Best AI for Research
Artificial Intelligence (AI) has made significant advancements in various fields, including research. The ability of AI to process vast amounts of data and extract meaningful insights has revolutionized the way researchers work. In this article, we will explore the best AI tools and platforms that researchers can utilize to enhance their work.
Key Takeaways
- AI has transformed the research process by enabling efficient data analysis.
- Researchers can leverage AI tools to automate repetitive tasks and increase productivity.
- AI-powered recommendation systems enhance the accuracy and relevance of research findings.
Leveraging AI for Research
One of the key advantages of using AI for research is its ability to quickly analyze large datasets, saving researchers substantial time. Tools like **Google Scholar** and **Semantic Scholar** employ machine learning algorithms to extract relevant information from academic papers and provide researchers with comprehensive literature reviews. These tools also offer AI-based citation analysis, enabling researchers to track the impact and importance of their own work.
AI can also aid in automating repetitive research tasks, such as data collection and organization. Services like **Import.io** and **Webhose.io** utilize AI algorithms to scrape information from websites and provide researchers with structured datasets. This automation streamlines the research workflow and allows researchers to focus on higher-value tasks.
*AI has the potential to revolutionize the way researchers work, eliminating the need to spend hours on manual data collection and literature reviews.*
AI-Powered Recommendation Systems
Another valuable application of AI in research is the use of recommendation systems. Platforms like **ArXiv Sanity Preserver** and **Sparrho** utilize AI algorithms to analyze research articles and provide personalized recommendations based on user interests. These systems consider contextual information such as the user’s previous searches and the relevance of articles to their field of study, improving the efficiency and accuracy of literature discovery.
*AI-powered recommendation systems can help researchers discover relevant papers and stay up-to-date with the latest research in their field.*
AI in Research Data Analysis
AI is also transforming data analysis in research. Tools like **IBM Watson Analytics** and **Microsoft Azure Machine Learning Studio** allow researchers to analyze complex datasets and extract meaningful insights. These platforms offer a range of AI algorithms, such as clustering and classification, which can help researchers uncover patterns and trends within their data.
Platform | Features |
---|---|
IBM Watson Analytics |
|
Microsoft Azure Machine Learning Studio |
|
*AI-based data analysis tools enable researchers to make data-driven decisions and gain deeper insights from their research.*
The Future of AI in Research
As AI continues to advance, we can expect further integration of AI technologies in research. AI’s ability to provide personalized recommendations, automate repetitive tasks, and process large datasets efficiently will revolutionize the way researchers work.
