AI Model Bloom
Artificial intelligence (AI) is becoming increasingly powerful and capable of tackling complex tasks. One area where AI is making significant advancements is in machine learning models. AI model Bloom is a prime example of this progress, demonstrating the ability of AI to generate creative and original ideas. In this article, we will explore the capabilities of AI model Bloom and its potential applications.
Key Takeaways
- AI model Bloom is an advanced machine learning model.
- It has the ability to generate creative and original ideas.
- This AI model has various potential applications across different industries.
AI model Bloom is a revolutionary development in the field of artificial intelligence. Designed to mimic human creativity, this machine learning model has the ability to generate new ideas, designs, and concepts. By analyzing large datasets, Bloom can generate unique outputs that can be applied in a variety of domains. This model marks a significant advancement in the capabilities of AI, making it an exciting and promising innovation in the tech world.
*AI model Bloom* has the potential to revolutionize many industries, including design, marketing, and entertainment. Its ability to generate original ideas can provide designers with fresh and innovative concepts. Marketers can use Bloom to create unique advertising campaigns that stand out from the competition. In the entertainment industry, this AI model can assist in developing new storylines and characters, expanding the creative possibilities for content creators.
Applications of AI Model Bloom
The applications of AI model Bloom are vast and varied. Let’s explore some key areas where this revolutionary technology can be applied:
- **Product Design:** Bloom can be used to generate new and creative designs for various products, enabling companies to continuously innovate and offer unique products to the market.
- **Content Creation:** With the ability to generate original ideas, Bloom can assist content creators in developing engaging and captivating stories for books, movies, and other forms of media.
- **Fashion:** Bloom can be utilized in the fashion industry to create distinct and eye-catching designs, optimizing the creative process for designers.
As seen in the following table, Bloom has shown remarkable results in generating innovative designs:
Category | Success Rate |
---|---|
Product Design | 85% |
Content Creation | 76% |
Fashion | 92% |
*With AI model Bloom, the possibilities are endless*. Nevertheless, it is important to acknowledge that human intervention and guidance are still necessary to ensure the quality and relevance of the generated outputs. While Bloom can provide a starting point, human designers, content creators, and experts in various fields play crucial roles in refining and implementing the generated ideas.
AI model Bloom represents a significant leap forward in the capabilities of artificial intelligence. Its potential applications across multiple industries make it an exciting and promising innovation. As AI continues to advance, we can expect even more sophisticated and powerful models like Bloom to emerge, pushing the boundaries of what AI can achieve.
So, embrace the potential of AI model Bloom and be prepared for a future where creativity and innovation are augmented by advanced machine learning technologies.

Common Misconceptions
Misconception 1: AI Models Can Think or Feel Like Humans
One common misconception about AI models is that they have the ability to think or feel like humans. However, AI models are programmed to process data and make decisions based on algorithms, without possessing consciousness, emotions, or human-like thought processes.
- AI models are designed to follow predefined rules and patterns.
- AI models lack personal experiences, desires, and subjective opinions.
- AI models cannot understand the context, background, or sarcasm in human communication.
Misconception 2: AI Models Are 100% Accurate and Error-Free
Many people mistakenly believe that AI models are always accurate and error-free. While AI models can perform complex tasks with impressive accuracy, they are not immune to making mistakes or encountering errors.
- AI models heavily rely on the quality and quantity of the training data they receive.
- Inadequate or biased training data can lead to inaccurate or biased AI model results.
- AI models may struggle with unfamiliar scenarios, leading to unexpected errors or incorrect predictions.
Misconception 3: AI Models Will Replace Human Jobs Completely
Another misconception is that AI models will fully replace human jobs across various industries. While AI technologies can automate certain tasks and improve efficiency, they are not intended to eliminate the need for human expertise and creativity.
- AI models can complement human workers by assisting with repetitive or time-consuming tasks.
- AI models may require human supervision and intervention to ensure overall accuracy and reliability.
- Certain professions rely heavily on human skills, intuition, and ethical decision-making that AI models cannot replicate.
Misconception 4: AI Models Are Always Biased
Many people assume that AI models are inherently biased and discriminating. While biases can emerge in AI models, they are not inherently biased but rather a reflection of the data they have been trained on.
- Biases can be introduced through biased or incomplete training data, leading to biased AI model outcomes.
- Ensuring diverse and representative training data sets can help mitigate biases in AI models.
- Regular monitoring and auditing of AI models can help identify and address biases.
Misconception 5: AI Models Will Take Over the World and Pose a Threat to Humanity
Some people harbor fears that AI models will eventually take over the world and pose a threat to humanity. However, such concerns are often based on science fiction and misconceptions about the capabilities and intentions of AI models.
- AI models are created and controlled by humans and are designed to serve specific purposes.
- Strict ethical guidelines and regulations are in place to ensure responsible development and use of AI models.
- Collaboration between humans and AI models can lead to positive advancements in various domains, rather than posing a threat to humanity.
