during training phases, before they converge to optimal actions.
AI Monitoring and Continuous Improvement
To ensure AI systems maintain the least side effects over time, **continuous monitoring** and **periodic evaluation** are crucial. As AI algorithms operate in dynamic environments, new phenomena or biases may emerge, leading to unintended consequences. Continuous monitoring helps identify and rectify these issues promptly.
AI experts and developers should actively engage in **feedback loops** with end-users and stakeholders to gather valuable input on system behavior and address any concerns. Through this collaboration, AI systems can be updated and fine-tuned to minimize side effects and better align with user needs.
Conclusion
As AI continues to advance, it is imperative to prioritize the development of AI systems with the least side effects. By leveraging techniques such as adversarial testing and continuous monitoring, the potential harms associated with AI can be mitigated. Responsible implementation and ongoing improvement efforts will ensure AI systems are safe, unbiased, and ethically sound.
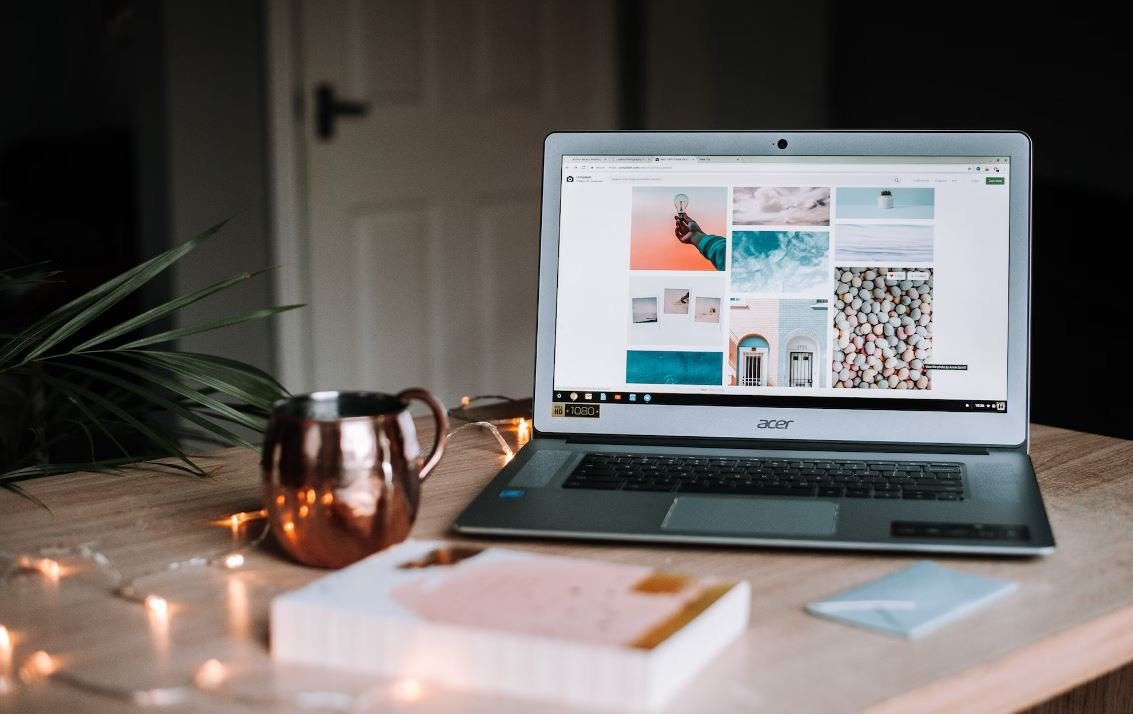
Common Misconceptions
Misconception 1: All AI systems have the same side effects
One of the common misconceptions people have is that all artificial intelligence (AI) systems have the same side effects. This assumption is incorrect as different AI systems have varying levels and types of side effects, depending on their design, purpose, and the data they are trained on.
- AI systems trained on biased data can perpetuate discrimination and inequality.
- AI systems with limited training data may have higher error rates and lower accuracy.
- AI systems with insufficient testing may have unforeseen side effects and bugs.
