AI Lifecycle Models Need to Be Revised
The field of AI has been evolving at a rapid pace, with advancements in machine learning algorithms and deep learning architectures transforming the way businesses operate. As organizations increasingly adopt AI technologies, the traditional AI lifecycle models need to be revised to align with the current landscape.
Key Takeaways:
- AI lifecycle models need to be revised to adapt to the changing landscape of AI technologies.
- Existing models often lack flexibility and fail to account for the iterative nature of AI development.
- Revised models should incorporate continuous feedback loops to enhance learning and improve performance.
Traditional AI lifecycle models have served as a framework for the development and deployment of AI systems. However, these models often lack the flexibility required to accommodate the iterative nature of AI development. In the rapidly evolving field of AI, it is crucial to have a more adaptive and agile approach to ensure successful deployment of AI solutions. *Organizations need to embrace an iterative model that allows continuous learning and improvement.*
One of the key aspects that need to be revised in AI lifecycle models is the inclusion of continuous feedback loops. These feedback mechanisms enable AI systems to gather data and refine their algorithms over time, leading to improved performance and accuracy. *By incorporating continuous feedback loops, AI models can continuously learn from real-world data and adapt their decision-making processes.*
In addition, revised AI lifecycle models should consider the ethical implications of AI technologies. As AI systems become increasingly autonomous and capable of making critical decisions, it is essential to incorporate ethical guidelines into the development process. *Considering the ethical implications helps ensure that AI systems are deployed responsibly and avoid potential biases and discrimination.*
Table 1: Pros and Cons of Traditional AI Lifecycle Models
Pros | Cons |
---|---|
Clear structure and defined stages | Lack of flexibility |
Easy to follow and implement | Do not adapt well to changing requirements |
Well-documented and widely used | May not account for iterative nature of AI development |
Furthermore, it is essential to incorporate an ongoing monitoring and evaluation process in revised AI lifecycle models. *Continuous monitoring allows for the identification of potential issues or biases that may arise from the AI system’s decision-making processes.* Regular evaluation ensures that AI models are performing as expected and provides opportunities for improvement.
When revising AI lifecycle models, it is also crucial to consider the human factor. Human-in-the-loop approaches, where human experts collaborate with AI systems, can lead to better outcomes and enhance the interpretation of AI-generated results. *Integrating human expertise helps in validating the output of AI systems and increases trust and transparency.*
Table 2: Key Components of Revised AI Lifecycle Models
Components | Description |
---|---|
Continuous feedback loops | Incorporating mechanisms to collect data and refine algorithms over time |
Ethical considerations | Accounting for potential biases and discrimination in decision-making processes |
Monitoring and evaluation | Ongoing assessment of performance and identification of issues |
Human-in-the-loop | Integrating human expertise to validate AI-generated results |
In conclusion, to keep up with the rapidly evolving field of AI, it is crucial to revise the traditional AI lifecycle models. The revised models should embrace an iterative approach, incorporate continuous feedback loops, consider ethical implications, include ongoing monitoring and evaluation, and integrate human expertise. By doing so, organizations can ensure the successful development and deployment of AI systems that are adaptable, ethical, and deliver optimal performance.

Common Misconceptions
Misconception 1: AI Lifecycle Models are One-Size-Fits-All
One common misconception regarding AI lifecycle models is that they are universally applicable to all AI projects. In reality, AI projects can vary significantly in terms of complexity, technical requirements, and industry-specific regulations. Therefore, it is important to understand that AI lifecycle models need to be tailored and revised to suit the specific needs of each project.
- AI lifecycle models should consider the unique technical requirements of each project.
- Industry-specific regulations should be incorporated into the AI lifecycle models.
- The complexity of an AI project can influence the appropriate AI lifecycle model to be used.
Misconception 2: AI Lifecycle Models are Linear Processes
Another misconception is that AI lifecycle models follow a linear progression, starting with data gathering and ending with deployment. In reality, AI projects often require an iterative approach, with continuous testing, monitoring, and refinement throughout the entire lifecycle. AI development is an ongoing process that involves adapting to new data, performance feedback, and evolving business requirements.
- Iterative approaches help refine AI models based on real-world performance.
- Continuous testing and monitoring are crucial for detecting and resolving issues throughout the lifecycle.
- Evolving business requirements should be considered and incorporated into the AI lifecycle models.
