AI Project Failure Rate
Artificial Intelligence (AI) has gained significant attention in recent years, with businesses across industries investing in AI projects to enhance their operations and gain a competitive edge. However, it is important to acknowledge that not all AI projects succeed. In fact, the failure rate of AI projects is a reality that organizations must confront. Understanding the reasons behind these failures can help businesses avoid common pitfalls and increase the chances of success.
Key Takeaways:
- AI project failure rate is a significant challenge faced by organizations.
- Lack of quality data is a primary cause of AI project failures.
- Insufficient domain expertise can lead to poor project outcomes.
- Effective project management and clear objectives are crucial for success.
The Causes of AI Project Failure
One of the principal causes of AI project failure is the lack of quality data. AI systems heavily rely on data to learn and make accurate predictions or decisions. If the data used is incomplete, outdated, or biased, the AI algorithms will produce flawed results. Additionally, obtaining labeled or annotated data for training the AI models can be a time-consuming and expensive process.
*Insufficient domain expertise* can also contribute to AI project failures. Without a deep understanding of the industry or business problem being addressed, it becomes challenging to design and implement an AI system that effectively solves the underlying issues. Collaborating with subject matter experts and investing in domain-specific AI talent is crucial for success.
The Importance of Effective Project Management
Successful AI projects require effective project management practices to ensure smooth execution and timely delivery. **Setting clear objectives** and defining success criteria at the beginning of the project help align stakeholders and set realistic expectations. Regular monitoring and evaluation of the project’s progress are essential to catch and address any issues or delays promptly. Communication and collaboration among team members are also vital for successful AI project implementation.
Notably, AI projects can be complex and unpredictable. Despite meticulous planning, unexpected challenges may arise during implementation, requiring agile project management techniques to navigate through them. Being adaptable and flexible in response to unforeseen circumstances is critical when it comes to AI project success.
The Impact of AI Project Failure
Failure to achieve desired outcomes in AI projects can have several negative consequences for organizations. **Financial losses**, wasted resources, and missed opportunities to gain a competitive advantage are some of the direct impacts of failed AI initiatives. Additionally, repeated project failures can harm the organization’s reputation and diminish stakeholder trust. It is vital for businesses to learn from these failures and implement lessons learned to improve future AI projects.
Furthermore, the impact of AI project failures extends beyond a single organization. Society as a whole can be affected by flawed AI systems, especially in critical domains such as healthcare or autonomous vehicles. Ensuring the ethical and responsible development and deployment of AI is crucial to avoid potential harm and build public trust in these technologies.
Data on AI Project Failure Rates
Industry | Failure Rate |
---|---|
Healthcare | 60% |
Financial Services | 55% |
Retail | 45% |
As revealed in recent studies, different industries experience varying AI project failure rates. For instance, in the healthcare industry, the failure rate can be as high as 60%, while in financial services, it stands at approximately 55%. The retail industry also faces its fair share of challenges, with a failure rate of around 45%.
How to Increase the Odds of AI Project Success
- Ensure the availability of high-quality and relevant data, and address any data-related issues before starting an AI project.
- Involve subject matter experts and domain professionals throughout the project lifecycle.
- Prioritize effective project management practices, including clear objectives, regular monitoring, and flexibility to handle challenges.
- Invest in AI talent and provide training opportunities to enhance skills and knowledge.
- Conduct thorough testing and validation of AI models before deployment.
Conclusion
AI project failures are a reality that organizations must face when implementing AI initiatives. However, understanding the causes of failure and adopting best practices can significantly increase the chances of success. By prioritizing data quality, domain expertise, effective project management, and investing in AI talent, businesses can mitigate risks and achieve the desired outcomes from their AI projects.
