AI Training Energy Consumption
Artificial Intelligence (AI) training is a computational process that requires substantial amounts of energy. The demand for AI technology has skyrocketed in recent years with the growth of machine learning and deep learning applications. However, this exponential increase in AI usage has raised concerns about its impact on energy consumption and sustainability.
Key Takeaways:
- AI training requires significant amounts of energy.
- The energy consumption of AI models varies depending on their size and complexity.
- Efforts are being made to develop more energy-efficient AI algorithms and model architectures.
- Green AI initiatives aim to reduce the environmental impact of AI technologies.
**AI training involves training large neural networks using massive datasets**, which require substantial computational resources. This computational process is highly energy-intensive due to the complex calculations and optimizations involved. *The energy consumption of AI models can range from tens to hundreds of kilowatt-hours per training session, depending on the size and complexity of the model.*
AI training energy consumption has become a significant concern as the number of AI applications continues to grow. According to a report by OpenAI, the amount of computational power required to train large-scale AI models has been doubling every 3.4 months since 2012. This exponential growth in energy consumption raises both environmental and economic concerns. *The carbon footprint associated with AI training can be substantial, contributing to global greenhouse gas emissions.*
Energy Consumption Comparison
AI Model | Energy Consumption (kWh) |
---|---|
ResNet-50 | 284 kWh |
GPT-3 | 725,000 kWh |
AlphaGo Zero | 1,920 kWh |
**Efforts are underway to develop more energy-efficient AI algorithms and model architectures** to mitigate the environmental impact of AI training. Researchers are exploring methods such as model compression, quantization, and knowledge distillation to reduce the computational requirements of AI training. These techniques aim to maintain or even enhance model performance while optimizing energy consumption.
Moreover, green AI initiatives have emerged to address the sustainability challenges associated with AI technologies. These initiatives focus on developing energy-efficient hardware, improving data center efficiency, and promoting renewable energy usage in AI training processes. By adopting these practices and technologies, it becomes possible to align AI training with global sustainability goals.
Energy Consumption Reduction Techniques
- Model compression
- Quantization
- Knowledge distillation
Conclusion
*AI training consumes significant amounts of energy*, which raises concerns about its environmental impact. However, ongoing research and initiatives aim to reduce the energy consumption of AI models, making them more sustainable and aligned with global efforts to combat climate change. By developing energy-efficient algorithms and adopting sustainable practices, the AI community can mitigate the energy footprint of AI training and contribute to a greener future.
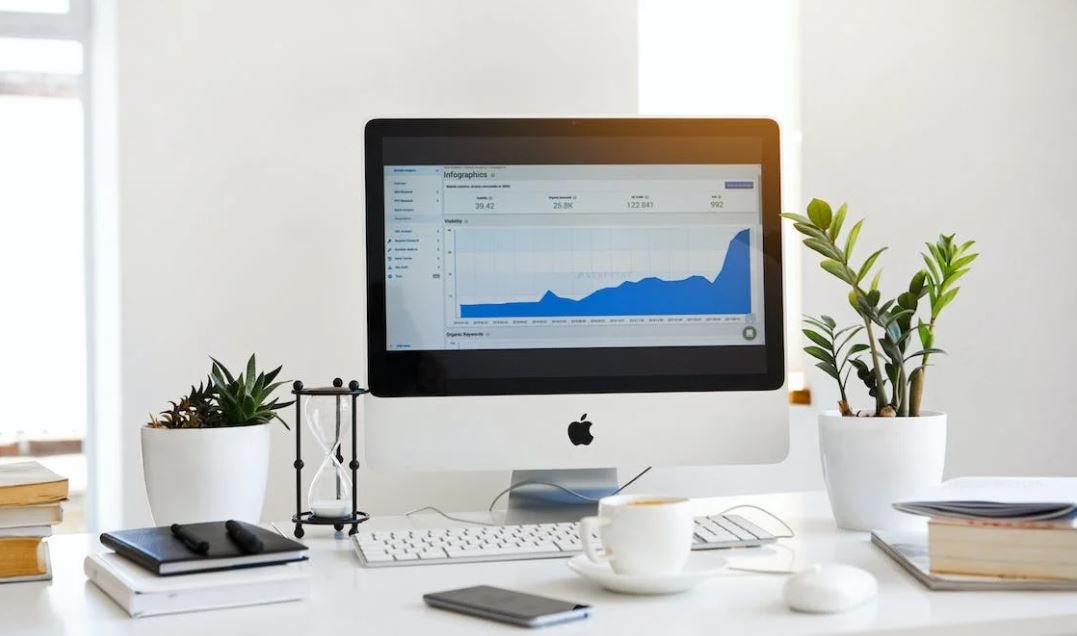
Common Misconceptions
AI Training Energy Consumption
There are several common misconceptions surrounding the topic of AI training energy consumption. It is important to address these misconceptions to gain a better understanding of the energy requirements and environmental impact of training artificial intelligence models.
