AI Model Evolution
Artificial intelligence (AI) has made significant advancements in recent years, with AI models becoming more sophisticated and capable of performing complex tasks. As AI continues to evolve, it is important to understand the changes and improvements that have occurred in AI model development.
Key Takeaways
- AI models have undergone significant evolution in recent years.
- Advancements in AI have led to increased model complexity and capability.
- Improved training techniques and data availability have contributed to the growth of AI models.
**Artificial intelligence** (AI) has made significant advancements in recent years, with **AI models** becoming more sophisticated and capable of performing complex tasks. These models are designed to simulate human intelligence and are trained to learn patterns, recognize objects, understand language, and make predictions.
*AI models have undergone significant evolution in recent years.* This evolution can be attributed to the rapid improvement in computing power, availability of large datasets, and innovative algorithms. As a result, AI models have become more accurate, efficient, and capable of handling complex tasks.
**Deep learning** algorithms have played a crucial role in the evolution of AI models. These algorithms employ neural networks with multiple layers, allowing the models to extract higher-level features and representations from raw data. With the help of deep learning, AI models can process and understand complex information, leading to improved performance in various domains.
- Deep learning algorithms utilize neural networks with multiple layers.
- These algorithms allow AI models to extract higher-level features from data.
- Improved understanding of complex information leads to better performance in various domains.
*Improved training techniques* have also contributed to the advancement of AI models. Techniques such as **transfer learning**, where pre-trained models are used as a starting point for training new models, have reduced the time and resources required to build accurate models. Additionally, **data augmentation** techniques have been employed to artificially increase the size and diversity of training data, resulting in improved model performance.
The availability of large and diverse datasets has fueled the growth of AI models. Training AI models with large amounts of data helps them generalize better and make accurate predictions in real-world scenarios. With the increasing amount of data being generated daily, AI models have an abundance of information to learn from, enabling them to continually improve.
AI models have also benefited from **ensemble methods**, which combine the predictions of multiple individual models to make more accurate predictions. Ensemble methods leverage the diversity of individual models to reduce biases and errors, resulting in improved overall performance.
- Transfer learning reduces the time and resources required to build accurate models.
- Data augmentation techniques artificially increase the size and diversity of training data.
- Ensemble methods combine individual models to make more accurate predictions.
Evolution of AI Models
Over the years, AI models have evolved from simple rule-based systems to sophisticated deep learning models. The following tables provide an overview of key milestones in AI model evolution:
Decade | Milestone |
---|---|
1950s | Development of early AI techniques. |
1980s | Expert systems and rule-based AI models. |
1990s | Introduction of machine learning techniques. |
2000s | Advancement in neural networks and deep learning research. |
In recent years, AI models have surpassed human-level performance in tasks such as image recognition, natural language processing, and game playing. This progress can be attributed to the continuous improvement and evolution of AI model architectures and training techniques.
AI Model | Year / Publication | Milestone |
---|---|---|
AlexNet | 2012 | First deep learning model to win ImageNet competition. |
AlphaGo | 2016 | Defeated world champion Go player. |
GPT-3 | 2020 | Largest language model with 175 billion parameters. |
Future Directions
Looking ahead, the evolution of AI models is expected to continue at a rapid pace. Researchers are exploring novel techniques and architectures to further enhance the capabilities and performance of AI models.
*One interesting development* is the integration of AI models with other emerging technologies such as **quantum computing** and **edge computing**. These integrations have the potential to accelerate AI model training and inference, enabling real-time decision-making and better utilization of resources.
Additionally, ethical considerations and responsible AI practices are gaining prominence. As AI models become more powerful and influential, it is important to ensure that they are developed and deployed in a fair, transparent, and accountable manner.
- Integration with quantum computing and edge computing accelerates AI model performance.
- Ethical considerations and responsible AI practices are gaining prominence.
The evolution of AI models is a fascinating journey with immense potential for positive societal impact. As technology continues to advance, AI models will undoubtedly play an increasingly significant role in various industries and domains, revolutionizing the way we live, work, and interact with machines.