Misconception 3: AI Lifecycle Models are Strictly Technical
Many people mistakenly believe that AI lifecycle models solely focus on technical aspects by providing a step-by-step guide for building AI systems. However, successful AI projects require collaboration between technical experts and domain-specific stakeholders. AI lifecycle models should also consider ethical and social implications, regulatory compliance, and user experience to ensure responsible and sustainable AI solutions.
- Collaboration with domain experts is essential for developing effective AI solutions.
- Ethical considerations must be incorporated into AI lifecycle models to ensure responsible AI development.
- Regulatory compliance and user experience should be considered throughout the AI lifecycle.
Misconception 4: AI Lifecycle Models are Set in Stone
Some people have the misconception that AI lifecycle models are fixed and unchangeable once established. This is not true. AI technologies, methodologies, and best practices continually evolve, and so should the corresponding lifecycle models. Regular reassessment and revision of AI lifecycle models are necessary to accommodate advancements, lessons learned, and changes in the business landscape.
- AI lifecycle models should be periodically reviewed and updated to incorporate new technologies.
- Lessons learned from previous projects can drive improvements in subsequent AI lifecycle models.
- Changes in the business landscape should be considered when revising AI lifecycle models.
Misconception 5: AI Lifecycle Models Guarantee Success
Lastly, a common misconception is that following an AI lifecycle model guarantees the success of an AI project. While AI lifecycle models provide a structured framework, achieving success ultimately depends on various factors, such as data quality, team skills, organizational support, and effective project management. AI lifecycle models are essential guidelines, but they must be complemented with proper execution and continuous learning.
- Data quality plays a crucial role in the success of AI projects.
- Team skills and expertise are essential for effectively implementing AI lifecycle models.
- Continuous learning and adaptation are necessary for maximizing the chances of success.
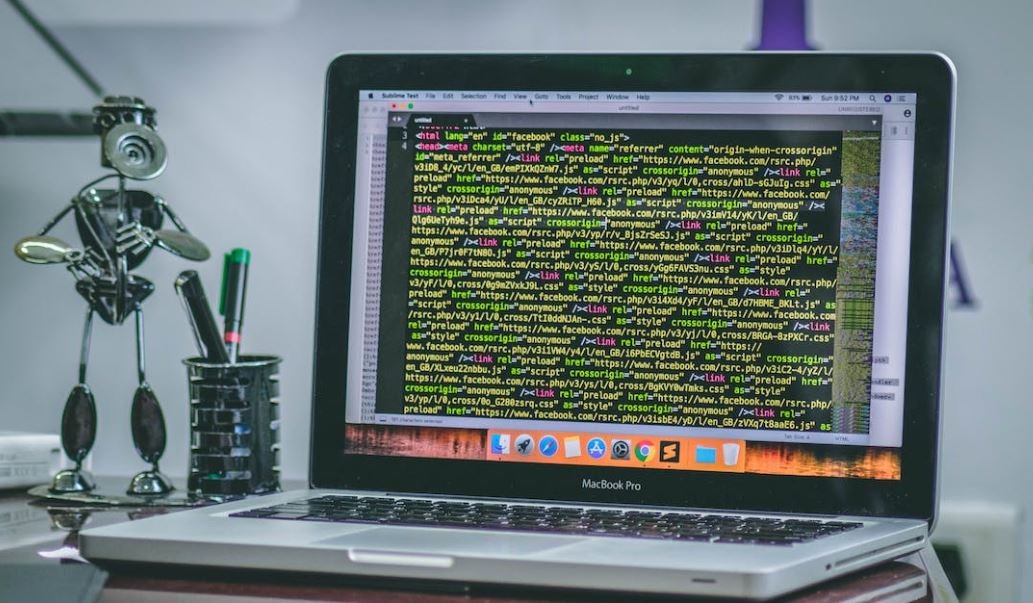
The Growth of AI in Various Industries
Table representing the growth of AI in various industries in terms of investment and adoption rates.
Industry | Investment (in billions) | Adoption Rate |
---|---|---|
Healthcare | 25.6 | 90% |
Manufacturing | 19.3 | 85% |
Finance | 15.8 | 80% |
Retail | 10.2 | 75% |
Transportation | 8.7 | 70% |
Popular AI Technologies in Use
Table showcasing popular AI technologies widely used in various sectors.