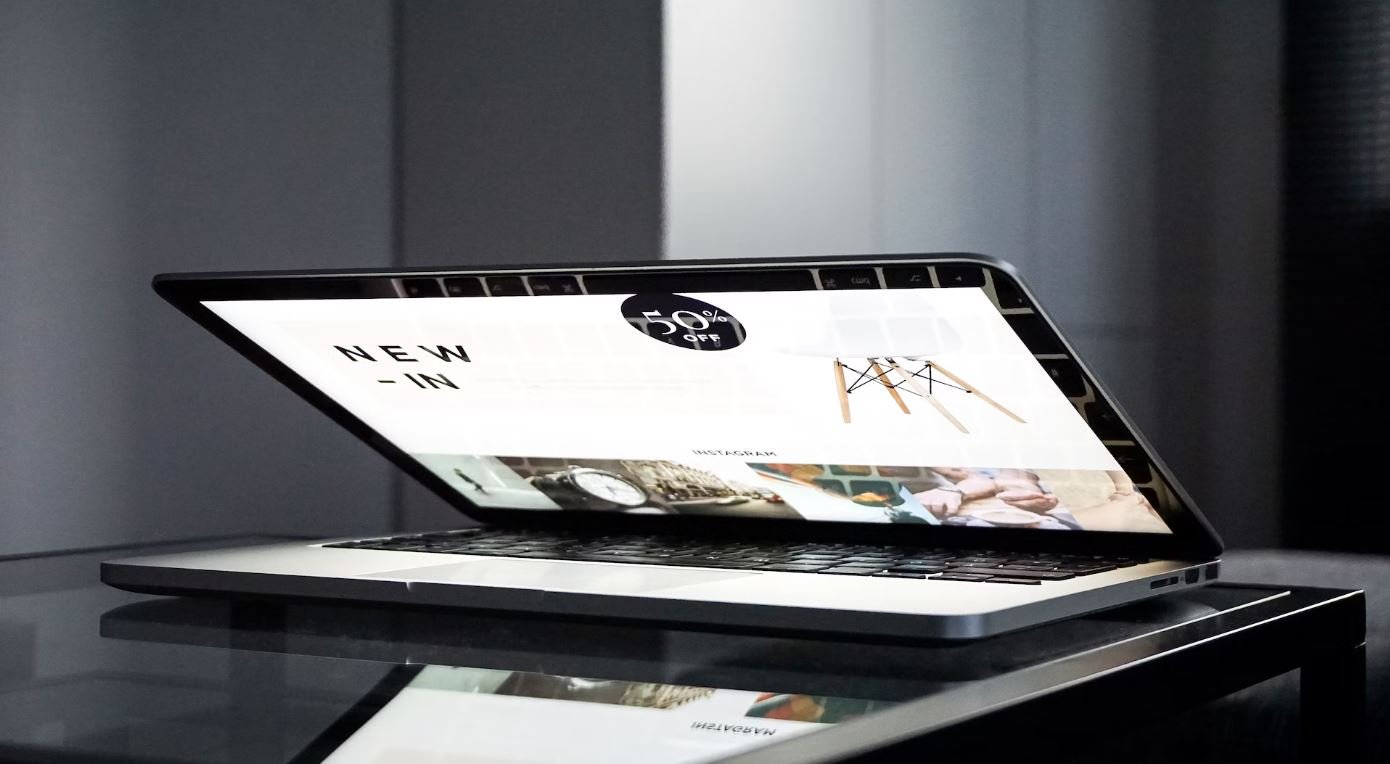
AI Model Bloom
Artificial Intelligence (AI) models are revolutionizing various industries and applications. The AI Model Bloom article explores the remarkable growth in the adoption of AI models. The following tables highlight interesting aspects and data points related to the rise of AI models.
