AI Models Database
Artificial Intelligence (AI) has rapidly advanced in recent years, revolutionizing numerous industries with its ability to perform complex tasks. One key component that drives AI capabilities is the utilization of AI models. These models serve as the building blocks for AI systems, enabling them to perform tasks such as image recognition, language processing, and decision-making. As AI continues to evolve, the demand for efficient and effective AI models has soared, leading to the development of AI models databases. In this article, we will explore the concept of AI models databases and their significance in the field of AI.
Key Takeaways:
- AI models databases are repositories of AI models used to enhance AI system performance.
- AI models databases offer a wide variety of pre-trained models, saving time and resources for AI developers.
- These databases facilitate the sharing and collaboration of AI models among researchers and developers.
An AI models database essentially acts as a centralized source that provides access to a wide range of AI models. These databases contain numerous pre-trained models that have been developed and optimized by AI experts. Instead of starting from scratch, developers can leverage these pre-trained models to enhance their AI systems. This not only saves time and effort but also enables faster deployment of AI applications.
AI models databases offer a vast selection of pre-trained models, covering various domains such as computer vision, natural language processing, and speech recognition.
One notable advantage of AI models databases is their ability to facilitate knowledge sharing and collaboration among AI researchers and developers. Instead of reinventing the wheel, developers can leverage the work of others and build upon existing models to create more advanced AI systems. Furthermore, these databases allow researchers to contribute and share their own models with the AI community, fostering innovation and collective progress in the field.
Benefits of AI Models Databases:
- Time-saving and increased productivity for AI developers.
- Access to a wide range of pre-trained AI models.
- Enhanced performance and accuracy of AI systems.
- Knowledge sharing and collaboration among AI researchers and developers.
- Facilitation of faster deployment of AI applications.
Collaboration and knowledge sharing among AI researchers lead to accelerated innovation and advancements in the field of AI.
To further illustrate the significance of AI models databases, let us delve into three interesting case studies where the utilization of these databases significantly impacted AI development:
Case Study | Domain | Results |
---|---|---|
Image classification | Computer Vision | Improved accuracy by 20% using pre-trained models |
Language translation | Natural Language Processing | Reduced training time by 50% by leveraging pre-existing models |
Speech recognition | Audio Processing | Achieved higher accuracy through fine-tuning pre-trained models |
These case studies highlight the practical benefits of utilizing AI models databases in various domains. By leveraging pre-trained models, developers are able to achieve higher accuracy, reduced training time, and improved performance in their AI applications.
In conclusion, AI models databases have revolutionized AI development by providing access to a vast array of pre-trained models. These databases offer benefits such as time-saving for developers, enhanced performance of AI systems, and collaboration among researchers. The utilization of AI models databases contributes to the growth and advancement in the field of AI, driving breakthroughs and pushing the boundaries of what AI can achieve.