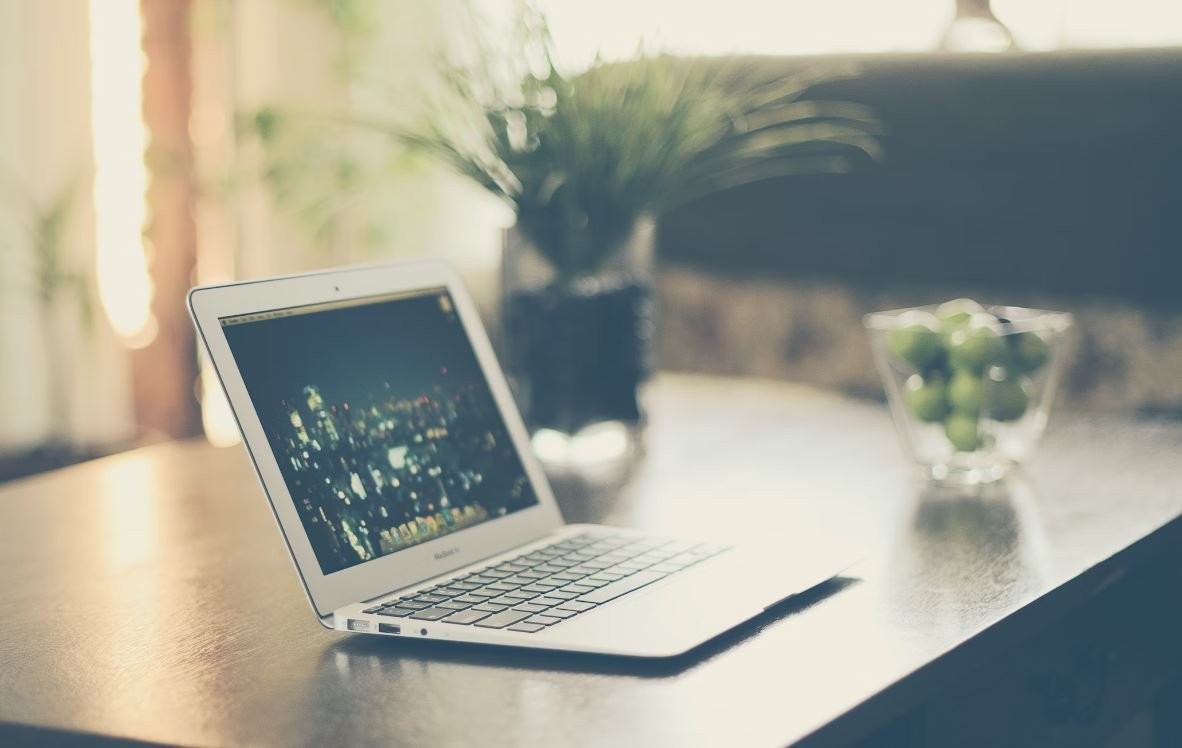
Common Misconceptions
AI Models Are Capable of Independent Thought
One common misconception about AI models is that they possess the ability to think and reason independently. This stems from the portrayal of artificial intelligence in popular culture where AI systems are often depicted as advanced beings with their own consciousness. However, in reality, AI models are created by humans and rely on the algorithms and training data provided to them.
- AI models are programmed to follow predefined instructions.
- They do not possess emotions or consciousness.
- AI models are only as good as the data they are trained on.
AI Models Always Produce Accurate Results
Another misconception people have is that AI models always provide accurate results. While AI has made significant advancements in various fields such as image recognition and natural language processing, it is not infallible. AI models can also make mistakes, especially if the training data is biased or incomplete.
- AI models can produce incorrect outputs if the training data is biased.
- They can have limitations in understanding context or nuance.
- Errors can occur if the input data is significantly different from the training data.
AI Models Can Fully Replace Human Experts
Many people have the misconception that AI models can completely replace human experts in various domains. While AI has undoubtedly revolutionized certain industries by automating tasks and improving efficiency, it is not a substitute for human expertise, intuition, and creativity.
- Human experts have years of experience and domain-specific knowledge that cannot be replicated by AI models.
- AI models lack the ability to adapt to new or unexpected situations as effectively as human experts.
