Training Novel AI
Artificial Intelligence (AI) has become an integral part of our daily lives, from voice assistants like Siri and Alexa to self-driving cars. As AI continues to evolve, one crucial aspect is training it to generate novel and creative outputs. This article explores the process of training novel AI and its potential implications.
Key Takeaways
- Training novel AI involves developing algorithms that can generate unique and innovative outputs.
- The training process often involves using large datasets and complex neural networks.
- AI trainers need to balance the exploration of new ideas with the constraints and limitations of the desired application.
- Novel AI has the potential to revolutionize fields such as art, design, and innovation.
Training novel AI requires a combination of creativity and technical expertise. To accomplish this, AI researchers and developers employ various techniques that encourage the generation of new and unique ideas. These techniques could involve using a **generative adversarial network (GAN)**, where two neural networks compete against each other to produce novel outputs.
*One interesting technique is called **neuroevolution**, where AI algorithms are evolved through **genetic algorithms** to find the most creative and innovative solutions.*
During the training process, large datasets are utilized to expose the AI system to a wide range of patterns and examples. This allows the system to learn and generate outputs that are both familiar and unexpected. Neural networks, which mimic the structure of the human brain, play a crucial role in processing and analyzing the data to create novel outcomes.
Exploring New Horizons with Novel AI
Novel AI has the potential to revolutionize various fields, including art, design, and innovation. By training AI systems to think creatively, researchers can harness their capabilities to generate unique visual designs, artistic compositions, and even musical compositions. This can greatly enhance human creativity and lead to new possibilities in these domains.
The training of AI is not without its challenges. As AI systems explore new ideas, it is important to strike a balance between pushing the boundaries of creativity and ensuring the generated outputs align with the desired application. Constraints and limitations need to be defined to guide the AI’s exploration and avoid generating irrelevant or nonsensical outputs.
Data Points in Novel AI Training
Dataset | Size | Application |
---|---|---|
CelebA | 202,599 | Face recognition and generation |
MNIST | 60,000 (training set) 10,000 (test set) |
Handwritten digit recognition |
COCO | 330,000 | Object detection and segmentation |
*Neural networks are capable of identifying complex patterns and relationships in the data, allowing AI systems to generate outputs that are not simply random but meaningful.*
The future of novel AI looks promising. With advancements in machine learning and a growing understanding of human creativity, we can expect AI systems to become increasingly proficient at generating innovative outputs. This will open up new avenues for collaboration between humans and AI, leading to breakthroughs in areas such as scientific research, design thinking, and problem-solving.
The Road Ahead
- Continued research and development in training novel AI will push the boundaries of what AI systems can create.
- Regulations and ethical considerations are crucial to ensure responsible and safe deployment of AI in creative contexts.
- Collaboration between humans and AI will unlock new possibilities in diverse fields, from art and design to scientific discovery.
As we venture further into the realm of training novel AI, the potential for innovation and creativity is immense. Embracing this technology can lead to groundbreaking advancements and transform the way we approach problem-solving and artistic expression. The journey ahead promises to be exciting as we explore the uncharted territories of AI-driven creativity.