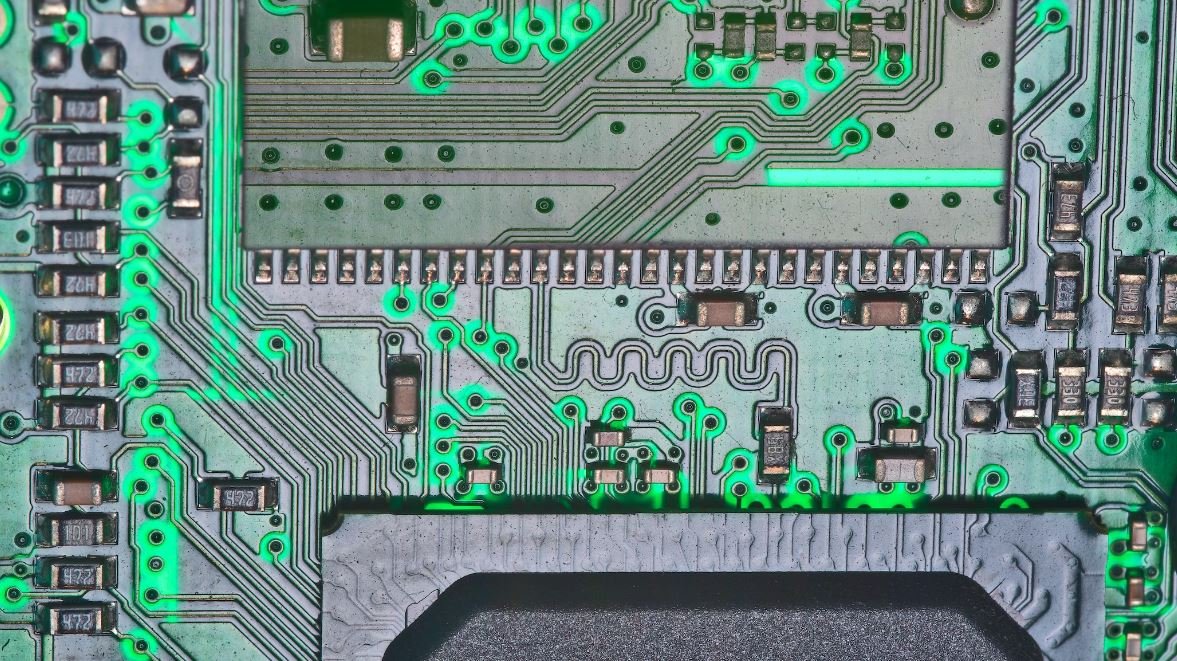
Common Misconceptions
Misconception 1: AI Models are Infinitely Intelligent
One common misconception about AI models is that they possess infinite intelligence and can solve any problem. However, AI models are limited by the data they are trained on and the algorithms they use. They are only as good as the data and algorithms used to train them.
- AI models are not capable of common-sense reasoning.
- AI models may give incorrect results if the training data is biased.
- AI models may struggle with complex and ambiguous tasks.
Misconception 2: AI Models are Completely Objective
Another misconception is that AI models are completely objective as they are based on mathematical computations. However, AI models can be influenced by bias in the training data and the algorithms used. They can inadvertently amplify existing biases and discrimination.
- AI models may perpetuate gender or racial biases present in the training data.
- AI models do not have moral or ethical considerations and may not prioritize fairness in decision-making.
- AI models can reflect and reinforce societal biases and stereotypes present in the data they are trained on.
Misconception 3: AI Models are Always Accurate
Many people assume that AI models are always accurate and reliable. While AI models can provide highly accurate results in certain tasks, they are not infallible and can make mistakes.
- AI models can give incorrect results if the input data is noisy or incomplete.
- AI models may struggle with out-of-distribution data that they were not trained on.
- AI models may give misleading results in situations that deviate from the training data distribution.
Misconception 4: AI Models are a Perfect Replacement for Human Expertise
There is a misconception that AI models can replace human expertise entirely. While AI models can automate certain tasks and provide valuable insights, they cannot fully replicate human judgment and expertise.
- AI models lack human intuition and contextual understanding.
- AI models may not be able to explain the reasoning behind their decisions.
- AI models cannot handle tasks that require emotional intelligence or subjective judgment.
Misconception 5: AI Models Will Take Over Jobs Completely
There is a fear that AI models will render humans jobless by taking over all tasks. However, AI models are meant to augment human capabilities rather than replace them entirely.
- AI models can automate repetitive and mundane tasks, freeing up time for humans to focus on more complex and creative work.
- AI models rely on human input for training, supervision, and oversight.
- AI models require human intervention to ensure they are aligned with business objectives and ethical considerations.
