Introduction:
Artificial Intelligence (AI) deployment models play a crucial role in implementing AI solutions and leveraging its capabilities effectively. Organizations must choose the right deployment model that aligns with their goals, resources, and operational requirements. In this article, we examine different AI deployment models and discuss their benefits and considerations.
Key Takeaways:
– AI deployment models are essential for the successful implementation of AI solutions.
– Organizations must choose a deployment model that aligns with their goals and operational requirements.
– The choice of AI deployment model impacts factors such as cost, scalability, and data privacy.
Understanding AI Deployment Models:
AI deployment models determine how an AI solution is implemented and operated within an organization. These models range from traditional on-premises deployments to cloud-based and hybrid solutions. Organizations must consider their specific needs and constraints when selecting a deployment model.
**The choice of an AI deployment model affects factors such as cost, scalability, and data privacy**. It is essential to evaluate the benefits and considerations of each model before making a decision.
On-Premises AI Deployment:
On-premises AI deployment involves hosting AI solutions within an organization’s own infrastructure. This model offers full control, data privacy, and high performance but requires significant upfront investment in infrastructure and maintenance.
*Organizations with strict data security requirements may prefer on-premises deployment to maintain complete control over their data*.
Cloud-Based AI Deployment:
Cloud-based AI deployment relies on cloud infrastructure provided by third-party vendors. This model offers scalability, easy access, and cost-effectiveness as organizations pay for the resources they use. Cloud providers handle infrastructure maintenance, allowing businesses to focus on leveraging AI capabilities.
*Cloud-based deployment eliminates the need for significant upfront investment and provides flexibility to scale resources as needed*.
Hybrid AI Deployment:
Hybrid AI deployment combines on-premises and cloud-based solutions. This model allows organizations to leverage the benefits of both approaches. They can store sensitive data on-premises, while utilizing the scalability and cost advantages of the cloud for computation and processing.
*Hybrid deployment offers the flexibility to manage data securely while utilizing cloud resources when required*.
Comparing AI Deployment Models:
Deployment Model | Benefits | Considerations |
---|---|---|
On-Premises | High control and data privacy | High upfront costs and maintenance |
Cloud-Based | Scalability and cost-effectiveness | Data security concerns |
Hybrid | Data privacy and flexible resource allocation | Potential complexity in integration |
Considerations for AI Deployment:
When choosing an AI deployment model, several factors need to be considered:
1. **Scalability**: Organizations with fluctuating computational demands may benefit from cloud-based or hybrid solutions that allow them to scale resources as needed.
2. **Data Privacy and Security**: Industries that handle sensitive data, such as healthcare or finance, may lean towards on-premises or hybrid deployment models to maintain control and compliance.
3. **Cost**: Cloud-based solutions offer cost advantages as organizations only pay for the resources they use. However, long-term costs should be evaluated, considering factors such as data transfer and storage fees.
4. **Infrastructure Requirements**: On-premises deployment requires organizations to invest in and maintain their infrastructure, including hardware, software, and IT resources.
5. **Integration and Capabilities**: Organizations must assess the compatibility of their existing systems with different deployment models and consider how easily they can integrate AI capabilities into their workflows.
Table 2: Key Considerations for AI Deployment
1. Scalability
2. Data privacy and security
3. Cost
4. Infrastructure requirements
5. Integration and capabilities
Choosing the Right AI Deployment Model:
Choosing the most suitable AI deployment model depends on the unique requirements and constraints of each organization. Careful consideration of factors such as data privacy, scalability, cost, and integration capabilities is necessary to make an informed decision.
By evaluating the benefits, limitations, and considerations of each deployment model, businesses can select the AI deployment model that best aligns with their goals and resources.
In conclusion:
Deploying AI effectively requires organizations to carefully consider different deployment models and choose the one that suits their specific needs. Whether through on-premises, cloud-based, or hybrid deployment, organizations must evaluate factors such as cost, scalability, data privacy, and integration capabilities to ensure the successful implementation of AI solutions.