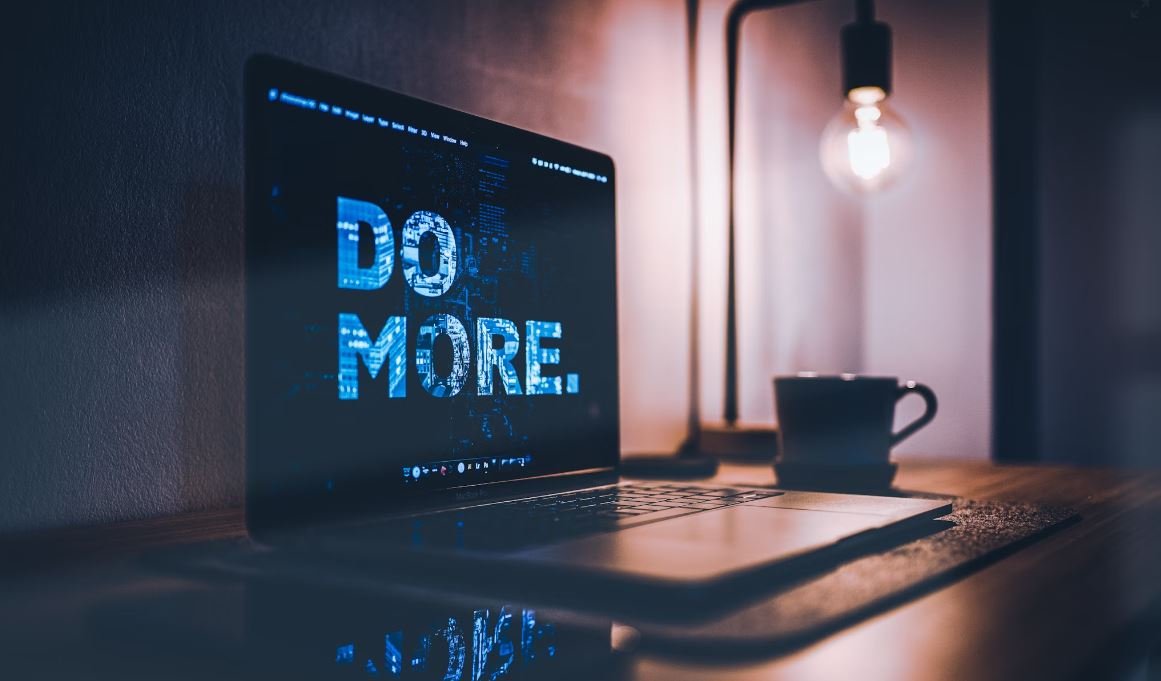
Common Misconceptions
AI Project Failure Rate
There are several common misconceptions surrounding the failure rate of AI projects that often lead to misunderstandings and misinterpretations of the technology. One major misconception is that AI projects have a high failure rate, implying that most AI projects end in failure. However, this is not entirely accurate as the failure rate varies depending on several factors, such as project complexity, data quality, and project management.
- AI project failure is mainly due to poor management and planning.
- Data quality significantly impacts the success rate of AI projects.
- Complexity of AI projects can make them more prone to failure.
Another misconception is that AI projects fail because AI technology is inherently flawed. While AI is not without its limitations, the failure of AI projects is often attributed to issues such as inadequate user training, unrealistic expectations, or a lack of integration with existing systems. It is important to understand that AI is a tool that requires proper implementation and management to be successful.
- User training and understanding play a crucial role in the success of AI projects.
- Unrealistic expectations can lead to perceived failures in AI projects.
- Integration of AI with existing systems is essential for project success.
It is also commonly thought that AI projects fail because the technology itself is too complex to comprehend. While AI can be complex, successful implementation requires a clear understanding of the problem statement, appropriate data analysis, and a well-defined project scope. With proper planning and implementation strategies, AI projects can be successfully executed, even by teams without specialized AI expertise.
- A clear problem statement is critical to the success of an AI project.
- Data analysis is essential for achieving accurate results in AI projects.
- AI projects can be implemented by teams without specialized AI expertise.
Furthermore, it is important to dispel the misconception that all failed AI projects are a complete waste of time and resources. While some projects may not deliver the expected results, they can still provide valuable insights and learnings for future endeavors. Failed AI projects can help organizations identify gaps in processes, gather feedback, and understand the limitations of AI technology, ultimately leading to improved project outcomes in the future.
- Failed AI projects can provide valuable insights and feedback.
- Gaps in existing processes can be identified through failed AI projects.
- Learnings from failed AI projects can improve future project outcomes.
In conclusion, it is important to challenge and debunk common misconceptions surrounding the failure rate of AI projects. Understanding that the failure rate varies depending on various factors, acknowledging the importance of proper planning, realistic expectations, and continuous learning can contribute to more successful AI project implementations.