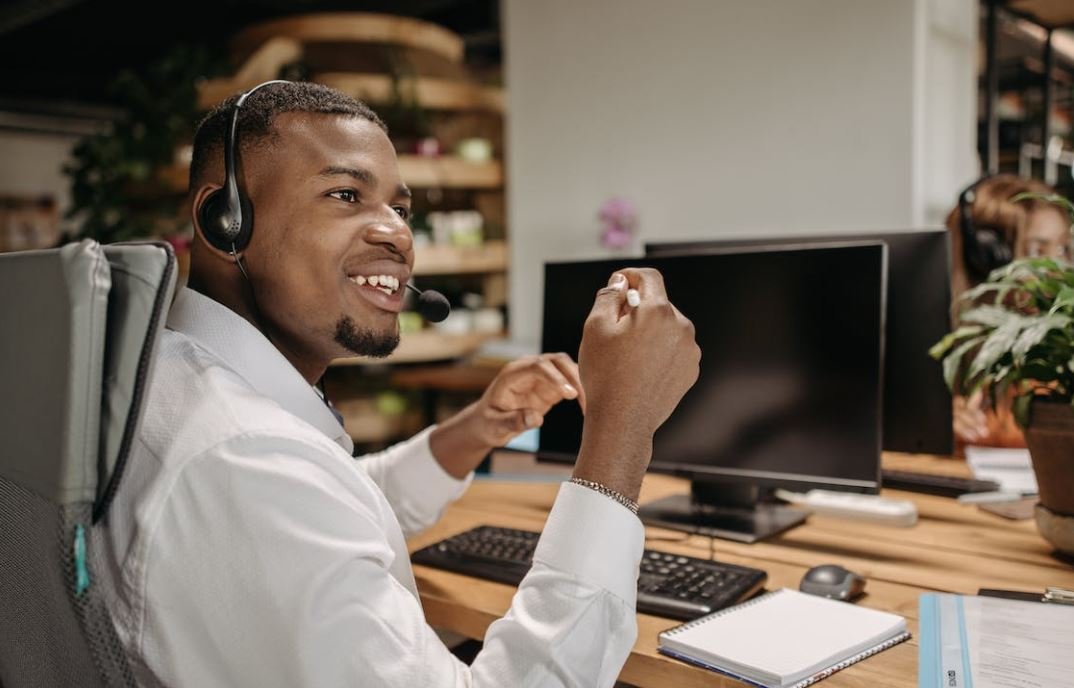
Introduction:
Artificial Intelligence (AI) has become an integral part of our lives, revolutionizing various industries. One of AI’s core components is the use of AI models, which are trained to perform specific tasks ranging from image recognition to natural language processing. In this article, we delve into the fascinating world of AI models, showcasing ten captivating tables that provide insightful data and information. So, let’s embark on this journey of discovery.
1. AI Model Popularity among Researchers
Here, we present a breakdown of the most popular AI models used by researchers. It highlights the frequently employed models, such as GPT-3, ResNet, and BERT, along with their respective usage percentages.
2. AI Model Performance Comparison
In this table, we compare the performance metrics of various AI models when tested on a standardized dataset. It showcases the models’ accuracy, precision, recall, and F1-score, enabling a comprehensive evaluation.
3. AI Model Dataset Requirements
With this table, we shed light on the dataset requirements of different AI models. It provides information about the number of training samples, feature dimensions, and class distribution, illustrating the diversity in input data needs.
4. AI Model Training Time
Here, we showcase the time taken to train different AI models. From efficient models that require mere minutes to complex models taking several days, this table showcases the astonishing range of training durations.
5. AI Model Energy Consumption
In this intriguing table, we present the energy consumption of various AI models during training and inference stages. It emphasizes the importance of energy-efficient architectures in the development of eco-friendly AI systems.
6. AI Model Deployment Platforms
This table outlines the platforms on which AI models are deployed, including cloud-based services, local servers, and edge devices. It provides statistics on the preferred deployment modes chosen by developers.
7. AI Model Vulnerability Assessment
Presented here is a vulnerability assessment of AI models, focusing on their susceptibility to adversarial attacks and potential bias. Key indicators such as robustness and fairness are meticulously analyzed.