Furthermore, the integration of AI and Natural Language Processing (NLP) will enable AI systems to understand and analyze research articles more effectively, providing researchers with even more accurate and relevant information.
Benefits of AI in Research |
---|
|
*The future of AI in research holds incredible potential for accelerating scientific progress and expanding human knowledge.*
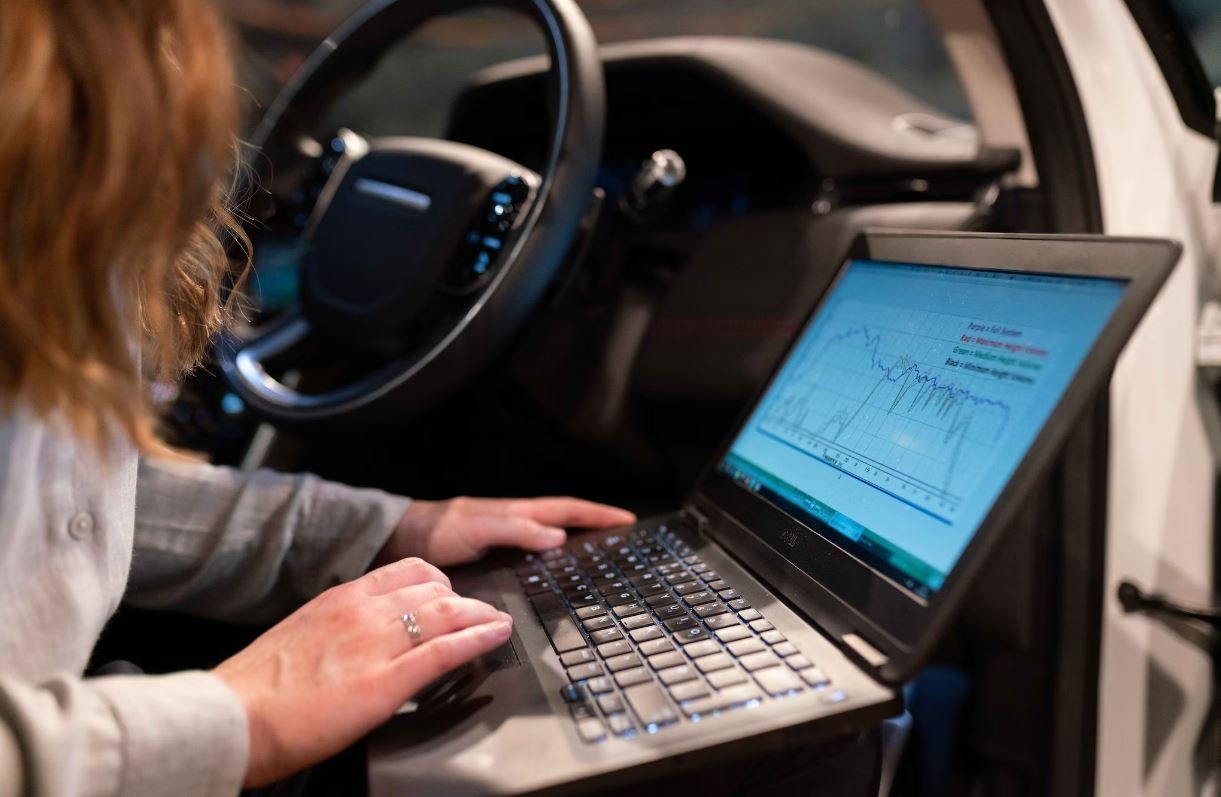
Common Misconceptions
Misconception 1: The best AI for research can replace human researchers entirely
One common misconception surrounding AI in research is that it has the potential to completely replace human researchers. While AI has undoubtedly revolutionized the field of research in many ways, it is important to understand its limitations. AI cannot replicate certain human qualities such as creativity, intuition, and critical thinking, which are crucial for research.
- AI is a tool that can assist human researchers in analyzing data.
- Human researchers provide unique insights and context that AI cannot replicate.
- The combination of AI and human researchers can lead to more innovative and comprehensive research outcomes.
Misconception 2: The best AI for research is always the most advanced and complex
Another common misconception is that the best AI for research is always the most advanced and complex one. While advanced AI models can provide valuable insights, they are not always necessary or appropriate for every research project. Simpler AI models can often suffice and yield meaningful results, depending on the research objectives and data available.
- The complexity of AI should be tailored to the specific research needs.
- Simpler AI models can be more interpretable and easier to validate.
- Choosing the right AI model requires considering the research question and available resources.
Misconception 3: The best AI for research is always the most accurate
There is a common misconception that the best AI for research is always the most accurate one. While accuracy is undoubtedly important, it is not the sole determining factor in assessing the quality of AI for research. Other factors such as interpretability, scalability, and ethical considerations also play a significant role in choosing the most suitable AI for research purposes.
- Interpretability of AI models can help researchers understand and trust the results.
- Scalability is important for handling large datasets and processing time-sensitive research.
- Ethical considerations, such as bias and fairness, should be evaluated alongside accuracy.
Misconception 4: The best AI for research can analyze any type of data
Many people have the misconception that the best AI for research can analyze any type of data, regardless of its format or quality. However, not all AI models are suitable for all types of data. Different AI algorithms are designed to handle specific types of data, such as text, images, or numerical data. It is essential to choose an AI model that is specifically trained for the type of data being analyzed.
- AI models excel in analyzing specific types of data, based on their training and architecture.
- Choosing the right AI model involves considering the format and characteristics of the data.
- Inappropriate use of AI models can lead to inaccurate or misleading results.