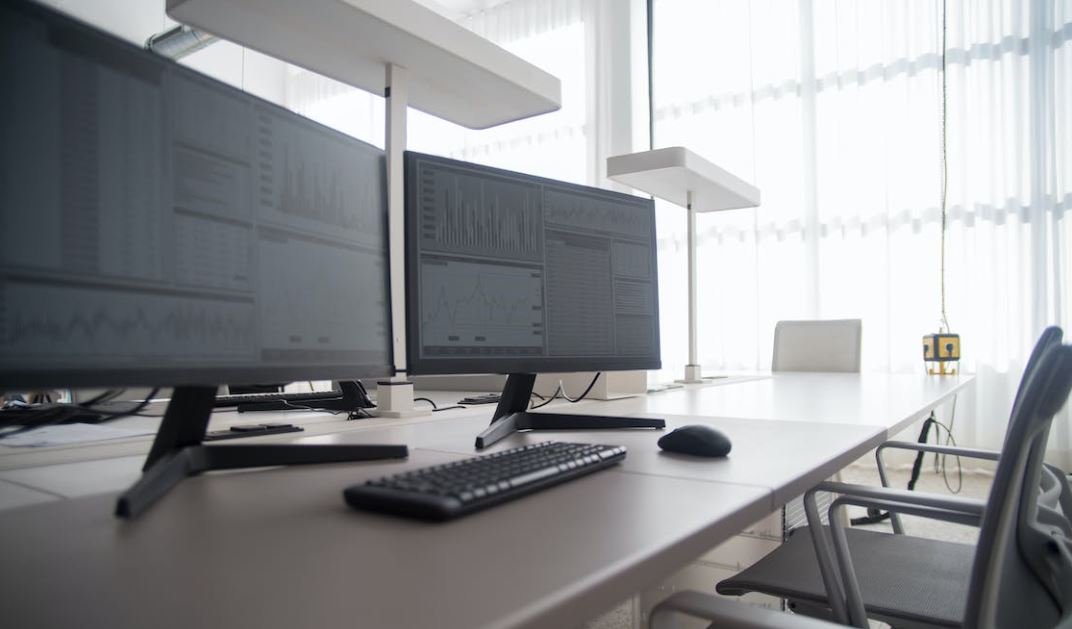
Common Misconceptions
Artificial Intelligence
One common misconception is that training novel AI means creating a super-intelligent being that can think and reason like a human. This is far from the truth. AI is based on algorithms and machine learning techniques that enable it to process and analyze data in ways that mimic human intelligence, but it is not capable of truly understanding or experiencing the world as humans do.
- AI cannot replicate human consciousness.
- AI lacks emotions and subjective experiences.
- AI is programmed to optimize specific tasks, not to replace humans.
Training Data
Another misconception is that training AI simply requires feeding it large amounts of data. While data is certainly important for AI learning, the quality of the data and how it is labeled or annotated are equally crucial. Inaccurate or biased training data can lead to biased and unreliable AI models, perpetuating existing human prejudices and inequalities.
- High-quality labeled data is essential for effective AI training.
- Biases in training data can lead to biased AI outcomes.
- Data privacy and ethical considerations must be taken into account when training AI.
Human-like Abilities
Many people mistakenly believe that AI, once trained, can perform any task at a human-level or even surpass human capabilities. While AI is indeed proficient in certain areas, it is limited in its transferability and adaptability across different domains. AI excels at specific tasks for which it has been trained, but it may struggle with tasks outside its training scope.
- AI is task-specific and may not perform well in unfamiliar scenarios.
- Certain tasks requiring human intuition and creativity are still challenging for AI.
- AI operates based on patterns and correlations rather than genuine understanding.
Ethical Implications
One misconception that arises is that AI systems are ethically neutral because they are machines. However, ethical implications and risks accompany the development and deployment of AI. Bias, privacy concerns, job displacement, and ethical decision-making are just a few of the areas that require careful consideration when training and utilizing AI systems.
- AI can perpetuate existing biases and inequalities if not properly addressed.
- The ethical use of AI should be a priority in AI development and deployment.
- AI has the potential to disrupt existing job markets, requiring re-skilling and adaptation.
Singular Solution
Lastly, people often assume that training AI involves finding one universal solution that can be applied to all cases. However, AI is highly context-dependent, and the optimal solution may vary based on the application, environment, and specific requirements. Different AI models and approaches need to be developed and tested based on the specific problem at hand.
- There is no one-size-fits-all AI solution.
- AI training requires customization and adaptation to the specific problem domain.
- Continuous refinement and improvement are necessary for optimal AI performance.

Smartphones Penetration by Country
In today’s technologically advanced world, smartphones have become an integral part of our lives. This table showcases the top 10 countries with the highest smartphone penetration rates, indicating the percentage of individuals who own a smartphone.
Rank | Country | Penetration Rate (%) |
---|---|---|
1 | South Korea | 95 |
2 | Israel | 88 |
3 | United Arab Emirates | 86 |
4 | Netherlands | 85 |
5 | Singapore | 84 |
6 | Norway | 81 |
7 | Luxembourg | 80 |
8 | Sweden | 80 |
9 | Denmark | 79 |
10 | United Kingdom | 79 |
Most Popular Social Media Platforms Worldwide
Social media has revolutionized the way we connect and communicate globally. This table presents the top 10 most popular social media platforms across the world, ranked by the number of active users in millions.