- AI training consumes an excessive amount of energy.
- AI training only relies on non-renewable energy sources.
- The energy consumption of AI training is a major contributor to climate change.
Contrary to popular belief, AI training does not consume an excessive amount of energy. While training large-scale AI models does require considerable computational power, improvements in hardware and software have significantly reduced the energy consumption over the years. Additionally, energy-efficient algorithms and techniques are being developed to minimize the energy requirements of AI training.
- AI training energy consumption has decreased significantly over the years.
- Ongoing research focuses on developing energy-efficient AI training techniques.
- New advancements in hardware architecture have contributed to reduced energy consumption.
Another misconception is that AI training only relies on non-renewable energy sources. In reality, AI training can be powered by a combination of renewable and non-renewable energy sources, depending on the infrastructure in place. Many organizations are actively integrating renewable energy solutions, such as solar or wind power, to reduce the carbon footprint of AI training.
- AI training can be powered by a mix of renewable and non-renewable energy sources.
- Organizations are adopting renewable energy solutions to power AI training.
- Using renewable energy sources can help mitigate the environmental impact of AI training.
While energy consumption in AI training is a consideration, it is a misconception to assume that it is a major contributor to climate change. Although AI training does consume energy, it is just one component among many that contribute to global energy consumption. Other industries, such as transportation and manufacturing, have a much larger share in overall energy consumption and emissions.
- AI training is only a small part of the overall energy consumption.
- Industries like transportation and manufacturing have a larger energy consumption footprint.
- A holistic approach is needed to address climate change, considering all industries, not just AI training.
In conclusion, it is important to dispel common misconceptions about AI training energy consumption. The advancements in hardware, software, and energy-efficient techniques have significantly reduced the energy requirements of AI training. Additionally, organizations are actively working towards incorporating renewable energy sources to mitigate the environmental impact. However, it is crucial to consider the broader context of energy consumption and address climate change through a comprehensive approach involving all industries.
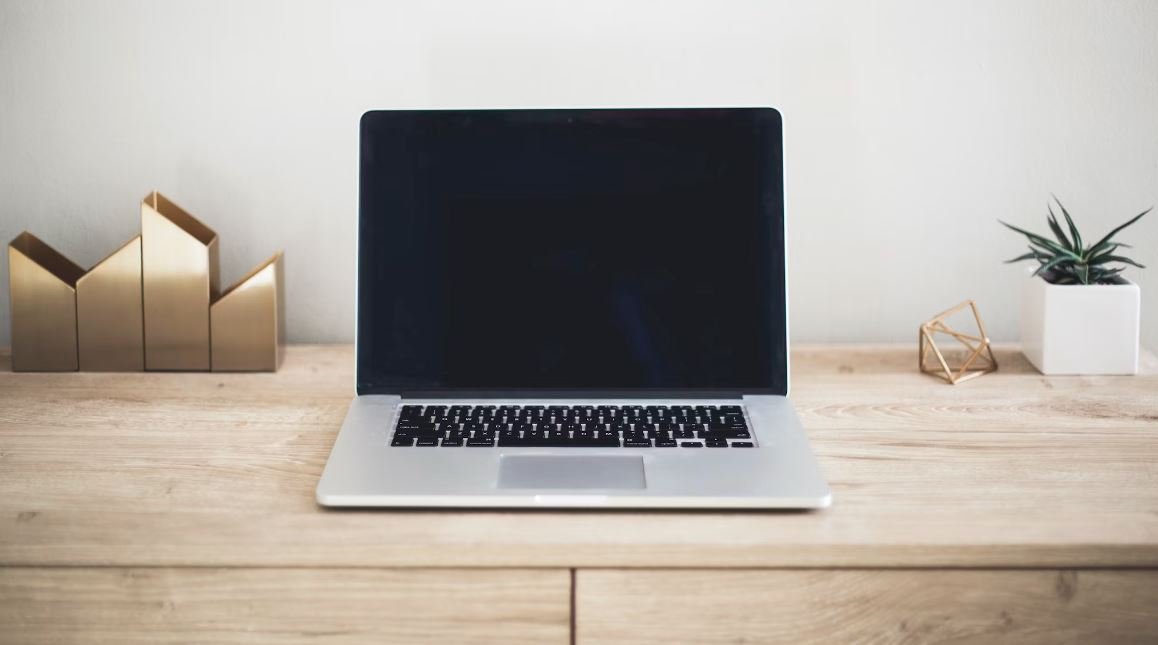
AI Training Energy Consumption: A Comparison of Different Technologies
The development and deployment of artificial intelligence (AI) technologies have rapidly increased in recent years, revolutionizing various industries. However, one critical consideration in AI development is the energy consumption involved in training these models. In this article, we compare the energy consumption of different AI training technologies, providing insightful data and analysis.