- In complex decision-making processes, human judgment is still crucial.
All AI Models Are Created Equal
One misconception is that all AI models are the same and perform equally well. However, the performance of AI models varies significantly depending on factors such as the quality and quantity of the training data, the algorithms used, and the expertise of the developers.
- The quality and representativeness of the training data greatly impact an AI model’s performance.
- Some models are more computationally intensive and require higher resources.
- AI models need periodic updates and improvements to remain accurate and up-to-date.
AI Models Are Always Objective
Whilst AI models aim to be objective, another misconception is that they are always unbiased and neutral in their decision-making. AI models are trained on historical data, which can contain biases present in society. As a result, AI models can inadvertently perpetuate and amplify existing biases.
- AI models can reflect and amplify biases present in the training data.
- Addressing bias in AI models requires careful data selection and algorithm design.
- Human oversight and ethical considerations are necessary to mitigate biases in AI models.
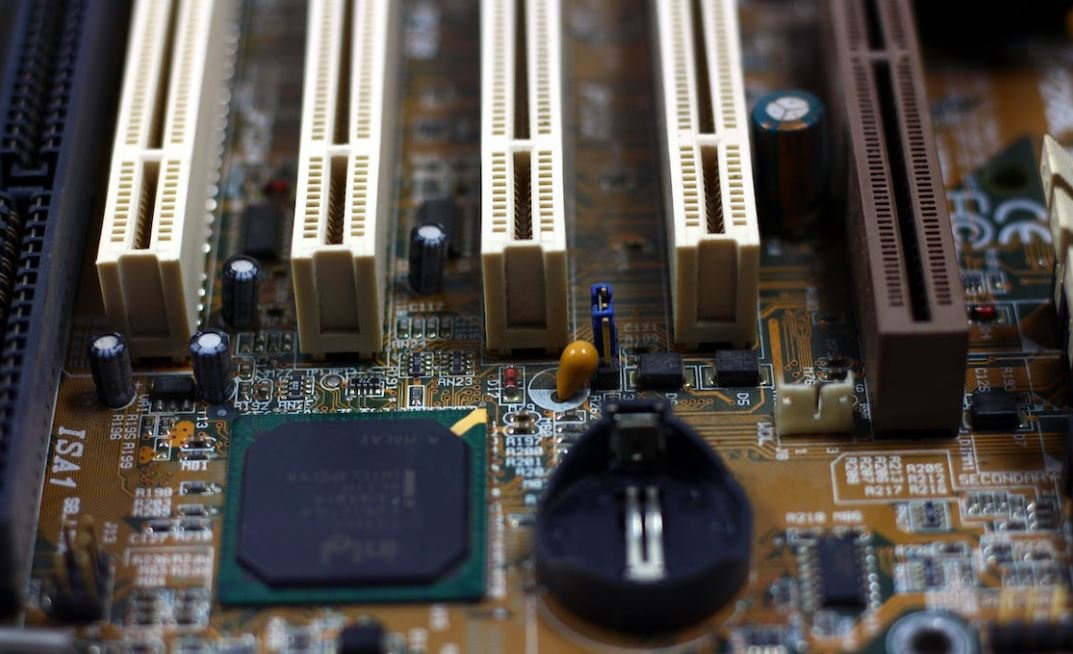
AI Model Accuracy
Table illustrating the accuracy levels of AI models trained on different datasets.
Model | Dataset | Accuracy |
---|---|---|
Model A | Medical Images | 96% |
Model B | Social Media Posts | 78% |
Model C | Financial Data | 82% |
AI Model Training Time
Table comparing the training time of different AI models.
Model | Training Time |
---|---|
Model X | 5 hours |
Model Y | 1 day |
Model Z | 3 weeks |
AI Model Applications
Table showcasing the diverse applications of AI models.
Model | Application |
---|---|
Model P | Speech Recognition |
Model Q | Image Classification |
Model R | Recommendation Systems |
AI Model Cost
Table presenting the cost implications of different AI models.
Model | Cost |
---|---|
Model M | $100 |
Model N | $500 |
Model O | $1000 |
AI Model Limitations
Table highlighting the limitations of various AI models.