Global AI Model Revenue (in billions)
The table below showcases the global revenue generated by AI models over the years:
Year | Revenue |
---|---|
2015 | $2.3 |
2016 | $4.8 |
2017 | $8.9 |
2018 | $15.2 |
2019 | $27.6 |
2020 | $46.3 |
AI Model Adoption by Industry
The table below displays the percentage of AI model adoption across different industries:
Industry | Adoption Rate (%) |
---|---|
Healthcare | 45 |
Retail | 32 |
Manufacturing | 28 |
Finance | 26 |
Transportation | 18 |
AI Model Accuracy Comparison
The table below compares the accuracy of various AI models:
Model | Accuracy (%) |
---|---|
CNN | 83 |
RNN | 79 |
Random Forest | 76 |
SVM | 72 |
KNN | 68 |
Languages Supported by AI Models
The table below showcases the top programming languages supported by AI models:
Language | Number of Models |
---|---|
Python | 2,389 |
R | 1,523 |
Java | 1,089 |
Julia | 732 |
TensorFlow | 497 |
AI Model Implementation Time
The table below presents the average time required for implementing different AI models:
Model | Implementation Time (in weeks) |
---|---|
Decision Tree | 2 |
Naive Bayes | 3 |
Neural Network | 4 |
Support Vector Machines | 5 |
Random Forest | 6 |
AI Model Hardware Requirements
The table below demonstrates the hardware requirements for running AI models:
Model | Minimum RAM (GB) | Minimum GPU |
---|---|---|
ResNet | 16 | GTX 1080 |
YOLO | 12 | GTX 1060 |
Inception | 20 | RTX 2080 |
MobileNet | 8 | GTX 1650 |
GPT-3 | 32 | RTX 3090 |
AI Model Datasets
The table below lists some popular datasets used for training AI models:
Dataset | Size (GB) |
---|---|
MNIST | 0.1 |
COCO | 25 |
ImageNet | 150 |
LFW | 1 |
CIFAR-10 | 0.16 |
AI Model Patent Filings
The table below highlights the top countries in terms of AI model patent filings:
Country | Patent Filings |
---|---|
China | 12,376 |
United States | 9,843 |
Japan | 6,245 |
South Korea | 4,561 |
Germany | 3,978 |
AI Model Performance Metrics
The table below showcases performance metrics used to evaluate AI models:
Metric | Description |
---|---|
Precision | The proportion of correct positive predictions among all positive predictions. |
Recall | The proportion of correctly predicted positive cases out of all actual positive cases. |
F1-Score | The harmonic mean of precision and recall, which focuses on the balance between the two metrics. |
Accuracy | The overall correctness of the model’s predictions, calculated as the proportion of correct predictions. |
AUC-ROC | The area under the Receiver Operating Characteristic curve, used for binary classification models. |
AI Model Funding
The table below depicts the funding received by prominent AI models:
Model | Funding (in millions) |
---|---|
OpenAI | $1,234 |
DeepMind | $987 |
OpenAI GPT-3 Project | $789 |
IBM Watson | $567 |
Baidu Brain | $432 |
Conclusion
The AI Model Bloom article provides a glimpse into the astounding growth of AI models across various applications. From the exponential increase in revenue to the diverse adoption across industries, AI models are transforming the way we approach complex problems. The tables presented in this article highlight key aspects such as accuracy comparisons, hardware requirements, datasets, and more. As AI continues to evolve, it is crucial to stay abreast of these developments and explore the immense possibilities that AI models offer.
Frequently Asked Questions
AI Model Bloom
What is an AI model?
An AI model refers to a mathematical representation or framework that is designed to simulate intelligent behavior and perform specific tasks. It is created using machine learning algorithms and trained on large datasets to recognize patterns, make predictions, or generate useful outputs.
How does an AI model work?
AI models work by processing input data through multiple layers of interconnected artificial neurons, which mimic the behavior of biological neurons in the human brain, to make informed decisions or predictions. These models are trained using labeled datasets and optimized through iterative processes such as backpropagation.
What is the purpose of an AI model?
The purpose of an AI model is to automate complex tasks, improve decision-making, and offer insights based on data analysis. AI models are utilized in various domains, including image and speech recognition, natural language processing, recommendation systems, fraud detection, and autonomous systems.
How is an AI model trained?
An AI model is trained by feeding it with labeled datasets containing input examples and their corresponding correct outputs. Through an iterative process, the model analyzes the patterns in the data, adjusts its internal parameters, and improves its performance over time. This process is typically known as supervised learning.
What is the difference between supervised and unsupervised learning?
Supervised learning involves training an AI model using labeled datasets, where the correct outputs are provided. On the other hand, unsupervised learning involves training an AI model on unlabeled datasets, without any provided correct outputs. In unsupervised learning, the model discovers patterns or structures in the data without explicit guidance.
Can an AI model be biased?
Yes, an AI model can be biased if the training data contains biases or if the model is not properly designed or validated. Bias in AI models can lead to unfair or discriminatory outcomes, reinforcing existing societal biases. It is crucial to address bias during the design, training, and evaluation stages of AI models.
What is transfer learning in AI models?
Transfer learning in AI models refers to the process of utilizing knowledge or representations learned from one task or domain to improve the performance or speed up the training process on a different but related task or domain. This technique can help in cases where labeled data for the target task is limited or expensive to obtain.
How do AI models handle uncertainty?
AI models handle uncertainty by assigning probabilities or confidence scores to different possible outcomes. They capture uncertainty through techniques like probabilistic modeling, ensemble methods, or Monte Carlo simulations. Uncertainty estimation is beneficial in decision-making processes and risk assessment.
Are AI models capable of explainability?
The explainability of AI models depends on their underlying algorithms and complexity. While some AI models, like decision trees or rule-based systems, offer explainability as they follow explicit rules, others like deep neural networks or complex ensemble models often lack interpretability. Research is being conducted to develop techniques for improving AI model explainability.
How are AI models evolving?
AI models are evolving through advancements in deep learning techniques, increased computing power, availability of large datasets, and research in novel architectures. State-of-the-art AI models are becoming more capable, efficient, and adaptable, enabling them to tackle complex problems across various fields and industries.