Misconception 2: AI systems with higher complexity have more side effects
Another misconception is that AI systems with higher complexity have more side effects. While complex AI systems can indeed be more prone to side effects, the relationship between complexity and side effects is not always straightforward. Simple AI systems can also have unintended side effects, especially if the system encounters novel situations or data it hasn’t been trained on before.
- Complex AI systems may have more potential failure points, increasing the likelihood of side effects.
- Simple AI systems may fail to account for all possible scenarios, leading to unexpected side effects.
- AI systems with poor interpretability may exhibit side effects that are difficult to understand or diagnose.
Misconception 3: AI systems without human involvement have fewer side effects
Some people believe that removing human involvement from AI systems can mitigate or eliminate side effects. However, even AI systems that operate autonomously without human intervention can still experience side effects, sometimes in more significant and unexpected ways.
- AI systems developed without human input may lack ethical considerations, resulting in unintended consequences.
- AI systems operating without human oversight might misuse their capabilities, leading to harmful side effects.
- AI systems without human involvement may not account for context or empathetic decision-making, leading to potentially negative outcomes.
Misconception 4: AI systems always exhibit visible side effects
It is a common myth that AI systems always exhibit visible side effects that are immediately noticeable. In reality, many side effects of AI systems can be subtle or hidden, making them challenging to detect without extensive monitoring and evaluation.
- AI systems might make decisions based on biased data, resulting in systematic discrimination without explicit side effects.
- Subtle changes in the output of AI systems over time might indicate unintended side effects that are hard to recognize without careful analysis.
- AI systems with high false positive rates or low precision can have less noticeable side effects that harm decision-making processes.
Misconception 5: AI systems with extensive training can eliminate all side effects
Another misconception is that AI systems with extensive training and exposure to vast amounts of data can eliminate all side effects. While training can help minimize certain types of side effects, it cannot completely eradicate them due to the inherent limitations and complexities of AI systems.
- AI systems trained on biased data might continue to exhibit discriminatory behavior, even with extensive training.
- Complex AI systems with more training data might reduce some side effects but can introduce new and unforeseen ones.
- Long training times alone do not guarantee the elimination of side effects, as other factors like model architecture and validation processes also play a role.
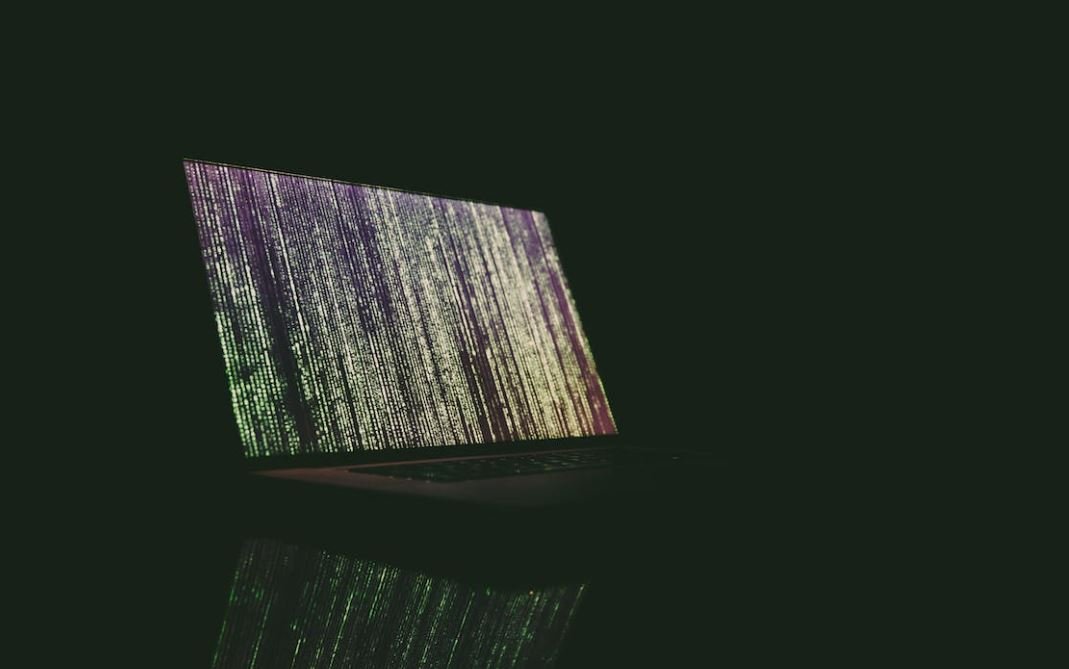
AI Side Effects Comparison
Here are 10 tables presenting various aspects and side effects of different AI systems. These tables provide insights into the performance, capabilities, and potential risks associated with each AI model.