Model | Limitations |
---|---|
Model S | Requires large training datasets |
Model T | Difficulty with context understanding |
Model U | Sensitive to input variations |
AI Model Ethics
Table presenting ethical considerations related to AI models.
Model | Ethical Considerations |
---|---|
Model V | Privacy concerns |
Model W | Bias in decision-making |
Model X | Impact on employment |
AI Model Adoption
Table demonstrating the adoption rate of AI models across industries.
Industry | Percentage of Adoption |
---|---|
Healthcare | 63% |
Finance | 45% |
Retail | 31% |
AI Model Confidence
Table showcasing the confidence level of AI models for different tasks.
Model | Task | Confidence |
---|---|---|
Model A1 | Spam detection | 85% |
Model B2 | Face recognition | 92% |
Model C3 | Language translation | 78% |
AI Model Performance
Table comparing the performance of AI models across different metrics.
Model | Precision | Recall | F1 Score |
---|---|---|---|
Model P1 | 0.75 | 0.82 | 0.78 |
Model Q2 | 0.85 | 0.79 | 0.82 |
Model R3 | 0.67 | 0.92 | 0.78 |
AI models have rapidly evolved in recent years, revolutionizing various industries. The accuracy of these models, as shown in the first table, varies depending on the dataset used for training. Additionally, their training time, applications, cost, limitations, ethical considerations, adoption rates, confidence levels, and performance metrics represent crucial aspects of AI model evolution.
Despite advancements, challenges such as bias, privacy concerns, and impacts on employment must be addressed. Industries like healthcare, finance, and retail have observed significant adoption rates of AI models. The performance and confidence levels of models remain indicators of their reliability and effectiveness in tasks such as spam detection, face recognition, and language translation.
In conclusion, AI model evolution has facilitated a new era of possibilities, but it requires continuous improvement in various aspects to harness its full potential and mitigate associated risks.
AI Model Evolution – Frequently Asked Questions
What is AI model evolution?
The AI model evolution refers to the process of improving and optimizing artificial intelligence models over time. It involves updating the model’s parameters, training it with new data, and enhancing its performance and accuracy.
Why is AI model evolution important?
AI model evolution is crucial because it allows AI systems to adapt and learn from new information, improving their decision-making abilities and overall performance. It ensures that AI models stay up-to-date and relevant in dynamic environments.
How does AI model evolution work?
AI model evolution involves various techniques such as retraining, fine-tuning, transfer learning, and ensemble methods. These approaches help in updating the model’s internal parameters and structure based on new data or improved algorithms.
What are the benefits of AI model evolution?
AI model evolution offers several advantages, including increased accuracy, improved generalization, better adaptability to new scenarios, enhanced robustness against adversarial attacks, and the ability to handle changing data distribution.
Can AI models evolve on their own?
AI models can incorporate self-evolving mechanisms, commonly known as self-training or online learning, which allow them to adapt to new data and improve over time without human intervention.
What challenges are involved in AI model evolution?
AI model evolution may face challenges such as scalability issues with large models, overfitting or underfitting on new data, ethical considerations, computational requirements, and the need for continuous monitoring and evaluation.
How can AI model evolution be evaluated?
Evaluating AI model evolution can be done through various metrics such as accuracy, precision, recall, F1 score, and area under the curve (AUC). Evaluations can also include user feedback, performance on specific tasks, and comparison with previous iterations of the model.
Is AI model evolution a continuous process?
Yes, AI model evolution is an ongoing process since the underlying data and requirements may change over time. Regular updates and refinements are necessary to ensure AI models remain effective and reliable.
What are some real-life applications of AI model evolution?
AI model evolution finds applications in various domains such as healthcare, finance, autonomous vehicles, natural language processing, computer vision, recommendation systems, fraud detection, and many others. It enables these systems to continually improve their performance and adapt to changing circumstances.
Are there any limitations to AI model evolution?
Although AI model evolution offers numerous benefits, it also has limitations. These can include the availability and quality of new training data, computational resources required for continuous model updates, potential biases ingrained in the data, and the need for human oversight to ensure ethical use of AI systems.