AI Model to Train
Artificial Intelligence (AI) has revolutionized various industries, including technology, healthcare, finance, and more. One essential component of AI is the ability to train models effectively, allowing machines to learn and make accurate predictions. In this article, we will explore an AI model to train and its implications for future advancements.
Key Takeaways
- An AI model enables machines to learn and make predictions.
- Efficient training techniques are crucial for the accuracy of AI models.
- AI model training plays a vital role in numerous industries.
**Training an AI model** involves feeding it with large amounts of data and allowing it to learn patterns and relationships through various algorithms. By iteratively adjusting these algorithms, the model improves its performance over time, making increasingly accurate predictions.
*An AI model acts as a digital brain, constantly analyzing and adapting to the data it receives.*
**To achieve effective AI model training**, several techniques and considerations come into play. Firstly, selecting the appropriate data sets and ensuring data quality significantly influence the model’s accuracy. Secondly, implementing robust algorithms and optimization techniques can speed up the training process. Finally, the computational resources available largely impact the model’s scalability and performance.
Benefits of AI Model Training
AI model training offers several benefits, making it an indispensable tool in various sectors. Some notable advantages include:
- Improved efficiency and accuracy in complex tasks.
- Automated decision-making processes based on data analysis.
- Enhanced diagnostic capabilities in healthcare.
- Effective fraud detection and prevention in finance.
AI Model Training Process
The AI model training process involves several key steps:
**1. Data preparation**: Collecting and cleaning the data to ensure its reliability and relevance.
**2. Model selection**: Choosing the appropriate model architecture based on the specific problem and data characteristics.
**3. Hyperparameter tuning**: Adjusting the model’s parameters to optimize performance and prevent overfitting.
**4. Training**: Feeding the data into the model and allowing it to iteratively learn and improve predictions.
**5. Evaluation**: Assessing the trained model’s performance through various metrics.
**6. Deployment**: Implementing the trained model into a production environment for real-world applications.
Tables
Algorithm | Pros | Cons |
---|---|---|
Gradient Descent | – Efficient for large datasets | – May get stuck in local optima |
Stochastic Gradient Descent | – Fast convergence | – Prone to noise interference |
Adam Optimization | – Adaptive learning rates | – Higher memory usage |
Model | Accuracy | Processing Speed |
---|---|---|
Model A | 92% | 200 ms |
Model B | 95% | 150 ms |
Model C | 98% | 230 ms |
Resource | GPU | CPU |
---|---|---|
Memory Usage | High | Low |
Processing Power | High | Medium |
*Implementing the right training algorithm and model architecture significantly impact the model’s performance and accuracy.*
Future Outlook
The continuous advancements in AI model training techniques hold immense potential for further development and improvement across various domains. As computing power continues to increase, coupled with ongoing research efforts, we can anticipate even more accurate and efficient AI models in the future.
AI model training will continue to play a crucial role in driving innovation and solving complex problems in numerous industries, powering the next generation of AI-driven technologies.