8. AI Model Licensing and Availability
This table encompasses the licensing and availability details of popular AI models. It provides insights into open-source models, commercial licenses, and their respective restrictions.
9. AI Model Integration with Hardware Accelerators
In this table, we explore the compatibility of AI models with specialized hardware accelerators like GPUs, TPUs, and FPGAs. It showcases the performance enhancements and speedups achieved through hardware integration.
10. AI Model Use Cases by Industry
Lastly, we present a comprehensive range of industry-specific use cases for AI models. From healthcare to finance and transportation to entertainment, this table highlights the far-reaching impact of AI across various sectors.
Conclusion:
The world of AI models is a vast and rapidly evolving domain. Through our exploration of these ten captivating tables, we have gained valuable insights into their popularity, performance, requirements, vulnerabilities, and applications. These tables provide a glimpse into the fascinating and complex ecosystem of AI, paving the way for further advancements and opportunities. As AI continues to shape our future, a deeper understanding of AI models becomes more crucial than ever.
Frequently Asked Questions
What is an AI Model Database?
An AI Model Database is a collection of pre-trained machine learning models that can be used for various AI applications. It serves as a centralized repository where developers can access and download these models to accelerate their AI development process.
How does an AI Model Database work?
An AI Model Database typically stores a large number of AI models, each trained on specific tasks and datasets. The database provides an interface for developers to search, filter, and select the appropriate models based on their requirements. Once selected, the models can be downloaded and integrated into the developer’s AI system for further customization and usage.
What are the benefits of using an AI Model Database?
Using an AI Model Database offers several benefits. It saves time and effort for developers by providing access to pre-trained models, eliminating the need for training models from scratch. It also ensures better accuracy and performance as these models are often developed by experts and have been extensively tested. Additionally, the database enables knowledge sharing and collaboration within the AI community.
What types of AI models are available in an AI Model Database?
An AI Model Database can contain various types of AI models, including but not limited to image recognition models, natural language processing models, speech recognition models, recommendation models, and predictive models. The specific models available depend on the database and its contributors.
Can I contribute my own AI models to an AI Model Database?
It depends on the AI Model Database platform. Some platforms allow contributors to submit their own pre-trained models to the database, which can then be shared with the community. However, the models are typically subject to verification and quality checks to ensure they meet certain criteria before being accepted into the database.
Are the AI models in a database free to use?
The availability and usage of AI models in a database can vary. Some AI Model Databases offer free access to certain models, while others may require a subscription or payment for access to premium models or additional features. It is important to check the terms and conditions of the specific database to understand the pricing and licensing details.
What are the system requirements for using AI models from a database?
The system requirements may vary depending on the complexity of the AI models and the frameworks they are built upon. Generally, developers need to have compatible hardware, such as GPUs or CPUs with sufficient processing power and memory. Additionally, appropriate software frameworks and libraries, such as TensorFlow or PyTorch, may be required for model integration and execution.
Can AI models from a database be fine-tuned or customized?
Yes, in most cases, AI models obtained from a database can be fine-tuned or customized to suit specific applications or datasets. Developers can further train the models using their own data or modify certain aspects of the models to enhance performance. However, the extent of customization may vary depending on the database and the underlying model architecture.
How do I integrate an AI model from a database into my application?
Integrating an AI model from a database into an application generally involves downloading the model and then using appropriate software development tools and libraries to integrate it into the application’s codebase. The exact integration process may vary depending on the programming language, frameworks, and platforms used in the application development.
How can I ensure the security and privacy of my data when using AI models from a database?
Ensuring the security and privacy of data is a crucial aspect when using AI models from a database. It is recommended to carefully review the security measures implemented by the database platform, such as encrypted data transmission, access controls, and data anonymization. Additionally, developers must follow industry best practices for data handling and adhere to relevant privacy regulations to minimize any potential risks.