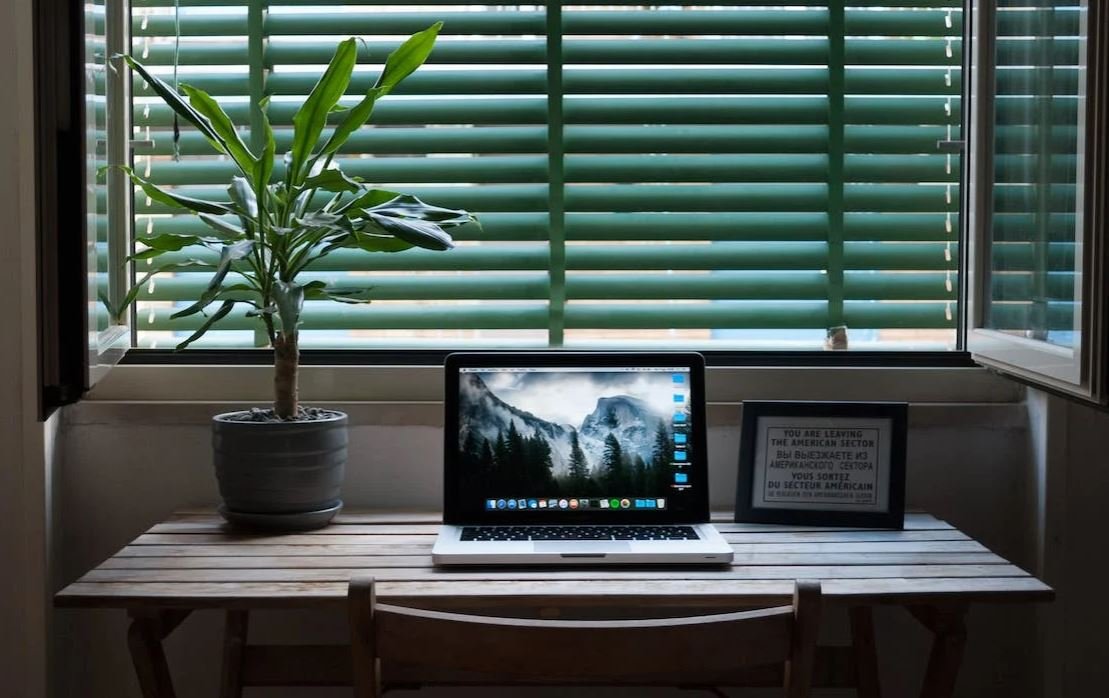
Common Misconceptions
AI Deployment Models
There are several common misconceptions that people have around AI deployment models. These misconceptions can lead to misunderstandings and misinformed decisions. It’s important to address these misconceptions to ensure a clear understanding of how AI deployment models work.
- AI deployment models are only beneficial for large corporations.
- All AI deployment models are the same.
- AI deployment models will replace human workers entirely.
Contrary to popular belief, AI deployment models are not only beneficial for large corporations. Many small and medium-sized businesses can also leverage AI deployment models to improve their operations and gain a competitive advantage. AI can automate repetitive tasks, increase productivity, and provide valuable insights for businesses of all sizes.
- AI deployment models require significant investment and expertise.
- AI deployment models always result in job losses.
- AI deployment models are a one-time implementation.
Another misconception is that all AI deployment models are the same. In reality, there are different types of AI deployment models, such as cloud-based AI, on-premises AI, and hybrid AI. Each model has its own advantages and considerations, and organizations need to choose the model that best aligns with their specific needs and requirements.
- AI deployment models can be costly and time-consuming to implement.
- AI deployment models are only applicable in certain industries.
- AI deployment models are fully autonomous and do not require human intervention.
It is also a misconception that AI deployment models always result in job losses. While AI can automate certain tasks, it also creates new opportunities and roles. AI deployment models can enhance human workers’ capabilities, allowing them to focus on more strategic and creative tasks. Collaboration between AI and humans can lead to greater productivity and innovation in various industries.
- AI deployment models are only beneficial for customer-facing applications.
- AI deployment models are prone to errors and biases.
- AI deployment models are a fad that will soon fade away.
Lastly, some people believe that AI deployment models are a one-time implementation. In reality, AI deployment models require continuous monitoring, training, and improvement to ensure optimal performance. It is an ongoing process that requires investment in resources and expertise. AI deployment models need to adapt and evolve along with the changing needs and advancements in technology.