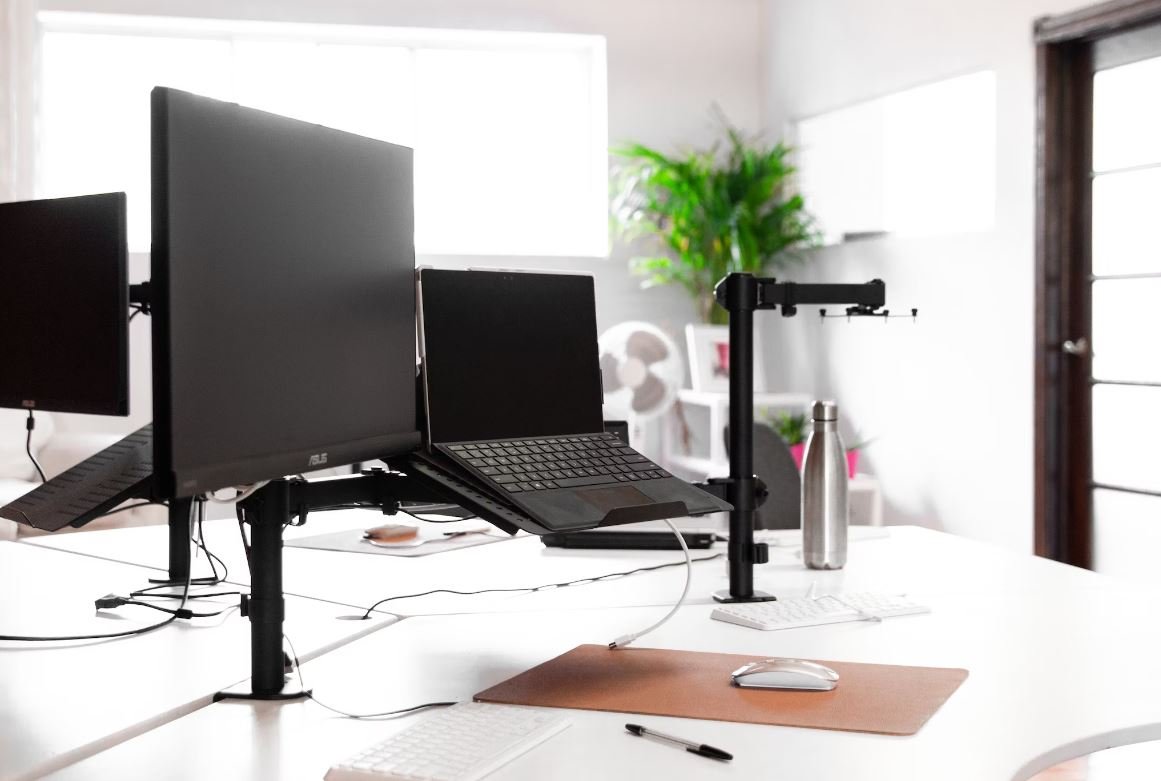
Common Misconceptions
Misconception 1: AI models can think and act like humans
One of the most common misconceptions about AI models is that they can think and act like humans. While AI models can process vast amounts of data and perform complex tasks, they are still limited by their programming and lack human consciousness.
- AI models operate based on algorithms and do not possess consciousness.
- They can only perform tasks they are programmed for and cannot think or reason.
- AI models lack emotions and cannot experience human-like sentiments.
Misconception 2: AI models are infallible
Another misconception is that AI models are infallible and always produce accurate results. While AI models are capable of learning from data and improving their performance, they are prone to errors and biases, just like any other technology.
- AI models can produce inaccurate results if the training data is biased or inadequate.
- They can also be vulnerable to adversarial attacks, where malicious input can manipulate their outputs.
- AI models require continuous monitoring and validation to ensure their accuracy and reliability.
Misconception 3: AI models will replace human jobs entirely
There is a widespread belief that AI models will replace human jobs entirely, leading to massive unemployment. While AI technology can automate certain tasks, it is unlikely to completely replace human labor in most industries.
- AI models are best suited for repetitive and mundane tasks that can be easily automated.
- They can augment human capabilities by automating routine tasks, freeing up time for more complex and creative work.
- AI models often require human supervision and intervention, especially in critical decision-making processes.
Misconception 4: AI models are universally intelligent
AI models are often believed to be universally intelligent and capable of excelling in various domains. However, AI models are typically designed for specific tasks and lack general intelligence.
- AI models are trained and optimized for specific tasks, such as image recognition or natural language processing.
- They struggle with tasks outside their training domain and often require substantial retraining.
- AI models may not possess common-sense reasoning abilities and can exhibit unexpected behavior in unfamiliar situations.
Misconception 5: AI models are always expensive to develop and deploy
Many people assume that developing and deploying AI models is always an expensive endeavor. While sophisticated AI models can indeed require substantial investment, there are also various open-source tools and platforms that make AI more accessible.
- There are numerous open-source libraries and frameworks available for developing AI models at a low cost.
- Cloud-based AI services allow businesses to use AI models without the need for extensive infrastructure or resources.
- AI models can be trained using existing data and resources, reducing the upfront costs of development.
