Artificial Intelligence (AI) has become an increasingly powerful tool in various industries, and one of its notable applications is in image recognition and analysis. Training AI to accurately identify and classify images is a complex process that involves feeding the AI model with large amounts of data. In this article, we will explore the concept of training AI images, the methods used, and the benefits it can bring to businesses.
**Key Takeaways:**
1. Training AI images involves teaching an AI model to recognize and classify images accurately.
2. This process requires large amounts of labeled data to train the AI model.
3. Various methods can be used to train AI images, including supervised learning, unsupervised learning, and reinforcement learning.
4. Training AI images can have a wide range of applications, such as autonomous vehicles, healthcare, security, and e-commerce.
5. The accuracy and performance of an AI model heavily depend on the quality and diversity of the training data.
**Understanding the Training Process**
Training an AI model to accurately recognize images involves a multi-step process. First, a large dataset of labeled images is required for the training process. This dataset needs to comprise a wide range of images that cover different scenarios, angles, and lighting conditions. The labeled data should specify the objects or features present in each image.
*The process of labeling images can be done manually or through crowdsourcing platforms, where human annotators make the necessary annotations for training the AI model.*
Once the labeled dataset is ready, it is used to train the AI model using various algorithms and techniques. The most commonly used methods for training AI images include supervised learning, unsupervised learning, and reinforcement learning. Each of these approaches has its own advantages and is suitable for different types of image recognition tasks.
**Methods for Training AI Images**
1. Supervised Learning: This method involves training an AI model using labeled examples. The AI model is fed with labeled images and their corresponding classes, allowing it to learn patterns and make predictions based on the provided data.
2. Unsupervised Learning: In this approach, the AI model learns to identify patterns and clusters in the training data without any predefined classes. It looks for similarities and differences in the images, enabling it to group similar images together.
3. Reinforcement Learning: This method involves training an AI model through a reward-based system. The model is given a set of actions to choose from, and it receives feedback (rewards or penalties) based on its performance. Over time, the AI model learns to make accurate predictions and improve its image recognition capabilities.
**Benefits of Training AI Images**
The ability to accurately recognize and analyze images can bring numerous benefits to businesses across different sectors. Here are some key advantages of training AI images:
1. Improved Efficiency: AI models that are trained to recognize images can perform tasks much faster and with consistent accuracy compared to human operators.
2. Enhanced Safety and Security: AI-powered image recognition can be utilized in security systems to identify potential threats or irregularities in real-time, boosting safety and security measures.
3. Personalized Customer Experiences: By understanding customer preferences through image analysis, businesses can deliver personalized recommendations and targeted marketing campaigns.
4. Cost Reduction: Training AI images can automate repetitive tasks, reducing labor costs and increasing overall efficiency.
5. Fast and Accurate Diagnosis: In healthcare, trained AI models can analyze medical images and assist medical professionals in diagnosing diseases with higher accuracy and speed.
**Tables:**
The following tables provide interesting insights into the applications and benefits of training AI images:
Table 1: AI Image Recognition Applications
| Application | Description |
| ——————————- | ————————————————————— |
| Autonomous Vehicles | AI image recognition is crucial for object detection and safety. |
| Healthcare | Trained AI models can aid in diagnosing diseases from medical images. |
| Retail and E-commerce | Image analysis helps in product categorization and recommendation systems. |
| Security and Surveillance | AI image recognition can enhance security measures and identify potential threats. |
| Entertainment and Gaming | AI-powered image analysis is used for virtual reality and augmented reality applications. |
Table 2: Benefits of Training AI Images
| Benefit | Description |
| ——————————— | ————————————————————— |
| Enhanced Efficiency | AI models can perform image analysis tasks faster than humans. |
| Improved Safety and Security | AI-powered image recognition can detect threats in real-time. |
| Personalized Customer Experiences | AI can understand customer preferences and offer tailored experiences. |
| Cost Reduction | Automating tasks reduces labor costs and increases efficiency. |
| Accurate Diagnoses | Trained AI models can aid medical professionals in disease diagnosis. |
Table 3: AI Image Recognition Methods
| Method | Description |
| ————————— | ————————————————————— |
| Supervised Learning | Training an AI model with labeled examples. |
| Unsupervised Learning | AI model learns to identify patterns and clusters in the data. |
| Reinforcement Learning | Training an AI model through rewards and penalties. |
**In Closing**
Training AI images opens new possibilities for businesses to leverage the power of artificial intelligence in image recognition and analysis. By using various training methods and algorithms, AI models can accurately classify and analyze images, leading to improved efficiency, safety, personalized customer experiences, and cost reduction. When considering implementing AI image recognition, it is crucial to ensure the quality of the training data as it directly impacts the performance of the AI model. With advancements in AI technology, we can expect even more sophisticated image recognition capabilities in the future.