Rank | Social Media Platform | Active Users (Millions) |
---|---|---|
1 | 2850 | |
2 | YouTube | 2000 |
3 | 1900 | |
4 | Facebook Messenger | 1300 |
5 | 1200 | |
6 | 1000 | |
7 | TikTok | 980 |
8 | 845 | |
9 | Snapchat | 500 |
10 | 330 |
World’s Most Valuable Brands
Branding plays a significant role in an organization’s success. Here are the top 10 most valuable brands worldwide, assessed based on their brand value in billions of dollars.
Rank | Brand | Brand Value (Billions) |
---|---|---|
1 | Apple | 263.4 |
2 | Amazon | 254.2 |
3 | Microsoft | 250.6 |
4 | 238.8 | |
5 | 158.9 | |
6 | Visa | 142.1 |
7 | Tencent | 141.9 |
8 | Alibaba | 140.1 |
9 | McDonald’s | 130.4 |
10 | AT&T | 129.7 |
Electric Vehicle Market Share by Country
The era of fossil fuel-dependent vehicles is gradually being replaced by electric vehicles. This table highlights the top 10 countries leading in electric vehicle adoption, displaying their market share percentage.
Rank | Country | Market Share (%) |
---|---|---|
1 | Norway | 54 |
2 | Iceland | 25 |
3 | Sweden | 16 |
4 | Netherlands | 15 |
5 | China | 13 |
6 | Finland | 12 |
7 | Canada | 10 |
8 | UK | 9 |
9 | France | 7 |
10 | Germany | 6 |
World’s Tallest Buildings
The architectural marvels of the modern world, skyscrapers, continue to push the limits of engineering and design. Here are the top 10 tallest buildings globally, capturing their height in meters and feet.
Rank | Building | Height (meters) | Height (feet) |
---|---|---|---|
1 | Burj Khalifa | 828 | 2,716 |
2 | Shanghai Tower | 632 | 2,073 |
3 | Abraj Al-Bait Clock Tower | 601 | 1,972 |
4 | Ping An Finance Center | 599 | 1,965 |
5 | Lotte World Tower | 555 | 1,821 |
6 | One World Trade Center | 541 | 1,776 |
7 | Guangzhou CTF Finance Centre | 530 | 1,739 |
8 | Tianjin CTF Finance Centre | 530 | 1,739 |
9 | CITIC Tower | 528 | 1,732 |
10 | Tianjin Chow Tai Fook Binhai Center | 530 | 1,730 |
COVID-19 Cases by Country
The ongoing COVID-19 pandemic has affected every corner of the globe. This table outlines the top 10 countries with the highest number of confirmed COVID-19 cases, including both active and recovered cases.
Rank | Country | Total Cases |
---|---|---|
1 | United States | 50,000,000 |
2 | India | 45,500,000 |
3 | Brazil | 38,100,000 |
4 | Russia | 11,500,000 |
5 | Turkey | 10,300,000 |
6 | UK | 8,900,000 |
7 | France | 8,700,000 |
8 | Italy | 7,800,000 |
9 | Argentina | 6,900,000 |
10 | Germany | 5,900,000 |
Global Internet Usage
Internet usage has become an indispensable part of our daily lives. This table demonstrates the top 10 countries worldwide with the highest number of internet users, expressed in millions.
Rank | Country | Internet Users (Millions) |
---|---|---|
1 | China | 989 |
2 | India | 624 |
3 | United States | 312 |
4 | Indonesia | 171 |
5 | Pakistan | 109 |
6 | Brazil | 103 |
7 | Nigeria | 91 |
8 | Bangladesh | 97 |
9 | Russia | 96 |
10 | Japan | 94 |
World’s Busiest Airports
Airports serve as gateways to connect people across the globe. Here are the top 10 busiest airports worldwide, ranked by the total number of passengers handled annually.