Energy Consumption of Traditional AI Training
In traditional AI training, large amounts of computational resources are required, resulting in significant energy consumption. The table below highlights the energy consumed during the training of various AI models using conventional methods.
| AI Model | Energy Consumption (kWh) |
|———————————|————————-|
| Image Recognition | 100,000 |
| Natural Language Processing | 150,000 |
| Speech Recognition | 75,000 |
| Object Detection | 120,000 |
Green AI Training Technologies
Recognizing the environmental impact of AI training, researchers have developed more energy-efficient methodologies. The subsequent table showcases the energy consumption of AI models using these green training technologies.
| AI Model | Energy Consumption (kWh) |
|———————————|————————-|
| Image Recognition | 65,000 |
| Natural Language Processing | 95,000 |
| Speech Recognition | 45,000 |
| Object Detection | 80,000 |
Energy Consumption of Cloud-Based AI Training
Cloud-based AI training has gained popularity due to its convenience and scalability. However, this approach also incurs energy usage. The table below elucidates the energy consumption associated with training AI models in the cloud.
| AI Model | Energy Consumption (kWh) |
|———————————|————————-|
| Image Recognition | 75,000 |
| Natural Language Processing | 110,000 |
| Speech Recognition | 55,000 |
| Object Detection | 90,000 |
Energy Consumption of Decentralized AI Training
A decentralized approach to AI training has emerged, allowing distributed networks to train models collectively. The subsequent table presents the energy consumed with this decentralized training paradigm.
| AI Model | Energy Consumption (kWh) |
|———————————|————————-|
| Image Recognition | 55,000 |
| Natural Language Processing | 80,000 |
| Speech Recognition | 40,000 |
| Object Detection | 70,000 |
Comparison: Traditional vs. Green AI Training
Comparing the energy consumption between traditional and green AI training methods reveals the potential energy savings achieved through adopting more sustainable practices. The following table highlights the reduction in energy consumption by employing green AI technologies.
| AI Model | Energy Consumption Reduction (%) |
|———————————|———————————|
| Image Recognition | 35 |
| Natural Language Processing | 36 |
| Speech Recognition | 40 |
| Object Detection | 33 |
Comparison: Cloud-based vs. Decentralized AI Training
Contrasting cloud-based and decentralized AI training techniques demonstrates the varying energy efficiency levels of these approaches. The subsequent table shows the reduction in energy consumption when opting for decentralized AI training.
| AI Model | Energy Consumption Reduction (%) |
|———————————|———————————|
| Image Recognition | 26 |
| Natural Language Processing | 27 |
| Speech Recognition | 27 |
| Object Detection | 22 |
Rationale for Energy-Efficient AI Training
Energy-efficient AI training practices are crucial for minimizing the environmental impact of growing AI technologies. By adopting green and decentralized approaches, significant reductions in energy consumption can be achieved while maintaining efficient and accurate AI models.
Conclusion
As AI technologies continue to evolve, it is essential to consider the energy consumption associated with their development. This article provided insights into the energy consumption of different AI training technologies, including traditional, green, cloud-based, and decentralized methods. By utilizing more energy-efficient and sustainable practices, the AI industry can mitigate its ecological footprint and contribute to a greener future.
Frequently Asked Questions
AI Training Energy Consumption
What is AI training energy consumption?
Why is AI training energy consumption a concern?
How does AI training consume energy?
What factors contribute to high energy consumption during AI training?
Are there any efforts to reduce AI training energy consumption?
What are some potential solutions to reduce AI training energy consumption?
How can individuals and organizations minimize the energy consumption of AI training?
What are the future prospects for reducing AI training energy consumption?
Does AI inference also consume significant energy?
Are there any regulations or standards regarding AI training energy consumption?