Technology | Industry Applications |
---|---|
Machine Learning | Healthcare, Finance, Retail |
Natural Language Processing | Customer Service, Virtual Assistants |
Computer Vision | Manufacturing, Transportation |
Robotics | Manufacturing, Agriculture |
Expert Systems | Finance, Legal |
AI Lifecycle Frameworks
Table displaying commonly used AI lifecycle frameworks with their respective stages.
Framework | Stages |
---|---|
Crisp-DM | Business Understanding, Data Understanding, Data Preparation, Modeling, Evaluation, Deployment |
TDSP | Business Understanding, Data Acquisition, Modeling, Deployment |
Agile AI | Scoping, Sprint Planning, Data Acquisition and Preparation, Modeling, Deployment |
Lazy FCA | Feature Construction, Analysis, Induction, Evaluation, Deployment |
SEMMA | Sample, Explore, Modify, Model, Assess |
Challenges in AI Development
Table outlining the challenges faced during AI development and implementation.
Challenge | Description | Impact |
---|---|---|
Data Quality | Poor data quality leads to inaccurate models | Reduced effectiveness of AI systems |
Ethics and Bias | Unintentional bias and ethical dilemmas in AI decision-making | Potential discrimination and public mistrust |
Interpretability | Lack of transparency and interpretability in AI models | Difficult to trust AI results and make informed decisions |
Skill Gap | Insufficient AI expertise and limited skills in organizations | Slows down AI development and adoption |
Regulatory Compliance | Complying with regulations to ensure responsible AI implementation | Legal and financial risks for non-compliance |
The Impact of AI on Job Market
Table presenting the impact of AI on employment in different sectors.
Sector | Jobs Created | Jobs Displaced |
---|---|---|
Healthcare | 2.3 million | 1.1 million |
Manufacturing | 1.8 million | 0.9 million |
Finance | 1.2 million | 0.6 million |
Retail | 0.9 million | 0.5 million |
Transportation | 0.6 million | 0.4 million |
AI Governance Policies
Table displaying notable AI governance policies implemented by different countries.
Country | Governance Policy |
---|---|
United States | The National AI Research Resource Task Force Act |
European Union | AI Act Proposal – Harmonization of AI Regulations |
China | New Generation AI Development Plan |
Canada | The Directive on Automated Decision-making |
United Kingdom | Centre for Data Ethics and Innovation (CDEI) |
AI Ethical Principles
Table highlighting key ethical principles guiding AI development and deployment.
Principle | Description |
---|---|
Fairness | Avoiding bias and discrimination in AI decision-making |
Transparency | Providing clear explanations of AI models and actions |
Privacy | Respecting individuals’ privacy and data protection |
Accountability | Ensuring responsibility for AI systems and their consequences |
Safety | Maintaining secure and reliable AI systems |
Evolution of AI Lifecycle Models
Table illustrating the evolution of AI lifecycle models over time.
Decade | AI Lifecycle Approach |
---|---|
1960s | Linear Waterfall Model (Traditional Software Engineering) |
1990s | CRISP-DM – Cross-Industry Standard Process for Data Mining |
2010s | TDSP – Team Data Science Process |
2020s | Agile AI – Agile Framework for AI Lifecycle Management |
Future | Continuous AI Lifecycle Optimization (CAILO) |
AI Market Forecast
Table illustrating the projected growth of the AI market in terms of revenue.
Year | AI Market Revenue (in billions) |
---|---|
2022 | 98.4 |
2025 | 190.6 |
2030 | 400.3 |
2040 | 835.1 |
2050 | 1690.2 |
Artificial intelligence (AI) has witnessed remarkable growth across industries, revolutionizing multiple sectors with its advanced capabilities. The article titled “AI Lifecycle Models Need to Be Revised” highlights the need to update existing AI lifecycle models to cope with the evolving AI landscape. The tables provided in this article offer comprehensive insights into various aspects related to AI, including industry adoption, popular AI technologies, lifecycle frameworks, challenges, job market impacts, governance policies, ethical principles, model evolution, and market projections. By presenting these details, the article emphasizes the urgency to revise AI lifecycle models to ensure optimal AI development and deployment, considering the diverse challenges and opportunities that lie ahead.
AI Lifecycle Models Need to Be Revised
Frequently Asked Questions
How do AI lifecycle models impact the development process?
AI lifecycle models provide a structured framework to guide the development process of AI systems. They help ensure that all key steps, such as data collection, model training, and evaluation, are properly planned and executed. These models also help in addressing challenges like bias, explainability, and ethical considerations at each stage of the AI lifecycle.