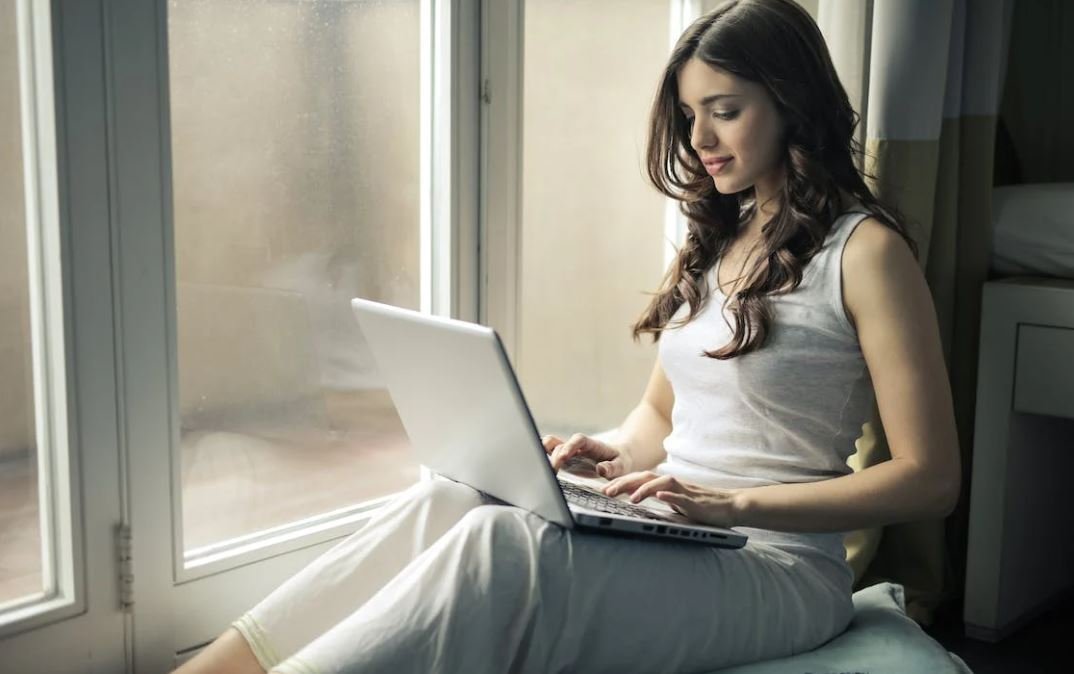
AI Deployment Models
This article discusses various AI deployment models that are commonly used in businesses. AI deployment models determine how artificial intelligence is implemented and utilized within an organization. Different models offer unique advantages and considerations. The following tables provide valuable insights into these deployment models, showcasing their characteristics, benefits, and use cases.
Deployment Model 1: On-Premises
The on-premises AI deployment model refers to hosting AI resources and infrastructure within the organization’s own physical premises. This model allows for direct control, customization, and seamless integration with existing systems.
Characteristics | Benefits | Use Cases |
---|---|---|
Locally hosted AI resources | Full control and data privacy | Highly sensitive industries |
Customizable infrastructure | Reduced network latency | Mission-critical applications |
Deployment Model 2: Cloud-based
The cloud-based AI deployment model involves utilizing cloud computing resources for hosting and managing AI applications. This model offers scalability, ease of access, and cost-efficiency by leveraging cloud service providers’ infrastructure.
Characteristics | Benefits | Use Cases |
---|---|---|
Hosted on cloud servers | Scalability and flexibility | Startups and small businesses |
Accessible from anywhere | Reduced infrastructure costs | Data-intensive applications |
Deployment Model 3: Hybrid
The hybrid AI deployment model combines both on-premises and cloud-based approaches to leverage the benefits of both worlds. This model allows organizations to maintain certain resources internally while utilizing cloud services for others.
Characteristics | Benefits | Use Cases |
---|---|---|
Combination of local and cloud resources | Flexibility and control | Enterprise-level applications |
Optimal resource allocation | Cost-efficient scalability | Data privacy regulations |
Deployment Model 4: Edge Computing
The edge computing AI deployment model involves processing data and running AI algorithms directly on edge devices, such as IoT devices or smartphones. This brings AI capabilities closer to the data source and enables real-time decision-making.
Characteristics | Benefits | Use Cases |
---|---|---|
The AI processing at the edge | Reduced latency and bandwidth usage | Smart cities and IoT applications |
Real-time decision-making | Data privacy and security | Remote or low-connectivity environments |
Deployment Model 5: Private Cloud
The private cloud AI deployment model involves dedicated infrastructure that is solely used by a single organization. It offers enhanced security, control, and flexibility compared to public cloud solutions.
Characteristics | Benefits | Use Cases |
---|---|---|
Dedicated cloud infrastructure | Higher data security and privacy | Financial institutions |
Customized resource allocation | Regulatory compliance | Government departments |
Deployment Model 6: Public Cloud
The public cloud AI deployment model utilizes shared computing resources managed by cloud service providers. It offers cost-efficiency, scalability, and access to a wide range of AI-related services.
Characteristics | Benefits | Use Cases |
---|---|---|
Shared cloud infrastructure | Scalability and cost-effectiveness | Research institutions |
Easy access to AI services | Rapid prototyping and experimentation | E-commerce platforms |
Deployment Model 7: Community Cloud
The community cloud AI deployment model involves multiple organizations pooling their resources to create a shared cloud infrastructure. It offers cost-sharing benefits and enables collaboration within specific communities.
Characteristics | Benefits | Use Cases |
---|---|---|
Shared among specific organizations | Cost-sharing and resource optimization | Healthcare networks |
Collaborative research and development | Industry-specific requirements | Academic institutions |
Deployment Model 8: Virtual Private Cloud (VPC)
The virtual private cloud AI deployment model involves creating a logically isolated section within a public cloud provider’s infrastructure. It offers enhanced control, security, and network isolation.