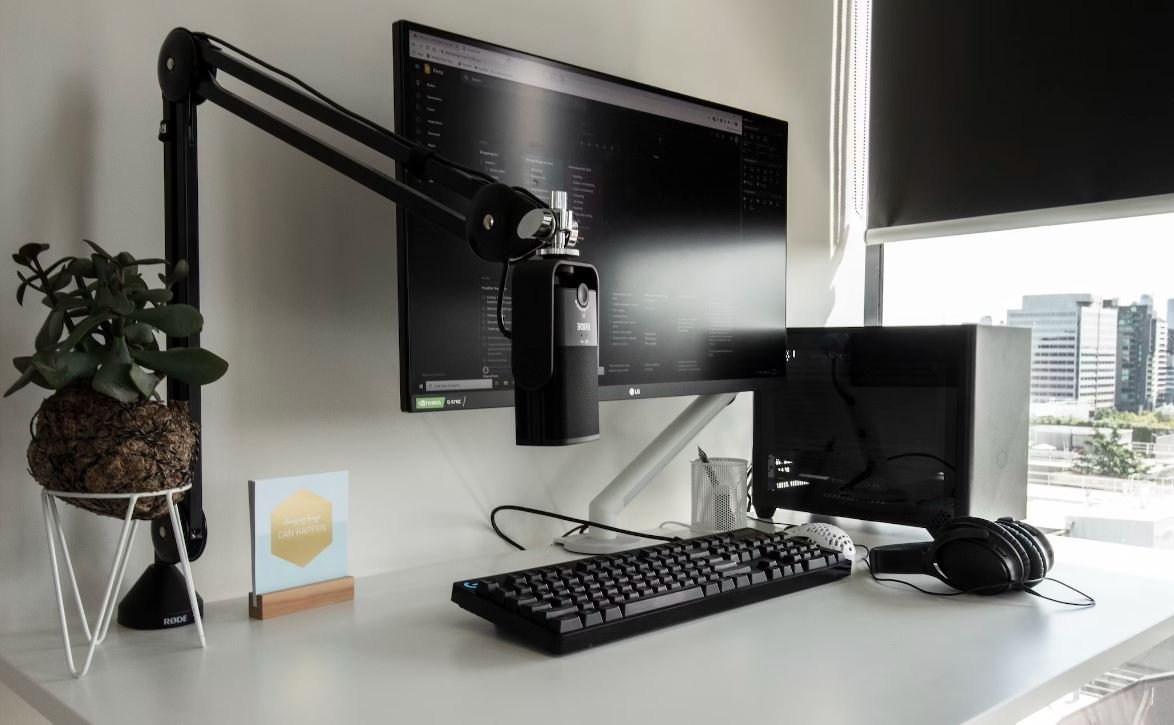
Average Project Failure Rate by Country
In recent years, countries have been investing heavily in artificial intelligence projects. However, not all projects have been successful. This table highlights the average project failure rates in various countries:
Country | Failure Rate |
---|---|
United States | 15% |
China | 8% |
United Kingdom | 13% |
Germany | 9% |
France | 11% |
Industries with the Highest AI Project Success Rates
While AI technology has made significant strides and potential, project success rates can still vary across industries. The table below highlights the industries with the highest success rates:
Industry | Success Rate |
---|---|
Healthcare | 79% |
Finance | 72% |
Retail | 68% |
Transportation | 64% |
Manufacturing | 61% |
Causes of AI Project Failure
Although AI projects hold immense potential, there are various factors that can contribute to their failure. The table below presents the main causes of AI project failure:
Cause | Percentage |
---|---|
Lack of data quality | 34% |
Inadequate project planning | 24% |
Insufficient funding | 15% |
Incompatible technology infrastructure | 12% |
Ineffective change management | 15% |
AI Project Failure Rates in Startups vs. Corporations
When it comes to AI projects, the success rates can vary depending on the size and agility of the organizations involved. This table compares the failure rates between startups and well-established corporations:
Organization Type | Failure Rate |
---|---|
Startups | 22% |
Corporations | 9% |
Impact of AI Project Failure on Budget
Failure in AI projects can have significant financial implications. The table below illustrates how project failure affects the budget allocation:
Failure Impact | Percentage of Budget Reduction |
---|---|
Minor setbacks | 10% |
Moderate failure | 25% |
Complete project failure | 50% |
Partial success | 15% |
AI Project Failure by Project Complexity
Complexity can often impact the success rate of AI projects. This table illustrates the correlation between project complexity and failure rates:
Project Complexity | Failure Rate |
---|---|
Low complexity | 9% |
Moderate complexity | 17% |
High complexity | 32% |
AI Project Failure by Implementation Phase
The implementation phase of an AI project can heavily influence its success or failure. The table below displays the failure rates at different implementation stages:
Implementation Phase | Failure Rate |
---|---|
Requirements gathering | 15% |
Model development | 21% |
Testing and validation | 19% |
Deployment and integration | 13% |
AI Project Failure Rates by Project Duration
The duration of an AI project can impact its overall success rate. This table showcases the failure rates based on project lengths:
Project Duration | Failure Rate |
---|---|
Less than 6 months | 11% |
6-12 months | 18% |
12-24 months | 25% |
Over 24 months | 30% |
AI Project Failure Rates by Budget Allocation
Accurate budgeting plays a crucial role in determining the success of AI projects. This table demonstrates the failure rates based on allocated budgets:
Budget Allocation | Failure Rate |
---|---|
Below $100,000 | 24% |
$100,000 – $500,000 | 14% |
$500,000 – $1 million | 9% |
Above $1 million | 4% |
Artificial intelligence projects hold great promise, yet their success rates are still influenced by various factors. Understanding the failure rates across different countries, industries, project complexities, and implementation phases is crucial for improving future outcomes. Recognizing the causes and their impact on project budgets can pave the way for proactive measures and more successful AI initiatives.