Misconception 5: The best AI for research is unbiased
A common misconception is that the best AI for research is inherently unbiased. AI models are trained on historical data, which can contain biases present in society. If these biases are not properly addressed and mitigated during the training process, AI can perpetuate and even amplify existing biases. It is important to carefully evaluate and ensure the fairness and ethical implications of the AI model used in research.
- AI models can inadvertently perpetuate biases present in historical data.
- Evaluating and addressing biases is essential for ensuring fairness in research outcomes.
- Ethical considerations should be incorporated throughout all stages of AI application in research.
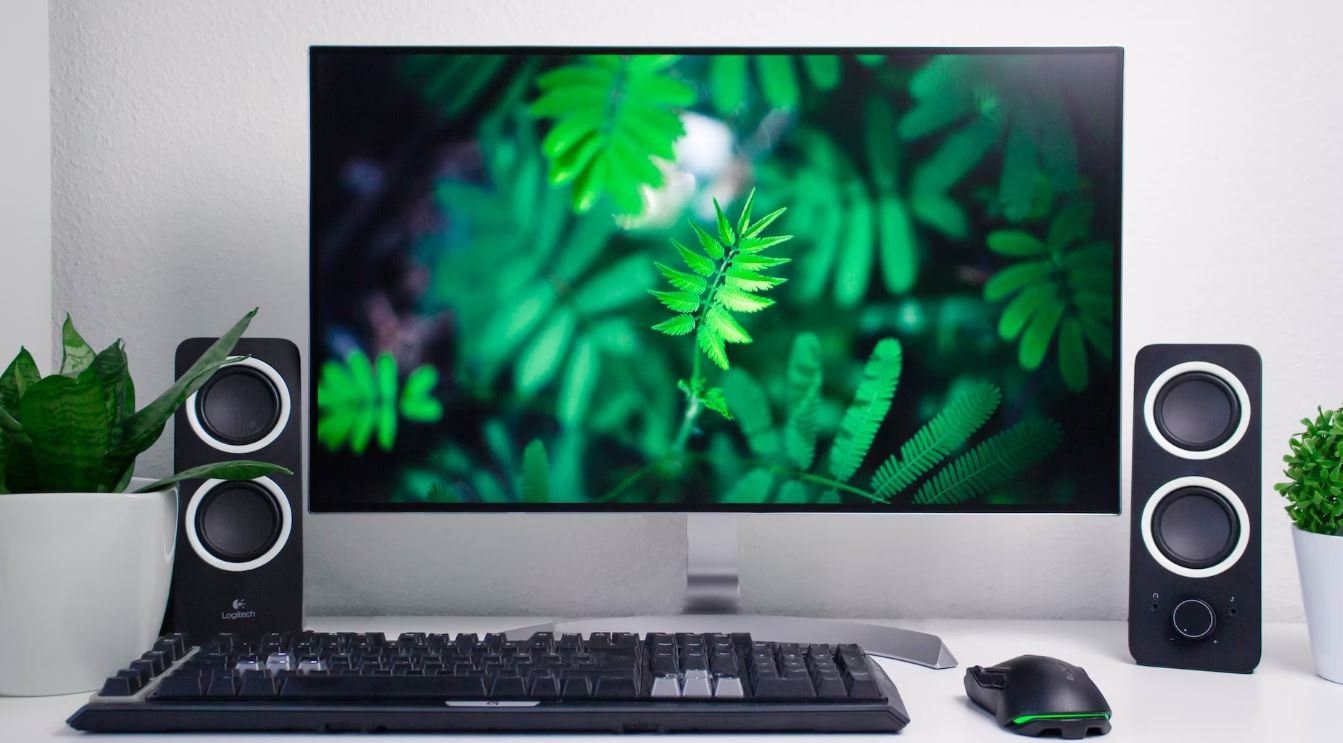
Introduction
Artificial intelligence (AI) has revolutionized the field of research, providing powerful tools and techniques to enhance productivity and drive discoveries. In this article, we explore ten fascinating aspects that demonstrate how AI is contributing to advancements in research across various domains.