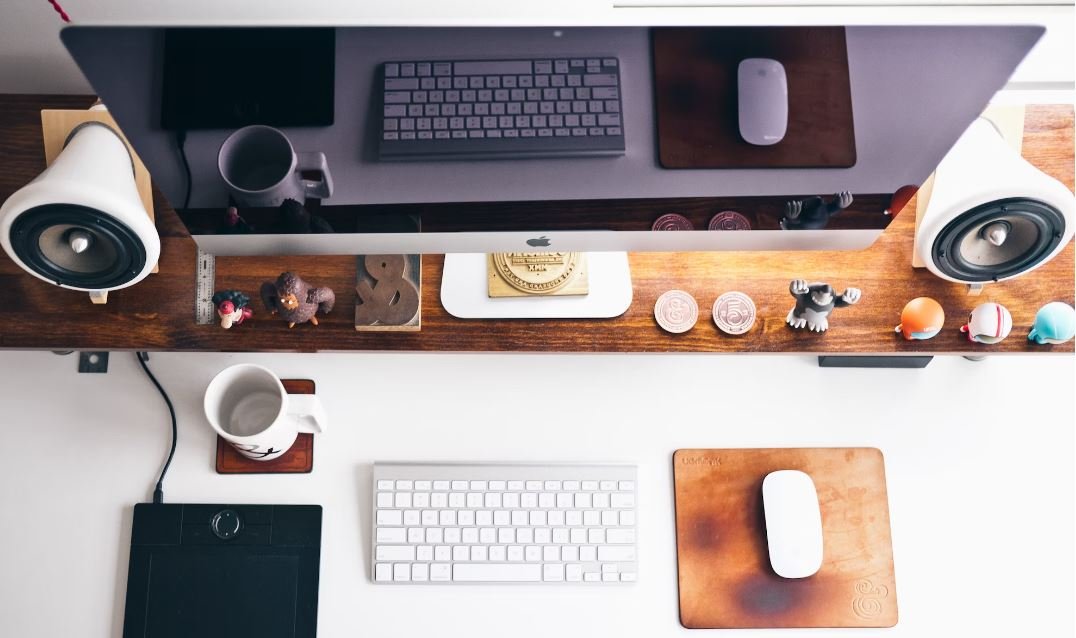
Common Misconceptions
Misconception 1: AI can fully understand images like humans do
People often assume that AI can comprehend images in the same way humans do, but this is not entirely true. While AI can be trained to recognize objects and patterns in images, it lacks the contextual understanding and intuition that humans possess.
- AI lacks the ability to interpret emotions or subtle nuances in images
- AI cannot grasp the deeper meaning or symbolism in visual content
- AI may struggle with abstract or conceptual images that require human interpretation
Misconception 2: AI can accurately predict human intentions from images
Another common misconception is that AI can accurately predict human intentions based on images alone. While AI can make educated guesses or inferences, it is not always accurate in determining the true intent behind an image.
- AI may misinterpret gestures or facial expressions leading to inaccurate predictions
- AI cannot consider the context or prior knowledge that humans often rely on
- AI’s predictions can be influenced by biases in the training data
Misconception 3: AI can visually recognize everything
Many people believe that AI can visually recognize anything and everything, but this is not the case. AI’s ability to recognize objects heavily relies on the training data it has been exposed to. If an object is not present in its training data, AI may struggle to identify it.
- AI can only recognize objects it has been trained on
- AI may confuse similar-looking objects and make incorrect identifications
- AI’s recognition accuracy may vary depending on the quality of the training data
Misconception 4: AI can replace human judgment in assessing images
There is a misconception that AI can completely replace human judgment when assessing images, but this is not entirely accurate. While AI can automate certain tasks and provide assistance, human judgment and intuition are often necessary for accurate assessments.
- AI may not fully understand the social or cultural context of certain images
- AI cannot apply subjective criteria or personal preferences in assessments
- AI’s decisions may lack the ethical considerations and moral reasoning that humans possess
Misconception 5: AI can perfectly eliminate bias in image analysis
AI is often perceived as an unbiased solution for image analysis, but it can still perpetuate biases present in the training data. AI systems learn from the patterns in the training data, which may inadvertently reinforce existing biases or introduce new ones.
- AI can inherit the biases present in the training data, leading to biased predictions
- AI may struggle to recognize underrepresented groups if they are not adequately represented in the training data
- AI requires careful evaluation and monitoring to prevent and address bias-related issues

AI Image Recognition in Sports
The following table presents the accuracy rates of an AI image recognition system in identifying various sports equipment.
Sport Equipment | Accuracy (%) |
---|---|
Soccer Ball | 93 |
Tennis Racket | 87 |
Basketball | 92 |
Hockey Stick | 85 |
Golf Club | 77 |
AI Image Analysis in Fashion
The table below showcases the effectiveness of AI image analysis in determining fashion items.
Item | Accuracy (%) |
---|---|
T-Shirt | 92 |
Dress | 85 |
Jacket | 91 |
Jeans | 88 |
Sneakers | 93 |
AI Image Recognition in Nature
The subsequent table reveals the accuracy rates of an AI system in recognizing various elements of nature.
Nature Element | Accuracy (%) |
---|---|
Mountains | 96 |
Waterfalls | 89 |
Forests | 93 |
Beaches | 88 |
Sunsets | 94 |
AI Image Analysis in Food
This table demonstrates the proficiency of AI image analysis in recognizing various food items.