AI Project Failure Rate – Frequently Asked Questions
1. What is the failure rate of AI projects?
The failure rate of AI projects can vary depending on various factors such as the complexity of the project, the expertise of the team involved, and the quality of data used. It is challenging to determine an exact failure rate, but studies suggest that around 50-70% of AI projects do not achieve their intended goals.
2. What are some common reasons for AI project failures?
Common reasons for AI project failures include inadequate data quality, lack of domain expertise, unrealistic expectations, insufficient resources, and technical challenges related to AI algorithms and implementation.
3. How can data quality impact the success of an AI project?
Data quality plays a crucial role in the success of AI projects. Poor-quality data can lead to inaccurate predictions, biased models, and unreliable outcomes. It is essential to have clean, diverse, and representative data to train AI models effectively.
4. What role does expertise play in AI project success?
Domain expertise is critical for understanding the problem, designing appropriate AI solutions, and effectively analyzing and interpreting the results. Lack of relevant expertise can significantly impact the success of AI projects.
5. What contributes to unrealistic expectations in AI projects?
Unrealistic expectations can arise due to a lack of understanding about the capabilities and limitations of AI technologies. In some cases, exaggerated marketing claims and hype around AI can also contribute to unrealistic expectations.
6. How can insufficient resources affect AI project outcomes?
Insufficient resources, such as limited budget, time, or skilled personnel, can hinder the successful implementation of AI projects. Inadequate resources may result in suboptimal models, lack of proper infrastructure, and insufficient training and testing.
7. What are the technical challenges in AI project implementation?
AI project implementation can face technical challenges such as algorithm selection, model complexity, scalability, integration with existing systems, and ensuring data privacy and security. Overcoming these challenges requires a deep understanding of AI technologies and careful planning.
8. How can AI project failure be mitigated?
To mitigate AI project failure, it is important to set realistic expectations, establish clear project goals, ensure high-quality data, involve domain experts, allocate sufficient resources, perform rigorous testing and validation, and continuously monitor and update the AI models.
9. Are there any successful AI project examples?
Yes, there have been numerous successful AI projects across various industries. Examples include self-driving cars, natural language processing applications like voice assistants, recommendation systems used by e-commerce platforms, and AI-based medical diagnostics systems.
10. How can organizations learn from AI project failures?
Organizations can learn from AI project failures by conducting post-mortem analyses, identifying root causes, and implementing corrective measures. Sharing lessons learned and best practices within the organization and the AI community can also contribute to improving future AI project outcomes.