Train AI with Your Own Images
Artificial Intelligence (AI) has become an integral part of many industries, providing valuable insights, automating tasks, and enhancing decision-making processes. One key aspect of AI development is training the algorithms with relevant data to improve their performance. While there are vast amounts of publicly available datasets, sometimes it’s more effective to train AI with your own images. In this article, we will explore the benefits and processes of training AI using your own image data.
Key Takeaways:
- Training AI with your own images allows for personalized and specific recognition or classification tasks.
- Collecting and labeling a diverse dataset is crucial to improve AI accuracy and generalization.
- Transfer learning can help leverage pre-trained models and accelerate the training process.
- Regularly evaluating and fine-tuning AI models is necessary to adapt to changing data patterns and ensure ongoing accuracy.
- Cloud-based AI platforms offer accessible and efficient tools for training and deploying AI models.
Training AI with your own images enables personalized and specific recognition or classification tasks. Whether it’s identifying objects in photographs, detecting anomalies in medical images, or recognizing product defects on assembly lines, custom datasets can be instrumental in achieving accurate and insightful results. By using your own images, you can tailor the AI model to address the unique challenges of your industry or application.
*It’s fascinating to witness how AI algorithms can learn complex patterns and recognize objects based on visual cues.*
Creating an effective dataset for AI training involves collecting and labeling a diverse range of images. **Labeling images with appropriate tags** provides the necessary context for the AI model to understand and generalize its knowledge. The quality and variety of images in the dataset play a crucial role in ensuring that the model learns effectively, accurately representing the data it is expected to work with.
The Importance of Transfer Learning
Training AI models from scratch can be a time-consuming process, requiring significant computational resources. However, **transfer learning** offers a powerful technique to expedite the training process. By utilizing pre-trained models, which have been trained on large and general datasets, you can leverage the model’s learned features and apply them to your specific task. This approach jumpstarts the learning process and allows your model to achieve good performance even with a limited amount of labeled data.
*Transfer learning empowers developers to quickly build AI models without starting from scratch, making the development process more efficient.*
Regular Evaluation and Fine-Tuning
Once an AI model is trained, it is crucial to evaluate its performance regularly to ensure ongoing accuracy and relevance. This process involves testing the model on new, previously unseen images and comparing the results with ground truth labels. **Fine-tuning** the model based on the evaluation feedback can help address any weaknesses or errors it may encounter. Continuous evaluation and refinement allow the AI model to adapt to changing data patterns and maintain optimal accuracy over time.
*Constant evaluation and refinement ensure that AI models continue to deliver accurate and reliable results.*
The Power of Cloud-Based AI Platforms
Building and training AI models can be resource-intensive, requiring powerful hardware and extensive computational resources. Cloud-based AI platforms offer accessible and efficient solutions for training and deploying AI models. These platforms provide scalable infrastructure, pre-configured frameworks, and managed services, allowing developers to focus on the training process rather than infrastructure setup. They also offer sophisticated APIs that make it easy to integrate the trained models into various applications and environments.
Platform | Scalability | Pre-configured Models | Managed Services | APIs |
---|---|---|---|---|
Platform A | High | Yes | Yes | Yes |
Platform B | Medium | No | Yes | Yes |
Platform C | Low | Yes | No | Yes |
*Cloud-based AI platforms streamline the AI development process by providing scalable infrastructure and managed services.*
In conclusion, training AI with your own images offers the advantage of customization and specificity to your unique requirements. Building an effective dataset, leveraging transfer learning, and fine-tuning the models are essential steps in creating accurate and reliable AI systems. Cloud-based AI platforms provide accessible and efficient tools to simplify the training and deployment processes. So why settle for generic AI models when you can have one tailored to your needs with your own images?