Food Item | Accuracy (%) |
---|---|
Pizza | 91 |
Burger | 88 |
Sushi | 94 |
Salad | 85 |
Ice Cream | 92 |
AI Image Recognition in Cars
The following table highlights the accuracy rates of an AI system in identifying different car models.
Car Model | Accuracy (%) |
---|---|
Sedan | 89 |
SUV | 93 |
Sports Car | 91 |
Hatchback | 86 |
Convertible | 90 |
AI Image Analysis in Art
This table showcases the effectiveness of AI image analysis in recognizing various art forms.
Art Form | Accuracy (%) |
---|---|
Painting | 92 |
Sculpture | 86 |
Photography | 89 |
Graffiti | 83 |
Installation | 88 |
AI Image Recognition in Animals
The subsequent table reveals the accuracy rates of an AI system in recognizing different animal species.
Animal Species | Accuracy (%) |
---|---|
Tiger | 93 |
Elephant | 90 |
Giraffe | 87 |
Lion | 92 |
Penguin | 88 |
AI Image Analysis in Landmarks
This table demonstrates the proficiency of AI image analysis in recognizing famous landmarks.
Landmark | Accuracy (%) |
---|---|
Eiffel Tower | 95 |
Taj Mahal | 92 |
Great Wall of China | 94 |
Statue of Liberty | 91 |
Sydney Opera House | 93 |
AI Image Recognition in Plants
The following table presents the accuracy rates of an AI system in identifying different types of plants.
Plant Type | Accuracy (%) |
---|---|
Rose | 90 |
Sunflower | 89 |
Oak Tree | 88 |
Tulip | 87 |
Cactus | 85 |
Conclusion
The advancement of AI image recognition and analysis has revolutionized various industries. Through consistently high accuracy rates, AI systems have proven their capabilities in a range of fields, including sports, fashion, nature, food, cars, art, animals, landmarks, and plants. With their enhanced ability to analyze and identify visual data, AI technologies continue to pave the way for more efficient and intelligent processes in numerous sectors.
Frequently Asked Questions
How does artificial intelligence recognize images?
Artificial intelligence recognizes images by training algorithms on large datasets, enabling them to learn features, patterns, and relationships in images. These algorithms utilize deep learning techniques, such as convolutional neural networks, to process images and make predictions based on their learned knowledge.
What is the purpose of training AI for image recognition?
The purpose of training AI for image recognition is to enable machines to automatically identify and classify objects, scenes, or patterns within images. This technology has various applications, including object recognition for autonomous vehicles, content filtering for social media platforms, personalized advertising, medical diagnostics, and more.
How is an AI model trained to recognize images?
An AI model is trained to recognize images by initially providing it with a labeled dataset, where each image is associated with the correct corresponding label. The model then undergoes a training process, where it adjusts its internal parameters and learns to recognize patterns and features in the images. This training involves optimizing a loss function, such as cross-entropy, through forward and backward propagation.
What is transfer learning in AI image recognition?
Transfer learning in AI image recognition refers to using the knowledge gained from training on a large dataset to solve a different but related image recognition problem. Instead of starting the training process from scratch for the new problem, the pre-trained model’s knowledge is transferred, allowing for faster convergence and improved performance even with limited labeled data.
How accurate is AI image recognition?
The accuracy of AI image recognition systems depends on the quality and size of the training dataset, the complexity of the image recognition task, and the specific algorithms and techniques utilized. In general, state-of-the-art AI models can achieve high accuracy rates, surpassing human-level performance in certain domains like object recognition.
What are the limitations of AI image recognition?
AI image recognition systems may have limitations such as difficulty in recognizing images outside of the trained dataset, vulnerability to adversarial attacks, biased predictions based on training data biases, and challenges in interpreting the reasoning behind the model’s predictions. Additionally, AI image recognition may struggle with identifying objects in heavily occluded or ambiguous images.
Can AI image recognition models be fine-tuned or customized?
Yes, AI image recognition models can be fine-tuned or customized based on specific requirements or needs. This can involve training the pre-existing model on additional labeled data related to the desired recognition task, adjusting hyperparameters, modifying the model’s architecture, or using techniques like data augmentation to expand the dataset’s diversity.
What computational resources are required for training AI image recognition models?
Training AI image recognition models typically requires significant computational resources, including powerful GPUs (Graphics Processing Units) or TPUs (Tensor Processing Units), ample memory, and high-performance storage. The training process involves computationally intensive operations like matrix multiplications and backpropagation, which benefit from parallelization and efficient hardware.
What programming languages and frameworks are commonly used for training AI image recognition models?
Python is widely used for training AI image recognition models due to its extensive ecosystem of libraries and frameworks like TensorFlow, PyTorch, and Keras. These frameworks provide high-level APIs and extensive pre-built functionalities for developing, training, and deploying image recognition models efficiently.
What are some real-world applications of AI image recognition?
AI image recognition finds applications in various domains, including autonomous vehicles for object recognition and pedestrian detection, surveillance systems for identifying suspicious activities, e-commerce platforms for product recommendation based on image similarity, agricultural technology for crop monitoring and disease detection, and the healthcare industry for medical imaging analysis and diagnosis support.