Table: Impact of AI in Medical Research
AI in medical research has revolutionized diagnostics, drug discovery, and patient care. This table highlights some remarkable achievements in the field:
Aspect | Impact |
---|---|
Disease Diagnosis | AI systems have shown high accuracy in diagnosing diseases like cancer and heart conditions. |
Drug Discovery | AI algorithms enable the identification of potential drug candidates more efficiently, accelerating the development process. |
Personalized Medicine | AI assists in tailoring treatments based on an individual’s genetic makeup and medical history, improving patient outcomes. |
Table: AI Applications in Climate Change Research
Climate change research is pivotal to understanding the environment and developing strategies to mitigate its impact. AI plays a crucial role in these endeavors, as demonstrated in the following table:
Application | Benefit |
---|---|
Climate Modeling | AI-powered models offer more accurate predictions of climate patterns, aiding in future climate change scenarios. |
Extreme Weather Detection | AI algorithms analyze vast amounts of data to identify patterns associated with extreme weather events, improving early warning systems. |
Sustainability Solutions | AI helps optimize resource utilization and energy efficiency, facilitating sustainable practices. |
Table: AI in Astrophysics Research
Astrophysics relies on AI to process massive amounts of data and unveil profound insights into the mysteries of the universe. Explained more in detail below:
Research Area | AI Contribution |
---|---|
Galaxy Classification | AI algorithms analyze images to classify galaxies more accurately, aiding in understanding cosmic evolution. |
Exoplanet Detection | AI assists in processing data from telescopes to identify exoplanets, expanding our knowledge of distant planetary systems. |
Dark Matter Studies | AI algorithms help analyze large-scale datasets to investigate the nature of dark matter, a major cosmic mystery. |
Table: AI-Driven Discoveries in Archaeological Research
AI applications have transformed archaeological research, aiding in the preservation and interpretation of historical artifacts. The following table highlights some notable achievements:
Discoveries | AI Contribution |
---|---|
Artifact Restoration | AI algorithms aid in reconstructing damaged artifacts and preserving cultural heritage. |
Language Decipherment | AI models facilitate the understanding of ancient languages, assisting in translating ancient texts. |
Hidden Structure Detection | Using AI, researchers can detect hidden structures or features within archaeological sites, revealing new insights. |
Table: AI in Social Science Research
Social science research benefits from AI applications that can analyze vast amounts of social data to extract meaningful patterns and insights:
Research Domain | AI Application |
---|---|
Sentiment Analysis | AI-powered sentiment analysis enables researchers to analyze public opinions on various social issues. |
Behavioral Modeling | AI algorithms simulate complex human behaviors, aiding in the study of social dynamics and decision-making processes. |
Demographic Prediction | AI models can predict demographic changes and population trends based on available data. |
Table: AI-Enhanced Environmental Research
AI revolutionizes environmental research by providing innovative solutions to address ecological challenges. Explore the following table for some intriguing examples:
Application | AI Impact |
---|---|
Ecosystem Monitoring | AI technologies monitor ecosystems in real-time, aiding in the preservation of biodiversity. |
Water Quality Assessment | AI algorithms analyze water samples, detecting pollutants and ensuring water safety. |
Wildlife Conservation | AI-assisted image recognition enables efficient identification and tracking of endangered species. |
Table: AI in Physics Research
AI complements physics research by assisting in various experiments and data analysis tasks. The table below presents key applications of AI in the field:
Application | AI Contribution |
---|---|
Particle Detection | AI algorithms identify and analyze particle collisions, aiding in the exploration of fundamental particles. |
Quantum Computing | AI techniques support the development of quantum computing algorithms, unlocking new computational capabilities. |
Simulation Optimization | AI helps optimize complex simulations, reducing computational time and improving accuracy. |
Table: AI Applications in Agricultural Research
Agricultural research leverages AI to increase crop yield, improve sustainability, and address global food security challenges. The following table exemplifies AI’s impact on agricultural practices:
Application | Benefit |
---|---|
Precision Farming | AI-driven sensors and drones enable precise mapping, irrigation, and fertilization, optimizing resource utilization. |
Pest Control | AI-based pest detection systems aid in early identification of crop threats, reducing the need for harmful pesticides. |
Crop Disease Diagnosis | AI models analyze plant characteristics to diagnose diseases accurately, facilitating prompt treatment and prevention. |
Table: AI-Driven Advances in Material Science Research
Material science research benefits immensely from AI-powered simulations and data analysis, resulting in the development of innovative materials with unique properties:
Research Area | AI Contribution |
---|---|
Drug Discovery | AI models predict molecular interactions, accelerating drug discovery and eliminating costly experimental trials. |
Material Design | AI algorithms help design materials with desired structural and functional properties, enhancing efficiency across industries. |
Nanomaterial Characterization | AI-based image analysis enables precise characterization of nanomaterials, aiding in their development for various applications. |
Conclusion
Artificial intelligence has transformed the research landscape across diverse disciplines, enabling advancements that were once unimaginable. From medical breakthroughs to environmental conservation, AI’s contribution is evident. As technology continues to evolve, AI will undoubtedly play an increasingly crucial role in scientific discoveries and innovation, driving us towards a more informed and prosperous future.