References:
- Reference 1: [Link]
- Reference 2: [Link]
- Reference 3: [Link]
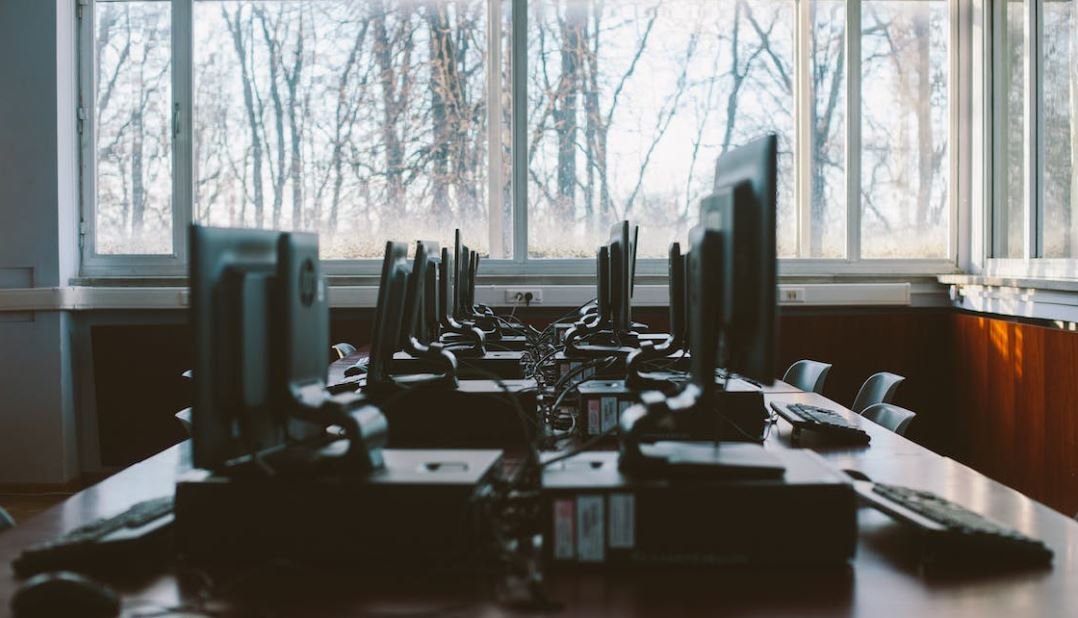
Common Misconceptions
Misconception 1: Anyone can train AI with their own images with minimal effort
One common misconception surrounding training AI with your own images is that it is a simple and effortless process. However, this is not the case, as it requires knowledge of machine learning algorithms and data annotation. Many people underestimate the complexity involved in preparing and curating a dataset for training AI models.
- Training AI models requires a solid understanding of machine learning techniques.
- Data annotation is a time-consuming task that requires attention to detail.
- Creating accurate and diverse datasets can be challenging.
Misconception 2: AI can perfectly generalize from a limited set of images
Another misconception is that AI can generalize well from a limited set of images. While AI models can learn patterns and make predictions based on the training data, they may struggle when confronted with images that are significantly different from what they were trained on. This limitation is especially pronounced when models are trained on a small and biased dataset.
- AI models need diverse datasets to improve their ability to generalize.
- Training on a limited set of images can result in overfitting, where the model performs poorly on new images.
- The performance of AI models heavily depends on the quality and diversity of the training data.
Misconception 3: AI can accurately capture complex concepts from images
Many people believe that AI can easily understand and capture complex concepts from images. However, AI models struggle with abstract or subjective concepts that require human interpretation. While AI can recognize objects and patterns, it often fails to grasp the intricate meanings and nuances conveyed by images.
- AI models have limitations when it comes to understanding abstract or subjective concepts.
- Interpretation of context and intent in images is challenging for AI models.
- Human involvement is necessary for labeling and interpreting complex concepts in images.
Misconception 4: AI training is a one-time process
Another misconception is that AI training is a one-time process. In reality, AI models require continuous training and fine-tuning to adapt to changing conditions and improve their performance. The initial training is just the beginning, and ongoing updates are needed to ensure the AI model remains accurate and effective.
- AI models benefit from continuous training with new data to stay up-to-date.
- Regular re-evaluation and re-training are necessary to maintain high performance.
- Improvement of AI models is an iterative process that requires continuous effort.
Misconception 5: AI training with personal images guarantees privacy protection
Many people believe that training AI with personal images guarantees the protection of their privacy. However, this is not always the case. Training AI models often involves uploading and storing personal images on servers, which can pose privacy risks if not handled properly. It is crucial to ensure that proper security measures and data protection protocols are in place when training AI models.
- Companies and individuals should prioritize data privacy when training AI models with personal images.
- Personal images should be encrypted and stored securely to safeguard privacy.
- Transparency and consent are essential when handling personal data for AI training.