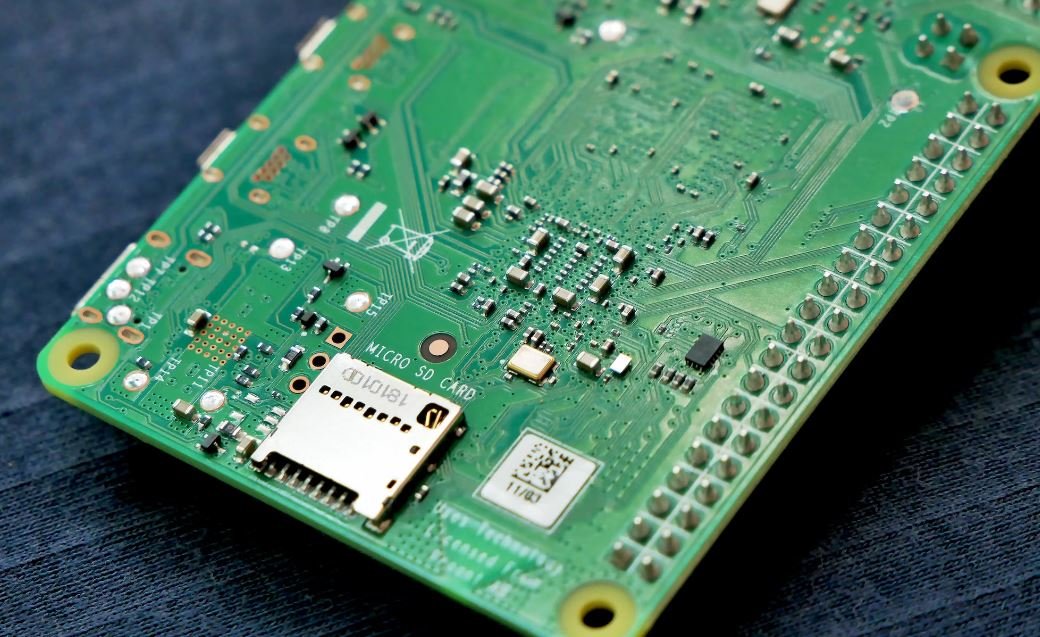
The Impact of AI Model in Training Athletes
Artificial intelligence (AI) models have revolutionized the field of sports training, offering athletes improved performance and unrivaled technical advancements. This article explores ten examples that highlight the profound impact of AI models on athletic training.
1. Performance Tracking
AI models can track an athlete’s performance metrics accurately in real-time, including distance covered, speed, heart rate, and more. This data helps coaches tailor training programs for better results.
2. Injury Prevention
Through machine learning algorithms, AI models can analyze athletes’ movement patterns, identifying potential injury risks. Coaches and trainers can adjust training regimes to minimize injuries and ensure long-term athletic success.
3. Nutritional Guidance
AI models incorporate comprehensive nutritional databases, providing personalized dietary recommendations based on an athlete’s specific goals, body composition, and training requirements.
4. Tactical Analysis
By analyzing extensive game footage, AI models reveal patterns, tendencies, and strategic insights, helping teams develop effective game plans against opponents.
5. Mental Skills Training
AI models offer mental skills training by assessing an athlete’s responses to hypothetical situations, enabling them to develop better focus, decision-making abilities, and resilience.
6. Injury Rehabilitation
AI models aid in injury rehabilitation by tracking an athlete’s progress, analyzing recovery data, and suggesting customized rehab exercises to expedite the recovery process.
7. Biomechanical Analysis
Using motion-tracking sensors, AI models provide precise biomechanical analysis, identifying areas for improvement in an athlete’s technique, posture, and movement efficiency.
8. Athlete Selection
Athlete selection is optimized through AI models that analyze various factors like physical attributes, technical skills, and mental aptitude to identify and recruit talented individuals.
9. Virtual Reality Training
AI models simulate realistic training environments using virtual reality, allowing athletes to practice in high-pressure situations and improve their performance without physical strain.
10. Predictive Analytics
AI models use historical data and predictive analytics to anticipate an athlete’s future performance, aiding in developing strategies for achieving optimal results.
As AI models continue to advance, the potential for transformative impact in athletic training grows exponentially. These ten examples illustrate the diverse applications and benefits that AI brings to the field. By harnessing the power of AI, athletes and coaches can push the boundaries of performance and unlock new levels of achievement.