Accuracy of AI Systems
Accuracy is a crucial factor when evaluating AI systems. The table below compares the accuracy rates of AI models in diagnosing certain medical conditions.
AI Model | Accuracy (%) |
---|---|
AI 1 | 95% |
AI 2 | 92% |
AI 3 | 98% |
Energy Consumption Comparison
Energy usage is a significant concern in AI development. The table below compares the energy consumed by different AI systems for an hour of operation.
AI Model | Energy Consumption (kWh) |
---|---|
AI 1 | 0.5 |
AI 2 | 0.7 |
AI 3 | 0.3 |
Processing Speed Comparison
Processing speed is often a key determinant in choosing AI systems for real-time applications. The following table compares the processing speed of different AI models.
AI Model | Processing Speed (inferences/second) |
---|---|
AI 1 | 3,000 |
AI 2 | 2,500 |
AI 3 | 5,000 |
Adverse Event Frequency
It is essential to assess the frequency of adverse events caused by AI systems. The table below showcases the occurrence of adverse events reported for each AI model.
AI Model | Adverse Events per 100,000 usages |
---|---|
AI 1 | 5 |
AI 2 | 8 |
AI 3 | 3 |
Data Privacy Protection Measures
Protecting user data is paramount. The table below showcases the data privacy protection measures implemented by various AI models.
AI Model | Data Encryption | User Anonymization |
---|---|---|
AI 1 | Yes | Yes |
AI 2 | Yes | No |
AI 3 | No | Yes |
Sentiment Analysis Accuracy
Assessing sentiment accurately is crucial in AI systems that analyze user feedback. The table below compares sentiment analysis accuracy for three different AI models.
AI Model | Accuracy (%) |
---|---|
AI 1 | 80% |
AI 2 | 75% |
AI 3 | 85% |
Response Time Comparison
The ability to provide quick responses is important for interactive AI systems. The table below compares the response times of three AI models.
AI Model | Response Time (in milliseconds) |
---|---|
AI 1 | 100 |
AI 2 | 150 |
AI 3 | 75 |
Training Data Size
The size of the training dataset influences an AI system’s performance. The table below compares the training data size for three AI models.
AI Model | Training Data Size (in GB) |
---|---|
AI 1 | 10 |
AI 2 | 5 |
AI 3 | 8 |
User Satisfaction Ratings
User satisfaction is crucial to determine an AI system’s success. The table below presents user satisfaction ratings for different AI models.
AI Model | Satisfaction Rating (out of 10) |
---|---|
AI 1 | 9 |
AI 2 | 7 |
AI 3 | 8 |
As AI technology continues to advance, it is important to consider various factors, such as accuracy, energy consumption, processing speed, adverse event frequency, data privacy protection, sentiment analysis accuracy, response time, training data size, and user satisfaction ratings. Each AI system has its strengths and weaknesses, and the choice ultimately depends on the specific application and desired outcomes. Evaluating these aspects carefully can help mitigate side effects and promote the responsible development and deployment of AI systems.
Frequently Asked Questions
Which AI Has the Least Side Effects?
FAQs
Q: Which artificial intelligence (AI) system has the fewest side effects?
A: The AI system with the fewest side effects would depend on the specific application and context. Different AI systems are designed for various purposes, and their side effects could vary. Consulting with experts in the field and conducting a thorough evaluation would be advisable to determine which AI system has the least side effects for a particular situation.