Best AI for Research – Frequently Asked Questions
What is artificial intelligence (AI)?
Artificial intelligence (AI) refers to the simulation of human intelligence in machines that are programmed to think, reason, and learn. AI technology allows computers to perform tasks that typically require human intelligence, such as problem-solving, decision-making, and natural language processing.
How can AI be used for research purposes?
AI can be utilized in research to analyze vast amounts of data, identify patterns, and make predictions or recommendations based on the findings. It can automate processes, support data collection and analysis, enhance decision-making, and contribute to scientific advancements in various fields.
What are the criteria for selecting the best AI for research?
The criteria for selecting the best AI for research may vary depending on the specific requirements of the research project. However, some important factors to consider might include the AI’s performance, accuracy, scalability, computational efficiency, interpretability of results, available features, ease of integration, and compatibility with existing research tools and systems.
What are some popular AI technologies used in research?
Some popular AI technologies used in research include machine learning, deep learning, natural language processing (NLP), computer vision, robotics, and expert systems. These technologies enable researchers to analyze data, gain insights, automate tasks, and develop intelligent systems that can assist in various research domains.
How can AI improve the accuracy and efficiency of research studies?
By harnessing AI technologies, researchers can automate repetitive tasks, handle large datasets, detect complex patterns or anomalies, eliminate human errors, and gain valuable insights from data more efficiently. AI algorithms can process and analyze data at a speed and scale that surpass human capabilities, leading to improved accuracy and increased research productivity.
What are the potential challenges of using AI in research?
Some challenges of using AI in research include the need for high-quality labeled data for training, potential bias in algorithmic decision-making, interpretability and explainability of AI models, limited availability of domain-specific AI solutions, ethical considerations related to privacy and data usage, and the requirement for skilled AI experts to develop and maintain AI systems.
Are there any ethical concerns associated with AI in research?
Yes, ethical concerns related to AI in research exist. These concerns may include issues such as data privacy, potential biases in AI algorithms, informed consent for the usage of personal data, transparency and accountability of AI systems, and the impact of AI on jobs and societal structures. Researchers should be attentive to these ethical considerations when utilizing AI in their studies.
Are there any AI tools specifically designed for research purposes?
Yes, there are AI tools that are specifically designed to support research activities. Some examples include data analysis platforms with built-in AI capabilities, research assistant chatbots that can provide relevant information and suggestions, AI-powered literature review tools for efficient literature search, and AI-based predictive models for various research domains.
How can I evaluate the effectiveness of an AI tool for research?
Evaluating the effectiveness of an AI tool for research involves assessing its performance against the intended research objectives. This can be done by benchmarking the tool’s accuracy, comparing it to alternative solutions, evaluating its impact on research outcomes, and seeking feedback from other researchers who have used the same or similar tools. Additionally, considering the tool’s usability, reliability, and user reviews can also help in the evaluation process.
Where can I find AI resources and support for research purposes?
There are various resources available for researchers seeking AI support. These resources include online communities, forums, and discussion groups where researchers can share knowledge and seek assistance. Additionally, universities, research institutions, and AI companies often provide workshops, training programs, and consultation services for researchers interested in incorporating AI into their projects.