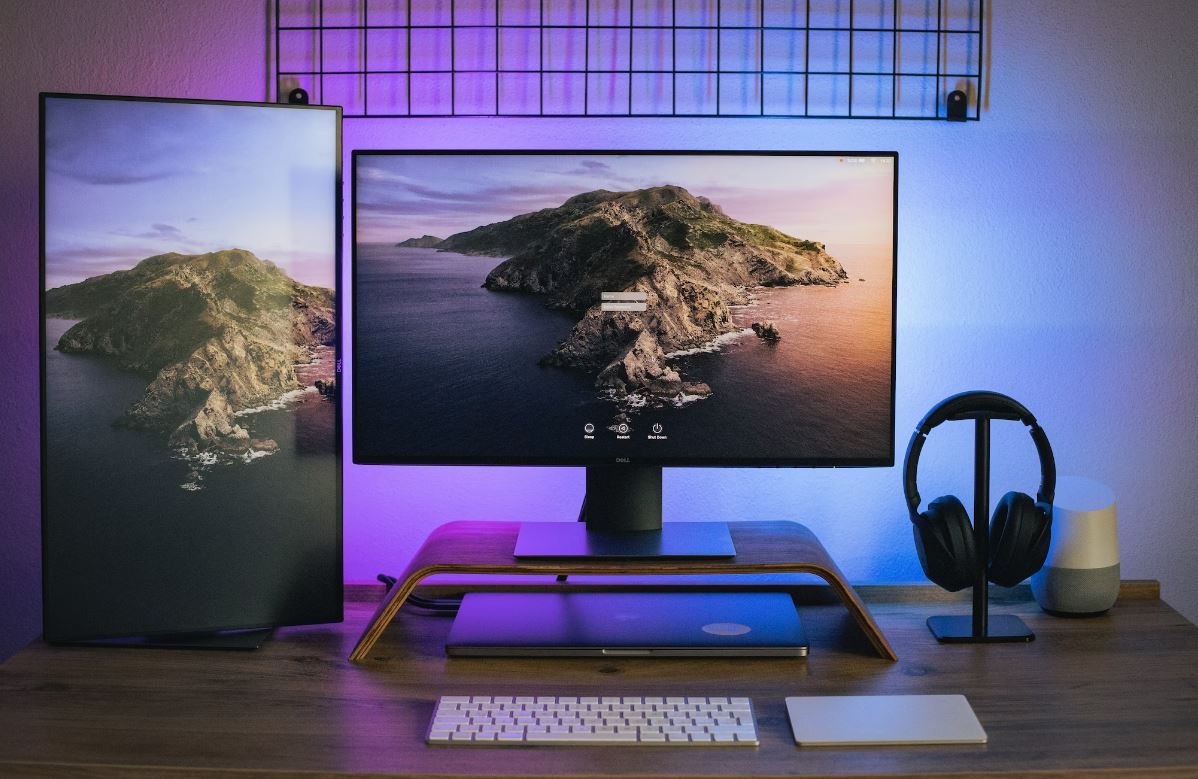
Train AI with Your Own Images
Your Daily Coffee Consumption
As coffee lovers, it is interesting to see how much coffee people in different countries consume on a daily basis. The table below shows the average daily coffee consumption in selected countries.
Country | Average Daily Coffee Consumption (in cups) |
---|---|
Finland | 4.2 |
Netherlands | 2.4 |
Canada | 2.3 |
Germany | 2.1 |
United States | 1.9 |
Smartphone Market Share
The smartphone industry is highly competitive, with various companies vying for market dominance. The table presents the global market share of leading smartphone brands in 2021.
Brand | Market Share (%) |
---|---|
Samsung | 22.2 |
Apple | 16.6 |
Xiaomi | 11.8 |
Oppo | 10.9 |
Huawei | 8.4 |
Population of Major Cities
Urbanization is rapidly changing the world, with more people flocking to major cities. Here are five of the world’s most populated cities, along with their estimated populations.
City | Population |
---|---|
Tokyo, Japan | 37.4 million |
Delhi, India | 31.4 million |
Shanghai, China | 27.1 million |
São Paulo, Brazil | 21.7 million |
Mexico City, Mexico | 21 million |
Tallest Buildings in the World
Skyscrapers are architectural marvels that showcase human achievement. The table below lists the five tallest buildings in the world along with their heights in meters.
Building | Height (m) |
---|---|
Burj Khalifa, Dubai | 828 |
Shanghai Tower, China | 632 |
Abraj Al-Bait Clock Tower, Mecca | 601 |
Ping An Finance Center, China | 599 |
Lotte World Tower, South Korea | 555 |
World Cup Winners
The FIFA World Cup is one of the most prestigious sporting events. Here are the previous winners of the tournament, showcasing the dominance of certain countries.