Characteristics | Benefits | Use Cases |
---|---|---|
Secure and isolated cloud environment | Control over data and network traffic | Large enterprises |
Hybrid cloud connectivity | Compliance with industry regulations | Financial services |
Deployment Model 9: Multi-Cloud
The multi-cloud AI deployment model involves utilizing multiple cloud service providers simultaneously. This allows organizations to leverage specific capabilities and avoid vendor lock-in.
Characteristics | Benefits | Use Cases |
---|---|---|
Utilizes multiple cloud providers | Best-of-breed selection and flexibility | Global enterprises |
Avoids vendor lock-in | Redundancy and disaster recovery | Highly regulated industries |
Deployment Model 10: Serverless Computing
The serverless computing AI deployment model allows developers to build and run applications without worrying about the underlying server infrastructure. It provides scalability and cost-efficiency by charging only for actual usage.
Characteristics | Benefits | Use Cases |
---|---|---|
Automated scaling and resource management | Cost optimization based on usage | Web and mobile applications |
Faster time to market | Event-driven AI applications | Startups and innovation projects |
In conclusion, when implementing artificial intelligence, businesses have the option to choose from various deployment models, each with its own advantages and considerations. The selection of an AI deployment model depends on factors such as data privacy, scalability requirements, control, and cost-efficiency. By understanding the characteristics, benefits, and use cases of different deployment models, organizations can make informed decisions to harness the power of AI in alignment with their unique needs and goals.
Frequently Asked Questions
AI Deployment Models
What are the different AI deployment models?
The different AI deployment models include on-premises deployment, cloud-based deployment, and hybrid deployment. On-premises deployment involves installing and running the AI system locally on dedicated hardware. Cloud-based deployment refers to hosting the AI system on remote servers and accessing it over the internet. Hybrid deployment combines both on-premises and cloud-based elements, allowing for flexible and scalable AI solutions.
What are the advantages of on-premises AI deployment?
On-premises AI deployment offers increased data privacy and security since the data remains within the organization’s infrastructure. It provides better latency and faster response times as the AI system is located close to the data source. Additionally, it allows for greater customization and control over the AI infrastructure.
What are the advantages of cloud-based AI deployment?
Cloud-based AI deployment offers easy scalability, allowing organizations to quickly increase or decrease their AI resources based on demand. It eliminates the need for upfront hardware investments and enables access to advanced machine learning tools and services provided by the cloud provider. Additionally, it supports collaboration and remote access.
What is hybrid AI deployment and when is it beneficial?
Hybrid AI deployment combines aspects of on-premises and cloud-based deployment. It is beneficial when organizations have sensitive data that needs to be kept on-premises for compliance reasons but also need the scalability and flexibility of the cloud. Hybrid deployment allows for workload optimization, where certain tasks can be performed locally while others can leverage cloud resources.
What factors should be considered when choosing an AI deployment model?
When choosing an AI deployment model, several factors should be considered. These include data privacy and security requirements, scalability needs, budget constraints, available infrastructure, latency requirements, and compliance regulations. Evaluating these factors will help determine the most suitable deployment model for the organization.
Can AI deployment models be switched after implementation?
Switching AI deployment models after implementation can be complex and challenging. It may involve data migration, retraining of models, and potential downtime. However, with proper planning and consideration of the migration process, it is possible to switch between different deployment models if necessary.
What are the limitations of on-premises AI deployment?
On-premises AI deployment requires significant upfront investments in hardware, infrastructure, and maintenance. It may lack the scalability of cloud-based solutions, making it more challenging to handle sudden spikes in demand. Additionally, it may require technical expertise and dedicated personnel to manage and operate the AI system.
What are the limitations of cloud-based AI deployment?
Cloud-based AI deployment relies on reliable internet connectivity, making it vulnerable to network outages or latency issues. Organizations may face security and compliance concerns if sensitive data needs to be stored in the cloud. Additionally, reliance on external cloud providers means limited control and potential reliance on their service availability.
How can AI deployment models be optimized for cost and performance?
AI deployment models can be optimized for cost and performance through various strategies. This includes right-sizing AI resources, leveraging auto-scaling capabilities, optimizing data storage and processing, adopting containerization or serverless architectures, and continuously monitoring and tuning the AI system. Choosing the appropriate deployment model based on workload characteristics can also contribute to cost and performance optimization.
What are some popular AI deployment platforms?
Some popular AI deployment platforms include Google Cloud AI Platform, Microsoft Azure Machine Learning, Amazon SageMaker, IBM Watson, and Nvidia Triton Inference Server. These platforms offer a range of tools, services, and infrastructure to facilitate the deployment and management of AI models at scale.