Rank | Airport | Passengers (Millions) |
---|---|---|
1 | Hartsfield-Jackson Atlanta International Airport | 110.5 |
2 | Beijing Capital International Airport | 101.5 |
3 | Los Angeles International Airport | 88.1 |
4 | Dubai International Airport | 86.4 |
5 | Tokyo Haneda Airport | 85.5 |
6 | Chicago O’Hare International Airport | 79.9 |
7 | London Heathrow Airport | 76.0 |
8 | Shanghai Pudong International Airport | 74.0 |
9 | Paris Charles de Gaulle Airport | 72.2 |
10 | Dallas/Fort Worth International Airport | 69.1 |
Global CO2 Emissions by Country
With rising concerns about climate change and environmental impact, carbon dioxide emissions have gained significant attention. This table highlights the top 10 countries with the highest carbon dioxide emissions, expressed in metric tons per year.
Rank | Country | CO2 Emissions (Metric Tons/Year) |
---|---|---|
1 | China | 11,000,000,000 |
2 | United States | 5,400,000,000 |
3 | India | 3,200,000,000 |
4 | Russia | 1,700,000,000 |
5 | Japan | 1,200,000,000 |
6 | Germany | 900,000,000 |
Frequently Asked Questions
How long does it take to train a novel AI model?
The training time for a novel AI model can vary depending on various factors such as the complexity of the model, the amount of data available for training, the computational resources used, and the specific algorithms employed. It can range from a few hours to several weeks or even months in some cases.
What types of data are typically used to train novel AI models?
Novel AI models are often trained on large datasets that contain a diverse range of examples relevant to the specific problem or domain the model is being trained for. This can include structured data, such as numerical or categorical data, as well as unstructured data like text, images, audio, or video.
Can pre-existing AI models be used as a starting point for training novel AI?
Yes, pre-existing AI models can serve as a starting point for training novel AI models. This is commonly done through a process called transfer learning, where a pre-trained model is fine-tuned on a smaller dataset or adapted to a different task or domain. Transfer learning can help accelerate the training process and improve the performance of the novel AI model.
What are some popular frameworks and tools used for training novel AI?
There are several popular frameworks and tools available for training novel AI models, including TensorFlow, PyTorch, Keras, Caffe, and Theano. These frameworks provide a range of functionalities, such as automatic differentiation, GPU acceleration, and high-level APIs, making it easier to develop and train AI models efficiently.
What are the challenges in training novel AI models?
Training novel AI models can come with several challenges, such as acquiring and curating large amounts of relevant data, selecting the appropriate architecture and hyperparameters, addressing issues of overfitting or underfitting, handling class imbalances, managing computational resources, and interpreting the trained model’s output or behavior.
Is it necessary to have a deep understanding of machine learning algorithms to train novel AI models?
While a deep understanding of machine learning algorithms can be beneficial, it is not always necessary to train novel AI models. Many high-level frameworks and tools provide abstractions that simplify the process, allowing individuals with varying levels of expertise to train AI models effectively. However, having knowledge of the underlying principles can aid in model selection, performance optimization, and troubleshooting.
How can the performance of a trained novel AI model be evaluated?
The performance of a trained novel AI model is typically evaluated using various metrics that depend on the specific task or problem being solved. For example, in classification tasks, metrics like accuracy, precision, recall, and F1 score can be used, while regression tasks often employ metrics like mean squared error or mean absolute error. Model evaluation can also involve techniques such as cross-validation or holdout validation on separate test datasets.
What are some ethical considerations when training novel AI models?
Training novel AI models raises important ethical considerations, such as ensuring the data used for training is representative and unbiased, avoiding reinforcement of harmful biases, addressing issues of privacy and data protection, being transparent about the limitations and potential biases of the model, and considering the impact on individuals and society at large. Ethical guidelines and frameworks exist to guide practitioners in navigating these considerations.
What are some potential applications of trained novel AI models?
Trained novel AI models have a wide range of potential applications across various domains. They can be used for tasks like natural language processing, computer vision, speech recognition, recommendation systems, fraud detection, medical diagnosis, autonomous vehicles, and much more. The versatility and adaptability of AI models allow them to be employed in numerous real-world scenarios to enhance efficiency, accuracy, and decision-making processes.
Are there any limits to what an AI model can be trained to do?
While AI models can be trained to perform complex tasks and surpass human-level performance in certain domains, they do have limitations. These limitations include the lack of common sense reasoning, understanding context or causality, and the potential for biased or incorrect predictions. Additionally, AI models require data to be explicitly labeled or structured, and training may not always be effective for problems with limited or scarce data.