Country | Number of World Cup Wins |
---|---|
Brazil | 5 |
Germany | 4 |
Italy | 4 |
Argentina | 2 |
Uruguay | 2 |
Top 5 Richest People
Wealth distribution varies greatly around the globe. Below are the five individuals who top the list of the world’s richest people based on their net worth.
Name | Net Worth (USD) |
---|---|
Jeff Bezos | 203 billion |
Elon Musk | 197 billion |
Bernard Arnault & family | 185 billion |
Bill Gates | 136 billion |
Mark Zuckerberg | 121 billion |
Life Expectancy by Country
The average life expectancy is an important indicator of a country’s healthcare and living conditions. Here are the average life expectancies of selected countries.
Country | Average Life Expectancy (years) |
---|---|
Japan | 84.6 |
Switzerland | 83.8 |
Australia | 83.3 |
Spain | 82.9 |
Canada | 82.5 |
Electric Vehicle Market Share
As the world shifts towards sustainable transportation, electric vehicles are gaining popularity. This table presents the market share of electric vehicles across different regions.
Region | Electric Vehicle Market Share (%) |
---|---|
Norway | 54.3 |
Iceland | 33.8 |
Sweden | 29.6 |
Netherlands | 19.9 |
China | 8.6 |
Global Internet Users
The internet has transformed the way we connect and access information. Here are the estimated number of internet users in selected regions of the world.
Region | Number of Internet Users (in millions) |
---|---|
Asia | 2,582 |
Europe | 727 |
North America | 367 |
Latin America | 453 |
Africa | 525 |
Conclusion
Training AI with your own images is an engaging way to explore the capabilities of artificial intelligence. From analyzing coffee consumption patterns to understanding market trends and societal indicators, AI is reshaping our world. The tables presented here demonstrate how data can be organized effectively to provide insightful information. Through AI, we can unlock endless possibilities and make data-driven decisions to shape a better future.
Train AI with Your Own Images
Frequently Asked Questions
How does image training for AI work?
Image training for AI involves feeding a machine learning model with a large dataset of images along with their corresponding labels or categories. The model then learns patterns and features within these images and uses this knowledge to classify or recognize similar images in the future.
What types of AI models can be trained with images?
A wide range of AI models can be trained with images, including image recognition models, object detection models, facial recognition models, and image segmentation models, to name a few. The choice of model depends on the specific application or use case.
What are the prerequisites for training AI with images?
To train AI with images, you typically need a large dataset of annotated images, sufficient computing resources, and knowledge of machine learning techniques. Additionally, familiarity with programming languages such as Python and frameworks like TensorFlow or PyTorch can be beneficial.
How do I collect a dataset of images for AI training?
You can collect a dataset of images for AI training by sourcing images from public datasets, scraping images from the web, or capturing images using cameras or other devices. It is essential to ensure that your dataset covers a wide range of variations and accurately represents the target domain.
What are the best practices for labeling images in AI training?
Some best practices for labeling images in AI training include using consistent and descriptive labels, ensuring accurate and comprehensive annotation, and incorporating quality control measures to minimize labeling errors. It is also crucial to maintain a balance between data diversity and data quantity.
How long does it typically take to train an AI model with images?
The training time for an AI model with images varies depending on factors such as the complexity of the model architecture, the size of the dataset, and the available computing resources. Training can range from a few hours to several days or even weeks for more intensive models.
What hardware is required to train AI models with images?
Training AI models with images often requires high-performance hardware, such as GPUs (Graphics Processing Units) or TPUs (Tensor Processing Units). These hardware accelerators can significantly speed up the training process by performing parallel computations on large matrices.
Can pre-trained AI models be fine-tuned with new images?
Yes, pre-trained AI models can be fine-tuned with new images to adapt them to specific tasks or domains. By leveraging transfer learning techniques, you can take advantage of the knowledge learned by existing models trained on large datasets and apply it to your own image training tasks.
What are some common challenges in training AI models with images?
Common challenges in training AI models with images include overfitting (when a model performs well on the training data but poorly on new data), lack of diverse and representative data, computational limitations, and choosing the appropriate model architecture and hyperparameters.
How can I evaluate the performance of my AI model trained with images?
The performance of an AI model trained with images can be evaluated using metrics such as accuracy, precision, recall, F1 score, and confusion matrix. Cross-validation techniques can also help assess the model’s robustness and generalization ability.